Which Box And Whisker Plot Represents The Data Set
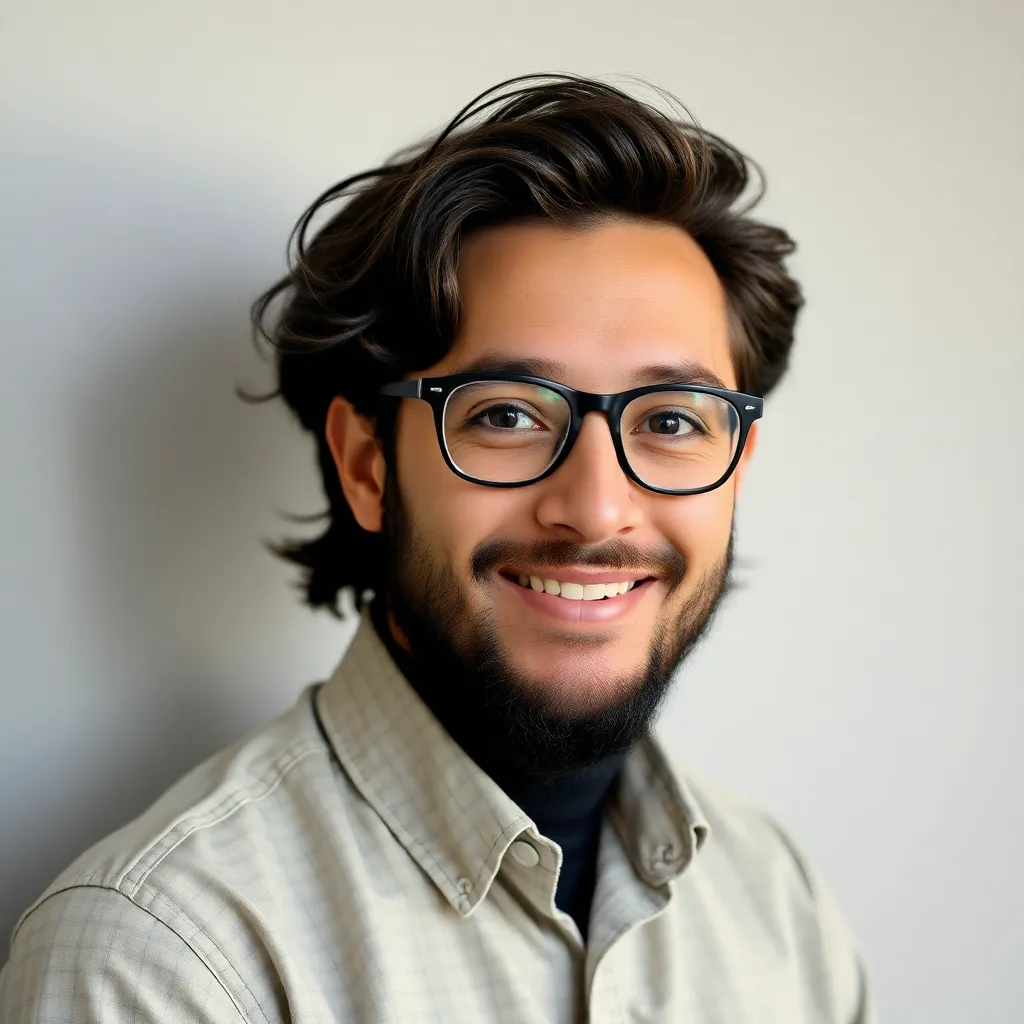
Onlines
Apr 17, 2025 · 6 min read

Table of Contents
Which Box and Whisker Plot Represents the Data Set? A Comprehensive Guide
Understanding how to interpret and create box and whisker plots is crucial for anyone working with data analysis. These plots provide a visual summary of the distribution of a dataset, highlighting key statistical measures like median, quartiles, and potential outliers. But how do you determine which box and whisker plot accurately represents a given data set? This comprehensive guide will walk you through the process, covering the fundamentals of box plots, interpretation techniques, and practical examples to solidify your understanding.
Understanding Box and Whisker Plots: The Fundamentals
A box and whisker plot (also known as a box plot) is a standardized way to display the distribution of a dataset. It visually represents five key summary statistics:
- Minimum: The smallest value in the dataset.
- First Quartile (Q1): The value that separates the bottom 25% of the data from the top 75%.
- Median (Q2): The middle value of the dataset when it's ordered. It separates the lower 50% from the upper 50%.
- Third Quartile (Q3): The value that separates the bottom 75% of the data from the top 25%.
- Maximum: The largest value in the dataset.
The box in the plot represents the interquartile range (IQR), which is the difference between Q3 and Q1 (IQR = Q3 - Q1). The whiskers extend from the box to the minimum and maximum values, providing a visual representation of the range of the data. Sometimes, outliers are identified and plotted as individual points beyond the whiskers.
Identifying Outliers: The 1.5 * IQR Rule
Outliers are data points that lie significantly outside the typical range of the data. A common method for identifying outliers is using the 1.5 * IQR rule:
- Lower Bound: Q1 - 1.5 * IQR
- Upper Bound: Q3 + 1.5 * IQR
Any data points falling below the lower bound or above the upper bound are considered outliers. These outliers are typically plotted as individual points beyond the whiskers. The whiskers themselves usually extend to the most extreme data points within the bounds (not including outliers).
