Time Series Data May Exhibit Which Of The Following Behaviors
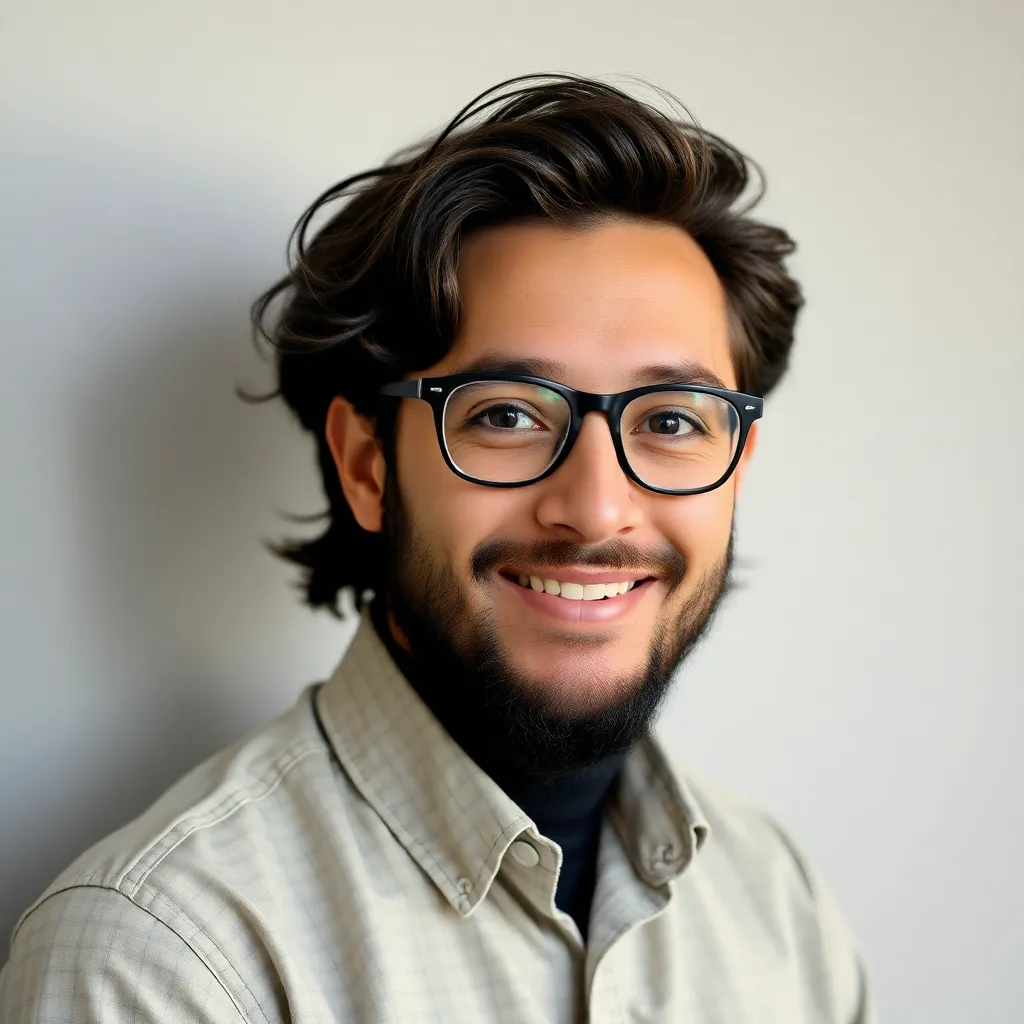
Onlines
Apr 08, 2025 · 7 min read

Table of Contents
Time Series Data: Unveiling the Dance of Trends, Seasonality, and Cycles
Time series data, a sequence of data points indexed in time order, is ubiquitous across diverse fields. From financial markets and weather forecasting to website analytics and healthcare monitoring, understanding its inherent behaviors is crucial for effective analysis and prediction. This article delves deep into the common characteristics of time series data, exploring the intricacies of trends, seasonality, cycles, and other significant patterns. We'll unpack each behavior, illustrating them with practical examples and discussing their implications for analysis and forecasting.
Key Behaviors of Time Series Data
Time series data often exhibits predictable patterns and unpredictable fluctuations. Understanding these behaviors is paramount for building accurate models and extracting meaningful insights. The key behaviors include:
1. Trend: The Long-Term Direction
A trend represents the long-term direction or movement of the data. It's the general tendency of the series to increase, decrease, or remain relatively constant over time. Trends can be:
- Upward Trend: The data consistently increases over time. Example: The growth of global internet users over the past two decades.
