What Does The Symbol R Indicate In An Experimental Design
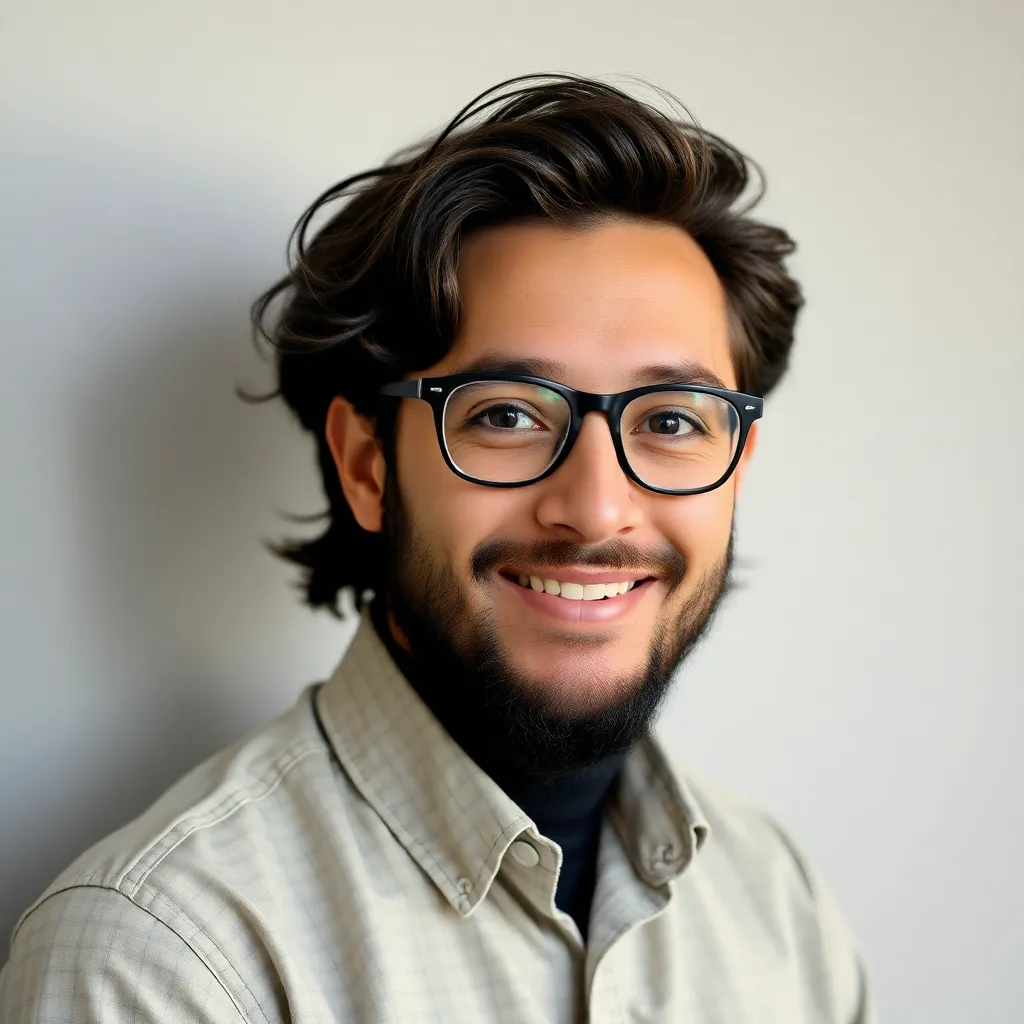
Onlines
May 08, 2025 · 5 min read
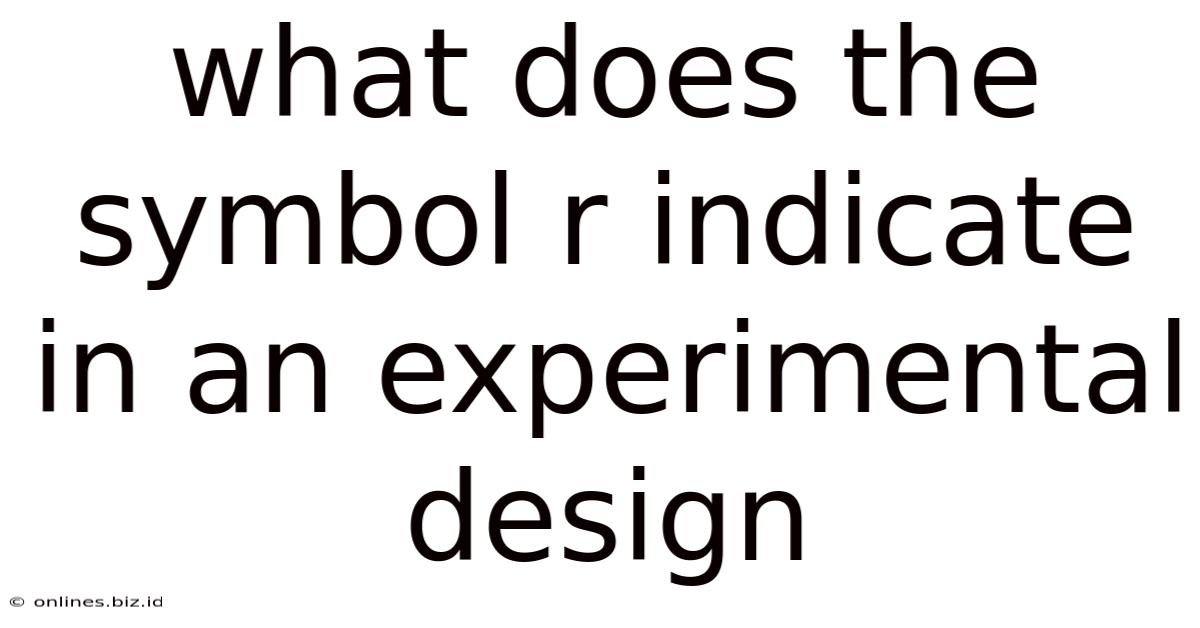
Table of Contents
What Does the Symbol 'r' Indicate in an Experimental Design?
The symbol 'r' in experimental design, specifically within the context of statistical analysis, typically represents the correlation coefficient. However, its precise meaning and interpretation are highly dependent on the specific experimental setup and the statistical test employed. This article will delve into the multifaceted role of 'r', exploring its various interpretations and applications in different experimental designs. We will examine its relationship to other statistical measures and highlight the crucial aspects of understanding and correctly interpreting this vital symbol.
Understanding the Correlation Coefficient (r)
At its core, 'r' quantifies the strength and direction of a linear relationship between two variables. It ranges from -1 to +1, where:
- r = +1: Indicates a perfect positive correlation. As one variable increases, the other increases proportionally.
- r = -1: Indicates a perfect negative correlation. As one variable increases, the other decreases proportionally.
- r = 0: Indicates no linear correlation between the variables. This doesn't necessarily mean there's no relationship, just that it's not linear. A non-linear relationship might exist.
The absolute value of 'r' signifies the strength of the relationship:
- |r| close to 1: Strong correlation
- |r| close to 0.5: Moderate correlation
- |r| close to 0: Weak correlation
Important Note: Correlation does not imply causation. Just because two variables are correlated doesn't mean one causes the other. A third, confounding variable could be influencing both.
r in Different Experimental Designs
The interpretation and use of 'r' varies across different experimental designs:
1. Correlation Studies
In correlation studies, 'r' is the primary focus. Researchers measure two or more variables without manipulating any of them and calculate 'r' to assess the relationship's strength and direction. For example, a study investigating the correlation between hours of sleep and academic performance would use 'r' to quantify the association. A high positive 'r' might suggest a link, but it doesn't prove that more sleep causes better grades.
2. Regression Analysis
Regression analysis uses 'r' (or its square, r²) to understand the predictive power of one or more predictor variables on an outcome variable. 'r²', the coefficient of determination, represents the proportion of variance in the outcome variable that's explained by the predictor variable(s). A higher r² suggests a better fit of the regression model. In simple linear regression (one predictor variable), r² is simply r².
