What Information About A Sample Does A Mean Not Provide
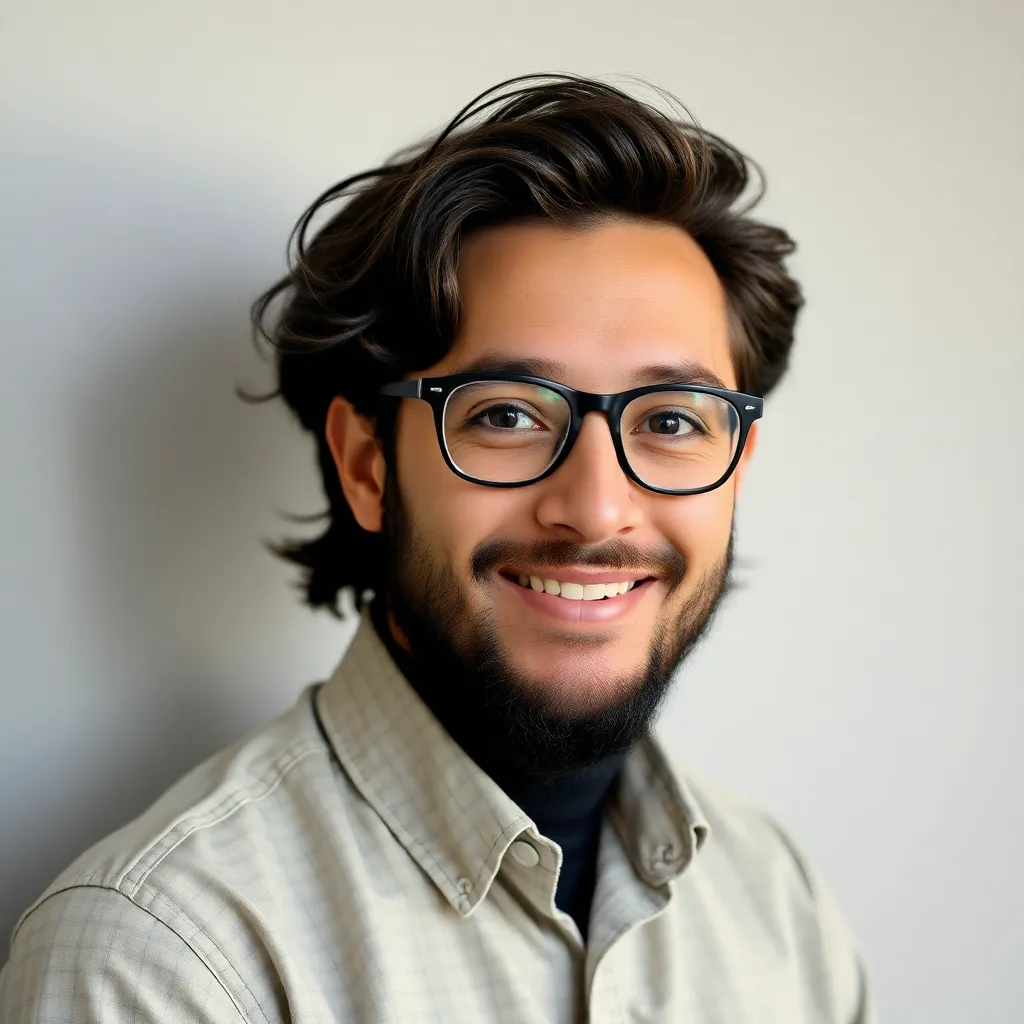
Onlines
May 08, 2025 · 5 min read
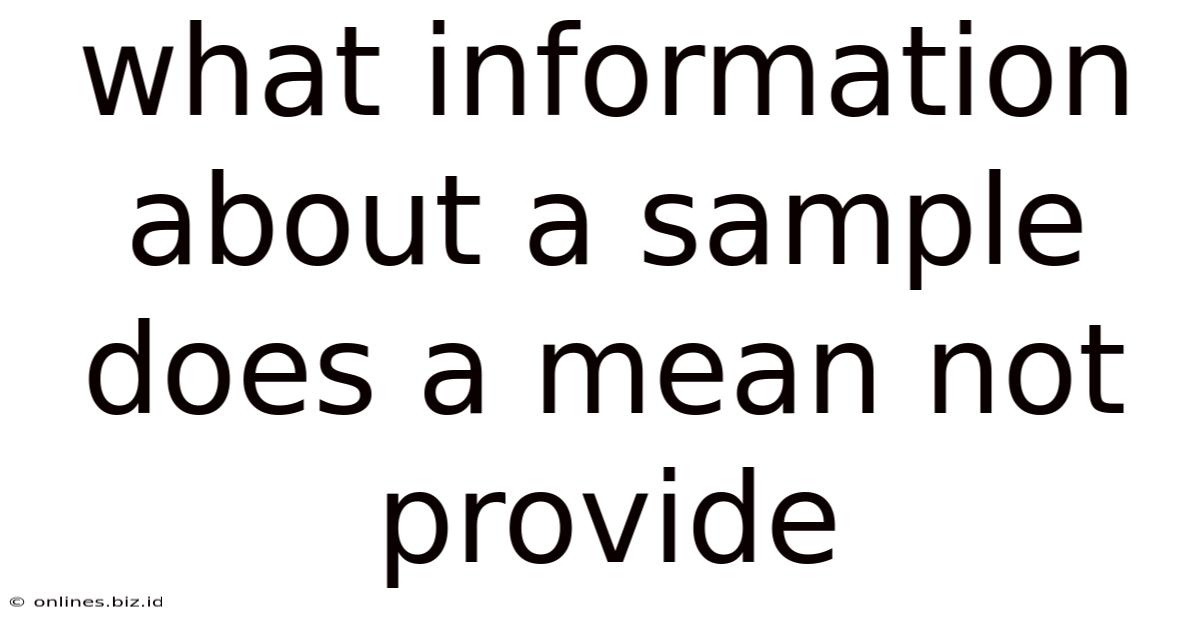
Table of Contents
What Information About a Sample Does a Mean Not Provide?
The mean, or average, is a fundamental descriptive statistic used across numerous fields. While incredibly useful for summarizing data, it's crucial to understand its limitations. A mean alone doesn't paint a complete picture of a dataset; it omits vital information about the distribution, variability, and the presence of outliers which are crucial for a thorough understanding. This article delves into the significant information a mean fails to convey, emphasizing the importance of using it in conjunction with other descriptive statistics for a comprehensive data analysis.
Beyond the Average: The Missing Pieces of the Puzzle
The mean, calculated by summing all values in a dataset and dividing by the number of values, provides a measure of central tendency. It indicates the central point around which the data is clustered. However, it's just one piece of the statistical puzzle. Here's what the mean doesn't tell you:
1. The Distribution of the Data: Shape and Skewness
The mean offers no insight into the shape of the data distribution. A dataset can have the same mean but vastly different distributions. Consider these scenarios:
-
Symmetrical Distribution: A perfectly symmetrical distribution (like a normal distribution) has the mean, median, and mode all at the same point. The mean accurately represents the center.
-
Skewed Distribution: In a skewed distribution, the mean is pulled towards the tail. A right-skewed distribution (positive skew) has a long tail on the right, and the mean will be greater than the median. A left-skewed distribution (negative skew) has a long tail on the left, and the mean will be less than the median. The mean, in this case, can be misleading as it's heavily influenced by the extreme values in the tail. It doesn't accurately reflect the typical value.
Example: Imagine two datasets representing the income of residents in two different towns:
- Town A: Mean income: $50,000. Distribution: Mostly clustered around $45,000 - $55,000 with a few very high earners. (Right-skewed)
- Town B: Mean income: $50,000. Distribution: Evenly spread between $40,000 and $60,000. (Symmetrical)
Both towns have the same mean income, yet the income distribution is drastically different. The mean alone masks this crucial difference.
2. The Variability and Spread of the Data: Standard Deviation and Range
The mean doesn't reveal the spread or dispersion of the data points around the mean. Two datasets can have the same mean but drastically different variability.
