Using Logic To Compare Samples With Different Sources Of Variation
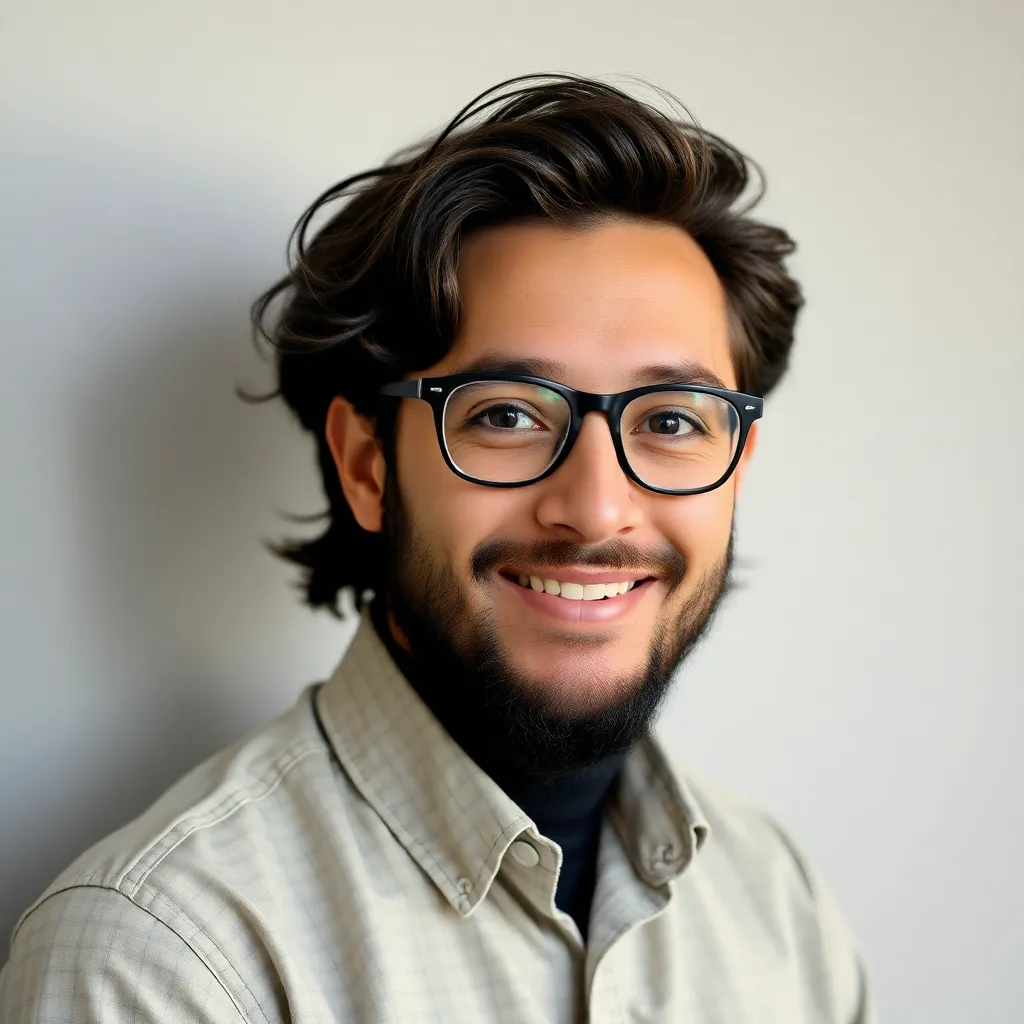
Onlines
Apr 06, 2025 · 6 min read

Table of Contents
Using Logic to Compare Samples with Different Sources of Variation
Comparing samples from different sources, each subject to unique variations, presents a significant challenge in data analysis. Simple comparisons often fail to account for these nuances, leading to inaccurate conclusions. This article explores the logical frameworks and statistical techniques needed to effectively compare such samples, ensuring robust and reliable insights. We'll delve into identifying sources of variation, employing appropriate statistical methods, and interpreting the results with a critical eye.
Understanding Sources of Variation
Before embarking on any comparison, a thorough understanding of the sources of variation affecting your samples is crucial. These variations can be broadly categorized into:
1. Treatment Variation:
This refers to the variation introduced by the factor you're investigating. For example, if you're comparing the effectiveness of two different fertilizers, the treatment variation represents the difference in plant growth attributable to the fertilizer itself.
2. Sampling Variation:
This reflects the natural variability within a population. Even if you're applying the same treatment, you'll observe differences in the samples due to inherent differences between individual units (plants, people, etc.). This is often the largest source of noise in data.
3. Environmental Variation:
External factors not directly controlled in the experiment can significantly influence your results. For instance, variations in temperature, sunlight, or soil composition can affect plant growth, irrespective of the fertilizer used. This is often difficult to account for completely.
4. Measurement Variation:
Variations arise from the process of collecting and measuring the data itself. Human error, instrument imprecision, and inconsistent measuring techniques all contribute to measurement variation.
Logical Frameworks for Comparison
Once you’ve identified the potential sources of variation, you need a logical framework to structure your comparison. This usually involves:
1. Defining a Null Hypothesis:
Begin by formulating a null hypothesis – a statement asserting no significant difference between the samples. For instance, "There is no significant difference in plant growth between the two fertilizers." Your analysis aims to either reject or fail to reject this null hypothesis.
2. Choosing Appropriate Statistical Tests:
The statistical test you choose depends heavily on the nature of your data and the sources of variation you're trying to account for. Several options exist:
-
t-tests: These are appropriate for comparing the means of two groups, assuming your data is normally distributed and variances are approximately equal. Variations of the t-test exist for independent samples (different groups) and paired samples (same group measured twice).