For instance, in a study examining the impact of advertising spend on sales, regression analysis could use 'r' and 'r²' to determine the strength of the relationship and the percentage of sales variance explained by advertising spend.
3. Experimental Designs with Control Groups
Even in experiments with control groups, 'r' can play a role, particularly when analyzing relationships between covariates (variables not directly manipulated) and the outcome variable. For example, in a drug trial comparing a new drug to a placebo, 'r' might be used to explore the relationship between baseline health measures (e.g., blood pressure) and the treatment response. This could help identify subgroups that respond differently to the treatment. Analyzing correlations between covariates and treatment effects could lead to more nuanced and valuable findings.
4. Factorial Designs
In complex factorial designs involving multiple independent variables, 'r' can be calculated for various pairs of variables, including the independent variables themselves, the independent and dependent variables, or even interactions between independent variables and the dependent variable. This can give a comprehensive picture of how the factors relate to each other and the outcome. However, it’s vital to carefully interpret the correlation in such a context as there might be confounding effects.
Interpreting 'r' with Caution
Several crucial points must be emphasized when interpreting 'r':
-
Sample Size: The reliability of 'r' depends heavily on the sample size. Larger samples generally yield more stable and reliable estimates. A small sample size can lead to spurious correlations.
-
Outliers: Outliers (extreme data points) can disproportionately influence the value of 'r'. Carefully examine the data for outliers and consider their impact on the analysis. Robust correlation measures may be more suitable in the presence of outliers.
-
Non-linear Relationships: 'r' only measures linear relationships. A strong non-linear relationship might yield a low 'r' value or even 'r' = 0. Visualizing the data with scatter plots is crucial to detecting non-linear patterns.
-
Causation vs. Correlation: Reiterate: correlation does not imply causation. A high 'r' value suggests a relationship, but it does not prove a causal link. Further investigation and stronger experimental designs (e.g., randomized controlled trials) are needed to establish causality.
-
Context is Key: The interpretation of 'r' should always be considered within the context of the research question, experimental design, and other relevant factors. A moderate 'r' might be significant in one context but trivial in another.
Advanced Considerations
-
Partial Correlation: This technique assesses the correlation between two variables after controlling for the influence of one or more other variables. It's useful in situations where confounding variables might obscure the true relationship between the variables of interest.
-
Spearman's Rank Correlation (ρ): This non-parametric measure of correlation is suitable for ordinal data or data that doesn't meet the assumptions of Pearson's correlation (the typical 'r'). Spearman's ρ assesses the monotonic relationship (consistent increase or decrease) between variables.
-
Statistical Significance: 'r' alone doesn't indicate statistical significance. A hypothesis test (e.g., t-test) is needed to determine if the observed correlation is statistically significant, meaning it's unlikely to have occurred by chance. This test considers the sample size and the magnitude of 'r'.
Conclusion
The symbol 'r' in experimental design plays a crucial role in understanding the relationships between variables. While the correlation coefficient provides valuable insights into the strength and direction of linear relationships, its interpretation requires careful consideration of several factors, including sample size, outliers, the possibility of non-linear relationships, and the critical distinction between correlation and causation. Understanding these nuances is essential for drawing accurate and meaningful conclusions from experimental data. Always remember to combine statistical analyses like 'r' with strong experimental design and visual representations of the data for a thorough and robust understanding of the results. Context, rigorous analysis, and a critical eye are paramount in successfully interpreting 'r' and using it to derive meaningful conclusions from experimental data.
Latest Posts
Latest Posts
-
Board Of Education Of Minneapolis V Hughes
May 08, 2025
-
Emparejar Match Each Item With The Corresponding Phrase
May 08, 2025
-
A 29 Year Old Pregnant Woman Complains Of Severe Vomiting
May 08, 2025
-
Suppose That The Market For Sweaters Is Perfectly Competitive
May 08, 2025
-
Kerry Is Conditioned To Fear Strawberries
May 08, 2025
Related Post
Thank you for visiting our website which covers about What Does The Symbol R Indicate In An Experimental Design . We hope the information provided has been useful to you. Feel free to contact us if you have any questions or need further assistance. See you next time and don't miss to bookmark.