- High Variability: Data points are widely scattered around the mean.
- Low Variability: Data points are tightly clustered around the mean.
The standard deviation measures the average distance of each data point from the mean. A high standard deviation indicates high variability, while a low standard deviation indicates low variability. The range (the difference between the maximum and minimum values) also provides information about the spread. The mean provides no information about either.
Example: Consider two classes taking the same exam:
- Class A: Mean score: 75, Standard deviation: 10
- Class B: Mean score: 75, Standard deviation: 20
Both classes have the same average score, but Class B exhibits significantly more variability in student performance. The mean alone obscures this critical difference in the consistency of student achievement.
3. The Presence of Outliers: Extreme Values
Outliers, or extreme values, significantly impact the mean. A single outlier can drastically inflate or deflate the mean, misrepresenting the typical value. The mean is highly sensitive to outliers, unlike the median (the middle value).
Example: Consider a dataset of house prices: {150,000, 160,000, 170,000, 180,000, 2,000,000}. The mean is heavily influenced by the outlier (2,000,000), making it an inaccurate representation of the typical house price. The median would provide a much more representative value in this case.
4. The Shape of the Underlying Population Distribution
The sample mean is an estimate of the population mean. However, the mean alone doesn't provide information about the shape of the underlying population distribution. It is important to consider whether the population is normally distributed or follows another distribution. This understanding is critical for statistical inference and hypothesis testing.
5. The Relationship Between Variables: Correlation and Covariance
The mean cannot show the relationship between two or more variables. It only summarizes a single variable at a time. For instance, knowing the average income and average education level separately tells us nothing about the relationship between the two – whether higher education is linked to higher income. Correlation and covariance are the statistical measures used to assess relationships between variables.
6. The Contextual Information: Units and Meaningful Interpretation
While the mean provides a numerical value, it's crucial to consider its context. Without understanding the units of measurement and the nature of the data, the mean loses its meaning. For example, a mean of 10 could refer to 10 apples, 10 kilometers, or 10 years. The context is crucial for meaningful interpretation.
Using the Mean Effectively: Combining with Other Statistics
The mean is a valuable tool, but it shouldn't be used in isolation. To gain a comprehensive understanding of a dataset, it's essential to combine the mean with other descriptive statistics, such as:
- Median: The middle value when data is ordered. Less sensitive to outliers than the mean.
- Mode: The most frequent value. Useful for identifying the most common observation.
- Standard Deviation: Measures the spread of the data around the mean.
- Variance: The square of the standard deviation.
- Range: The difference between the maximum and minimum values.
- Interquartile Range (IQR): The range containing the middle 50% of the data. Less sensitive to outliers than the range.
- Skewness: Measures the asymmetry of the distribution.
- Kurtosis: Measures the "tailedness" of the distribution. Indicates the presence of outliers.
- Histograms and Box Plots: Visual representations of the data's distribution and spread. These are crucial for identifying patterns and outliers.
By using a combination of these descriptive statistics and visual representations, you can get a complete and nuanced understanding of your data, moving beyond the limited information provided by the mean alone. This comprehensive approach will lead to more accurate interpretations, stronger conclusions, and more reliable decision-making. Ignoring the limitations of the mean and neglecting these complementary statistics can lead to flawed interpretations and potentially erroneous conclusions. Remember, the mean is a valuable tool, but it's just one piece of the puzzle. A more thorough data analysis requires a more complete picture.
Latest Posts
Latest Posts
-
An Edible History Of Humanity Chapter Summary
May 08, 2025
-
An Echo Sonnet To An Empty Page Analysis
May 08, 2025
-
Main Characters In Catcher In The Rye
May 08, 2025
-
A Short Term Creditor Would Be Most Interested In
May 08, 2025
-
The Dolls House Katherine Mansfield Summary
May 08, 2025
Related Post
Thank you for visiting our website which covers about What Information About A Sample Does A Mean Not Provide . We hope the information provided has been useful to you. Feel free to contact us if you have any questions or need further assistance. See you next time and don't miss to bookmark.