Ultimately What Does A Forecast Scenario Consist Of
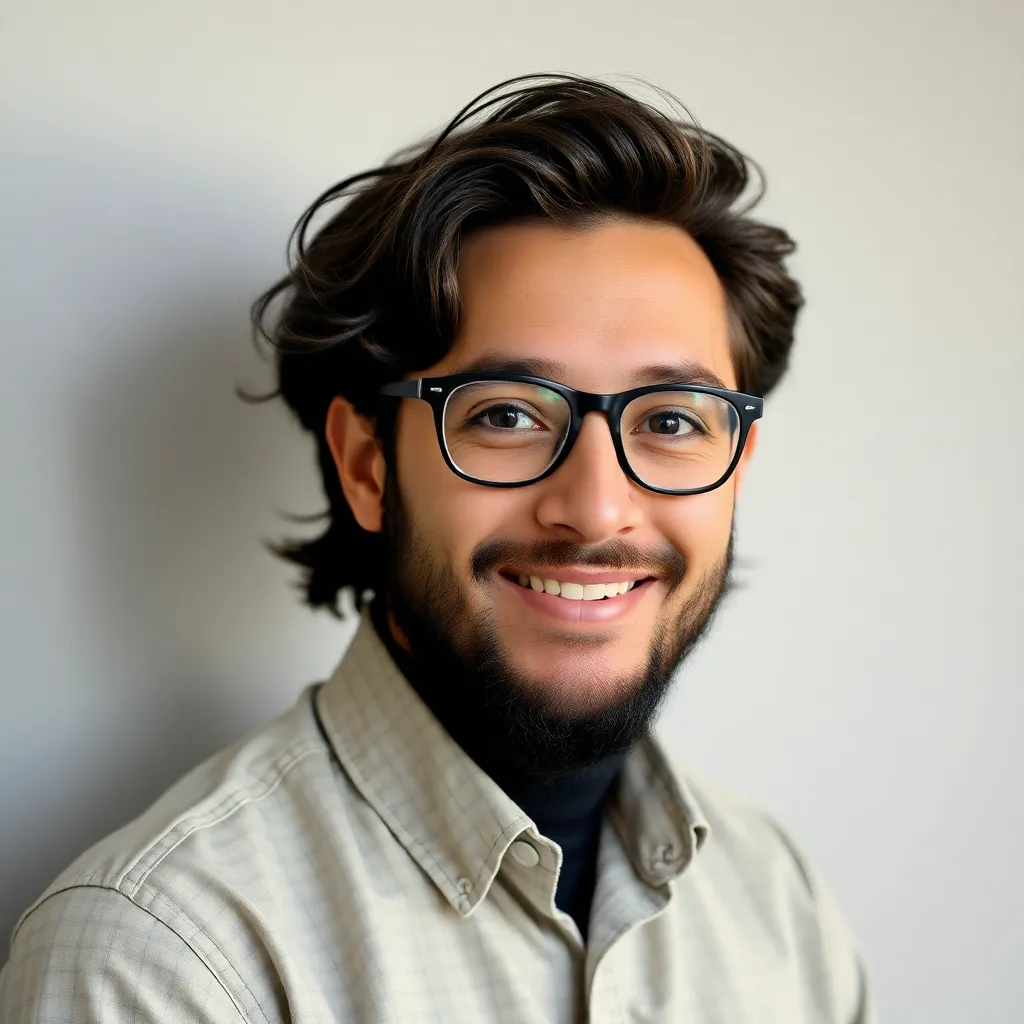
Onlines
May 05, 2025 · 6 min read

Table of Contents
Ultimately, What Does a Forecast Scenario Consist Of?
Forecasting, in its broadest sense, is the process of making predictions about the future based on past and present data. While seemingly simple, the creation of a robust and useful forecast scenario involves a complex interplay of data, assumptions, and modeling techniques. This article delves deep into the fundamental components of a comprehensive forecast scenario, exploring the various elements required for accuracy, reliability, and actionable insights.
The Foundation: Data Collection and Preparation
The bedrock of any successful forecast scenario is high-quality data. Without accurate and relevant data, even the most sophisticated models will produce unreliable predictions. This stage encompasses several crucial steps:
1. Identifying Relevant Data Sources:
This initial phase requires a clear understanding of the forecasting objective. What are you trying to predict? Sales figures? Market share? Economic growth? The answer dictates the types of data needed. Sources might include:
- Internal Data: Sales records, production data, customer databases, financial statements, inventory levels.
- External Data: Market research reports, economic indicators (GDP, inflation, unemployment), competitor analysis, industry trends, government statistics, social media sentiment.
- Proprietary Data: Unique data sets gathered through surveys, experiments, or specialized tracking systems.
2. Data Cleaning and Preprocessing:
Raw data is rarely perfect. This stage involves handling missing values, identifying and correcting errors (outliers), transforming data into a usable format (e.g., converting categorical variables into numerical ones), and dealing with inconsistencies. Techniques include:
- Imputation: Filling in missing values using statistical methods like mean/median imputation or more sophisticated techniques like k-nearest neighbors.
- Outlier Detection and Treatment: Identifying and either removing or transforming outliers to prevent them from disproportionately influencing the forecast.
- Data Transformation: Applying mathematical transformations (e.g., logarithmic, square root) to normalize data and improve model performance.