- Downward Trend: The data consistently decreases over time. Example: The decline in the number of landline phone subscriptions.
- No Trend (Stationary): The data fluctuates around a constant mean, exhibiting no consistent increase or decrease. Example: Daily temperature fluctuations in a stable climate.
Identifying Trends: Visual inspection using line graphs is often the first step. More sophisticated techniques include moving averages, regression analysis, and decomposition methods. A strong upward or downward trend often requires transformations (e.g., logarithmic transformation) to stabilize the variance before applying certain forecasting models.
Impact on Forecasting: Understanding the trend is critical for accurate forecasting. Models that ignore a strong trend will produce inaccurate predictions. Forecasting techniques like exponential smoothing and ARIMA models explicitly account for trends.
2. Seasonality: Recurring Patterns within Fixed Time Periods
Seasonality refers to recurring patterns within fixed time periods, such as daily, weekly, monthly, or yearly cycles. These fluctuations repeat consistently over time. Examples include:
- Daily Seasonality: Higher website traffic during weekdays compared to weekends; peak electricity consumption during evening hours.
- Weekly Seasonality: Increased sales of certain products on Fridays or Saturdays; higher hospital admissions on Mondays.
- Monthly Seasonality: Higher sales of winter coats in December; increased vacation bookings during summer months.
- Yearly Seasonality: Higher ice cream sales in summer; increased demand for heating oil in winter.
Identifying Seasonality: Visual inspection of time series plots is helpful, revealing cyclical patterns. Techniques like autocorrelation functions (ACF) and partial autocorrelation functions (PACF) can quantitatively identify the presence and periodicity of seasonality. Seasonal decomposition methods decompose the time series into its trend, seasonal, and residual components.
Impact on Forecasting: Seasonal patterns must be explicitly incorporated into forecasting models. Models like SARIMA (Seasonal Autoregressive Integrated Moving Average) and Prophet are designed to handle seasonality effectively. Ignoring seasonality leads to biased and inaccurate forecasts, especially when predicting future values within specific seasons.
3. Cyclicity: Recurring Patterns with Variable Period Lengths
Cyclicity involves recurring fluctuations, but unlike seasonality, the period length isn't fixed. These cycles can be irregular and difficult to predict. Examples include:
- Business Cycles: Periods of economic expansion and contraction.
- Sunspot Cycles: Fluctuations in solar activity.
- Population Cycles: Variations in animal populations.
Identifying Cyclicity: Identifying cycles is more challenging than identifying trends and seasonality due to their irregular nature. Spectral analysis and wavelet transforms can detect cyclical components, but interpretation requires careful consideration of the data's context and potential confounding factors.
Impact on Forecasting: Incorporating cyclicality into forecasting models is complex and requires more advanced techniques. Often, a combination of statistical and qualitative methods is used. Understanding the potential drivers of these cycles (e.g., economic policies, environmental factors) is crucial for informed prediction.
4. Irregularity (Noise): Random Fluctuations
Irregularity, also known as noise or randomness, represents unpredictable fluctuations in the data that cannot be attributed to trend, seasonality, or cycles. These fluctuations are often caused by random events or measurement errors.
Identifying Irregularity: Residuals from trend and seasonal decomposition models represent the irregular component. Analyzing the distribution and autocorrelation of the residuals can help assess the randomness of the fluctuations. High levels of irregularity can hinder accurate forecasting.
Impact on Forecasting: While randomness can't be predicted directly, understanding its level and characteristics helps evaluate the accuracy and uncertainty of forecasts. Robust forecasting techniques are needed to minimize the impact of noise on predictions.
5. Level Shifts: Abrupt Changes in the Mean
Level shifts represent sudden and permanent changes in the mean of the time series. These shifts can be caused by significant events or structural changes. Examples include:
- Policy Changes: Implementation of a new tax policy leading to a sudden shift in economic indicators.
- Technological Advancements: Introduction of a new technology leading to a disruption in market trends.
- Natural Disasters: A major earthquake leading to a significant decrease in economic activity.
Identifying Level Shifts: Visual inspection is often the first step, looking for abrupt changes in the average level of the data. Statistical tests like Chow tests can be employed to formally detect level shifts.
Impact on Forecasting: Level shifts require special attention in forecasting. Models need to be able to adapt to these structural changes to remain accurate. Methods incorporating intervention variables or structural breaks can accommodate level shifts effectively.