Interpreting Box and Whisker Plots: Key Features
Once you have a box and whisker plot, several key features can be analyzed:
-
Skewness: A symmetrical distribution will have a box plot with roughly equal distances between the median and the quartiles. A skewed distribution will have a box plot where the median is closer to one end of the box than the other. A right-skewed distribution (positive skew) will have a longer whisker on the right, while a left-skewed distribution (negative skew) will have a longer whisker on the left. Understanding skewness is crucial for interpreting the overall distribution and identifying potential biases in the data.
-
Spread: The length of the box and whiskers represents the spread or variability of the data. A larger spread indicates greater variability, while a smaller spread suggests less variability.
-
Median: The median's position within the box provides insight into the symmetry of the data. A median located in the center of the box suggests symmetry, while a median closer to one end indicates skewness.
-
Outliers: The presence and number of outliers provide valuable information about potential errors in data collection or unusual data points that warrant further investigation. Outliers could indicate significant deviations from the typical pattern and may require additional analysis to determine if they are truly anomalous or valid data points.
Matching Data Sets to Box Plots: A Step-by-Step Approach
Let's consider a practical example. Suppose you have a data set and several box and whisker plots. How do you determine which plot correctly represents the data? Here's a step-by-step approach:
-
Calculate the Five-Number Summary: From your data set, calculate the minimum, Q1, median, Q3, and maximum.
-
Identify Outliers (if any): Calculate the IQR (Q3 - Q1) and use the 1.5 * IQR rule to identify any outliers.
-
Compare with the Box Plots: Examine each box and whisker plot and compare its five-number summary with your calculated values. Pay close attention to:
- The position of the box: Does the box's location and length match your calculated Q1, median, and Q3?
- The whiskers: Do the whiskers extend to the correct minimum and maximum values (or to the bounds, excluding outliers)?
- The outliers: Are the plotted outliers consistent with your identified outliers?
-
Analyze Skewness: Examine the shape of the box plot and compare it to the skewness of your calculated data.
-
Match the Plot: The box and whisker plot that accurately reflects all the above should be your match.
Practical Example:
Let's assume you have the following dataset: 2, 3, 4, 5, 5, 6, 7, 8, 9, 10, 100
-
Five-Number Summary:
- Minimum: 2
- Q1: 4
- Median: 6
- Q3: 9
- Maximum: 100
-
IQR: 9 - 4 = 5
-
Outliers:
- Lower Bound: 4 - 1.5 * 5 = -3.5
- Upper Bound: 9 + 1.5 * 5 = 16.5
- 100 is an outlier since it exceeds the upper bound.
Now, if you're given several box plots, you should look for the one that shows:
- A box stretching from approximately 4 to 9.
- A median line at 6.
- Whiskers extending to 2 and 16.5 (or close to those values).
- An outlier point plotted above 16.5, representing the value 100.
Any box plot that doesn't align with these features does not accurately represent the data set.
Advanced Applications and Considerations
Box plots are a versatile tool and can be applied in various scenarios, including:
-
Comparing distributions: Multiple box plots can be displayed side-by-side to easily compare the distributions of different datasets. This is useful for identifying differences in central tendency, spread, and skewness.
-
Identifying influential points: While outliers are extreme values, influential points are data points that significantly alter the results of a statistical analysis. Box plots can help identify potential influential points by indicating unusual values that warrant a closer look.
-
Detecting potential errors: Box plots are useful for visually detecting potential errors in data entry or collection. Outliers and unusual patterns can highlight potential data issues that might otherwise go unnoticed.
-
Data exploration: Before performing more complex statistical analyses, box plots can be used to explore the basic characteristics of the data and gain initial insights.
Remember that box plots are just one visualization tool, and it’s often beneficial to combine them with other methods, such as histograms or scatter plots, for a more comprehensive understanding of your data. The interpretation of box plots requires careful consideration of the context of the data and the questions being asked.
Conclusion: Mastering Box and Whisker Plots for Data Analysis
Understanding how to interpret and create box and whisker plots is an invaluable skill for anyone working with data. They provide a concise visual summary of key statistical measures, allowing for quick assessments of data distribution, skewness, and potential outliers. By systematically comparing calculated five-number summaries with the visual representation of the box plots, you can confidently determine which plot accurately depicts your data. Mastering this technique empowers you to make better data-driven decisions and communicate your findings effectively. By incorporating these techniques and considerations into your data analysis workflow, you can improve the accuracy, efficiency, and communication of your findings. Remember, data visualization is a powerful tool, and mastering techniques like box and whisker plot interpretation is key to unlocking its full potential.
Latest Posts
Latest Posts
-
9 3 3 Packet Tracer Hsrp Configuration Guide
Apr 19, 2025
-
The Guideline For Programming Hypertrophy Is
Apr 19, 2025
-
Lets Focus On Pathos Answer Key
Apr 19, 2025
-
Pirate Riddle 2 Dividing Fractions Answer Key
Apr 19, 2025
-
Screen Addiction Among Teens Is There Such A Thing Answers
Apr 19, 2025
Related Post
Thank you for visiting our website which covers about Which Box And Whisker Plot Represents The Data Set . We hope the information provided has been useful to you. Feel free to contact us if you have any questions or need further assistance. See you next time and don't miss to bookmark.