3. Data Validation and Verification:
Before proceeding, it's crucial to verify the accuracy and reliability of the cleaned data. This can involve:
- Cross-referencing: Comparing data from multiple sources to identify inconsistencies.
- Data consistency checks: Ensuring that the data adheres to defined constraints and formats.
- Expert review: Consulting subject matter experts to assess the data's validity and relevance.
Building the Scenario: Assumptions and Models
Once the data is prepared, the next crucial step involves defining the assumptions and selecting appropriate forecasting models.
1. Defining Key Assumptions:
Forecast scenarios are inherently based on assumptions about future conditions. These assumptions can significantly impact the results, so it's vital to document them clearly. Examples include:
- Economic Assumptions: Projected GDP growth, inflation rates, interest rates.
- Market Assumptions: Expected market size, competitive landscape, consumer behavior changes.
- Technological Assumptions: Development of new technologies, adoption rates, impact on the industry.
- Political Assumptions: Government regulations, trade policies, geopolitical events.
Clearly articulating these assumptions allows for sensitivity analysis (testing the impact of varying assumptions on the forecast), enhancing the scenario's robustness and providing insights into the uncertainty inherent in forecasting.
2. Selecting Appropriate Forecasting Models:
The choice of forecasting model depends on several factors, including the nature of the data, the forecasting horizon, and the desired level of accuracy. Popular models include:
- Time Series Models: Analyze historical data patterns to predict future values (e.g., ARIMA, Exponential Smoothing). Suitable for forecasting data with clear temporal dependencies.
- Regression Models: Establish relationships between dependent and independent variables to predict future values (e.g., linear regression, multiple regression). Useful when other factors influence the variable being forecasted.
- Causal Models: Explore cause-and-effect relationships between variables to understand how changes in one variable impact another. Useful for understanding the drivers of change.
- Machine Learning Models: Advanced algorithms that can identify complex patterns and relationships in data (e.g., neural networks, support vector machines). Often require large datasets and significant computational power.
The selection process should involve considering the model's accuracy, interpretability, and computational requirements.
Refining the Scenario: Sensitivity Analysis and Validation
Once a model is selected and the forecast generated, it's essential to refine the scenario through thorough testing and validation.
1. Sensitivity Analysis:
This crucial step involves testing the model's robustness by systematically varying the key assumptions. By observing how changes in assumptions affect the forecast, you can identify the most influential factors and assess the uncertainty associated with the predictions. This analysis should be documented clearly, highlighting the range of possible outcomes under different scenarios.
2. Backtesting and Validation:
Backtesting involves applying the chosen model to historical data to assess its performance in predicting past events. This helps to evaluate the model's accuracy and identify potential biases. Comparing the model's predictions to actual results provides valuable insights into its strengths and weaknesses. This also helps in selecting the most appropriate metrics to evaluate the model's performance (e.g., Mean Absolute Error (MAE), Root Mean Squared Error (RMSE), Mean Absolute Percentage Error (MAPE)).
3. Scenario Planning:
Creating multiple forecast scenarios, each based on different assumptions, is a vital step. This allows for a more comprehensive understanding of the range of possible future outcomes. Common scenarios include:
- Best-case scenario: Assumes favorable conditions and positive outcomes.
- Base-case scenario: Assumes a continuation of current trends.
- Worst-case scenario: Assumes unfavorable conditions and negative outcomes.
This approach provides a more nuanced perspective, mitigating the risk of relying on a single, potentially inaccurate forecast.
Communicating the Forecast Scenario: Visualization and Reporting
The final, and often overlooked, critical stage is effectively communicating the forecast scenario.
1. Visualizing the Results:
Visual representations of the forecast (charts, graphs, tables) are crucial for conveying complex information clearly and concisely. Different visualizations are suitable for different types of data and target audiences. Clear and well-labeled visualizations are essential for understanding the key findings.
2. Reporting and Documentation:
A well-written report should clearly articulate the forecasting objectives, methodology, assumptions, results, and limitations. It should also include a discussion of the uncertainties associated with the predictions and implications for decision-making. The report should be accessible to the target audience, regardless of their technical expertise. Detailed documentation of the data, models, and assumptions ensures transparency and reproducibility.
Conclusion: A Holistic Approach to Forecasting
Creating a robust and reliable forecast scenario is a multi-stage process requiring careful attention to detail and a holistic approach. From meticulous data collection and preparation to the selection of appropriate models and thorough validation, each step is essential for generating accurate and actionable predictions. By incorporating sensitivity analysis, scenario planning, and clear communication, forecast scenarios can provide valuable insights into the future, empowering informed decision-making and strategic planning. Remember, forecasting isn't about predicting the future with absolute certainty, but rather about understanding the range of possibilities and improving decision-making in the face of uncertainty. Continuous monitoring, evaluation, and refinement of the forecast scenario are crucial for maintaining its relevance and accuracy over time.
Latest Posts
Latest Posts
-
To Quickly Run A Nut Onto A Bolt
May 05, 2025
-
Mr Nguyen Understands That Medicare Prescription
May 05, 2025
-
Identify The Structure At The Tip Of The Arrow
May 05, 2025
-
Everyone Must Learn How To Work Their New Computer
May 05, 2025
-
A Can Of Soda Is Placed Inside A Cooler
May 05, 2025
Related Post
Thank you for visiting our website which covers about Ultimately What Does A Forecast Scenario Consist Of . We hope the information provided has been useful to you. Feel free to contact us if you have any questions or need further assistance. See you next time and don't miss to bookmark.