-
Analysis of Variance (ANOVA): This technique is used when comparing means across multiple groups (more than two). ANOVA can partition the total variation in the data into variations attributable to different sources (treatment, sampling, etc.).
-
Analysis of Covariance (ANCOVA): This extends ANOVA by incorporating continuous variables (covariates) that may affect the outcome variable. For instance, if you're comparing plant growth while accounting for initial plant height, ANCOVA is a better choice.
-
Regression Analysis: This allows you to explore the relationship between your outcome variable and multiple predictor variables, thereby disentangling the effects of various sources of variation.
-
Non-parametric Tests: If your data doesn't meet the assumptions of parametric tests (normality, equal variances), non-parametric alternatives exist (e.g., Mann-Whitney U test, Kruskal-Wallis test). These tests are less powerful but more robust.
3. Controlling for Confounding Variables:
Confounding variables are factors that influence both the independent and dependent variables, potentially obscuring the true relationship. Careful experimental design and statistical methods are crucial to control for these variables. Techniques include:
-
Randomization: Randomly assigning subjects to treatment groups helps to minimize the influence of unknown confounding variables.
-
Blocking: Grouping similar subjects together before random assignment can reduce variability within groups.
-
Matching: Selecting subjects that are similar in terms of relevant characteristics can also reduce confounding.
-
Statistical Control: Incorporating confounding variables as covariates in your analysis (e.g., ANCOVA, regression) can help isolate the effect of your treatment.
Interpreting Results and Drawing Conclusions
Interpreting the results of your statistical analysis requires careful consideration:
1. P-values and Significance Levels:
The p-value represents the probability of observing your results (or more extreme results) if the null hypothesis were true. A small p-value (typically less than 0.05) suggests that the observed differences are unlikely due to chance alone and provides evidence to reject the null hypothesis. However, it's crucial to remember that statistical significance doesn't automatically imply practical significance.
2. Effect Sizes:
While p-values indicate statistical significance, effect sizes quantify the magnitude of the difference between groups. Effect sizes provide a more meaningful interpretation of the practical implications of your findings. Common effect size measures include Cohen's d and eta-squared.
3. Confidence Intervals:
Confidence intervals provide a range of plausible values for the true difference between groups. A narrow confidence interval indicates a more precise estimate.
4. Visualizations:
Graphs and charts are essential for communicating your findings effectively. Box plots, scatter plots, and bar charts can clearly illustrate differences between groups and the extent of variability within each group.
Case Study: Comparing Crop Yields
Let's consider a hypothetical example: comparing the yields of three different wheat varieties (A, B, and C) grown in different fields under varying weather conditions.
Sources of Variation:
- Treatment Variation: Differences in wheat varieties (A, B, C).
- Environmental Variation: Variations in soil quality, rainfall, and sunlight across different fields.
- Sampling Variation: Inherent differences in wheat growth within each field.
- Measurement Variation: Errors in weighing and measuring the harvested wheat.
Logical Framework:
-
Null Hypothesis: There is no significant difference in the average yield among the three wheat varieties.
-
Statistical Test: ANOVA would be appropriate here to compare the means of the three groups, while accounting for the field as a blocking factor (to control for environmental variation).
-
Controlling for Confounding Variables: We could use ANCOVA to incorporate soil quality as a covariate if we have data on soil quality for each field.
Interpretation:
After conducting the ANOVA, we'd examine the p-value. A significant p-value would lead us to reject the null hypothesis, concluding that there is a significant difference in the average yield among the wheat varieties. We would then examine effect sizes and confidence intervals to understand the magnitude and precision of these differences. Visualizations (e.g., box plots) would help illustrate these findings clearly.
Advanced Considerations
-
Mixed-effects Models: These models are particularly useful when dealing with hierarchical or nested data structures (e.g., students nested within classrooms). They allow you to account for both fixed effects (treatment) and random effects (variations due to nesting).
-
Bayesian Methods: Bayesian approaches offer a flexible framework for incorporating prior knowledge into the analysis and quantifying uncertainty in a probabilistic manner.
-
Robust Statistical Methods: Robust methods are less sensitive to outliers and violations of assumptions, providing a more reliable analysis when dealing with noisy or non-normal data.
Conclusion
Comparing samples with different sources of variation requires a multifaceted approach combining logical reasoning, careful experimental design, appropriate statistical techniques, and critical interpretation of results. By meticulously identifying sources of variation, employing suitable statistical methods, and vigilantly controlling for confounding variables, you can draw robust and meaningful conclusions from your data, leading to more informed decision-making. The choice of statistical method depends heavily on the specific context, data characteristics, and research questions. Remember that statistical significance is just one piece of the puzzle; effect sizes, confidence intervals, and visualizations are equally important for a complete and insightful understanding of your findings.
Latest Posts
Latest Posts
-
Pn Learning System Comprehensive Final Quiz
Apr 07, 2025
-
Their Eyes Were Watching God Study Guide
Apr 07, 2025
-
I Sing The Body Electric Poem Analysis
Apr 07, 2025
-
The Scarlet Letter Chapter 21 Summary
Apr 07, 2025
-
Student Exploration Gmos And The Environment
Apr 07, 2025
Related Post
Thank you for visiting our website which covers about Using Logic To Compare Samples With Different Sources Of Variation . We hope the information provided has been useful to you. Feel free to contact us if you have any questions or need further assistance. See you next time and don't miss to bookmark.