6. Outliers: Extreme Values Deviating Significantly from the Pattern
Outliers are extreme values that deviate significantly from the overall pattern of the data. They can be caused by measurement errors, unusual events, or simply random fluctuations.
Identifying Outliers: Visual inspection of time series plots is a good starting point. Statistical methods, such as the boxplot rule or robust outlier detection techniques, can be used to identify outliers more objectively.
Impact on Forecasting: Outliers can significantly influence the estimation of forecasting models. Their impact should be carefully evaluated and addressed. Robust regression techniques or methods that downweight outliers can produce more reliable forecasts.
7. Autocorrelation: Dependence Between Data Points
Autocorrelation refers to the correlation between data points at different time lags. Positive autocorrelation indicates that consecutive data points tend to be similar, while negative autocorrelation indicates that consecutive data points tend to be dissimilar.
Identifying Autocorrelation: Autocorrelation functions (ACF) and partial autocorrelation functions (PACF) are used to measure and visualize autocorrelation.
Impact on Forecasting: Autocorrelation is a fundamental characteristic used in many time series models, such as ARIMA models. Understanding the autocorrelation structure of the data is crucial for selecting and fitting appropriate forecasting models.
Analyzing and Forecasting Time Series Data: A Holistic Approach
Analyzing and forecasting time series data is a multifaceted process that requires careful consideration of the data's characteristics and the selection of appropriate modeling techniques. Here’s a structured approach:
-
Data Exploration and Preprocessing: Begin by visually inspecting the data through plots (line graphs, histograms, scatter plots) to identify patterns like trends, seasonality, and cycles. Check for missing values, outliers, and data quality issues, addressing these through imputation or outlier treatment.
-
Stationarity Assessment: Many time series models assume stationarity (constant mean and variance over time). If the data is non-stationary, transformations (e.g., differencing, logging) might be necessary to achieve stationarity before modeling.
-
Model Selection: Choose an appropriate forecasting model based on the identified patterns. Consider models like:
- Simple Moving Average: Suitable for data with no strong trends or seasonality.
- Exponential Smoothing: Handles trends and seasonality effectively.
- ARIMA (Autoregressive Integrated Moving Average): A versatile model that captures autocorrelation and trends.
- SARIMA (Seasonal ARIMA): Extends ARIMA to handle seasonality.
- Prophet (Facebook's Forecasting Model): Designed for business time series data, handles trends, seasonality, and holidays.
-
Model Fitting and Evaluation: Fit the selected model to the data and evaluate its performance using appropriate metrics like Mean Absolute Error (MAE), Root Mean Squared Error (RMSE), and Mean Absolute Percentage Error (MAPE). Cross-validation techniques ensure robust model evaluation.
-
Forecasting and Interpretation: Use the fitted model to generate forecasts for future time periods. Interpret the forecasts in the context of the data and the identified patterns. Consider the uncertainty associated with the forecasts and communicate this uncertainty effectively.
-
Monitoring and Updating: Time series data often evolves over time. Continuously monitor the model's performance and update it as new data becomes available to ensure its accuracy and relevance.
Conclusion: Mastering the Rhythms of Time Series Data
Time series data, with its rich tapestry of trends, seasonality, cycles, and randomness, presents both challenges and opportunities. By carefully analyzing its behaviors, selecting appropriate models, and interpreting forecasts in context, we can unlock valuable insights and make informed predictions across a spectrum of applications. Mastering the nuances of time series analysis empowers data-driven decision-making and a deeper understanding of the dynamic world around us. Remember that the journey towards effective time series forecasting is an iterative process – continuous monitoring, model refinement, and adaptation are key to maintaining accuracy and relevance in the ever-changing landscape of data.
Latest Posts
Latest Posts
-
Drag The Labels To Identify The Structures On A Vertebra
Apr 16, 2025
-
Cody Has Been Dealing With Chronic Stress
Apr 16, 2025
-
Amoeba Sisters Video Recap Monohybrid Crosses
Apr 16, 2025
-
Luis Fernando Compra Tres En La Libreria
Apr 16, 2025
-
How Might The Following Synthesis Be Carried Out
Apr 16, 2025
Related Post
Thank you for visiting our website which covers about Time Series Data May Exhibit Which Of The Following Behaviors . We hope the information provided has been useful to you. Feel free to contact us if you have any questions or need further assistance. See you next time and don't miss to bookmark.