Which Experiment Would Most Likely Contain Experimental Bias
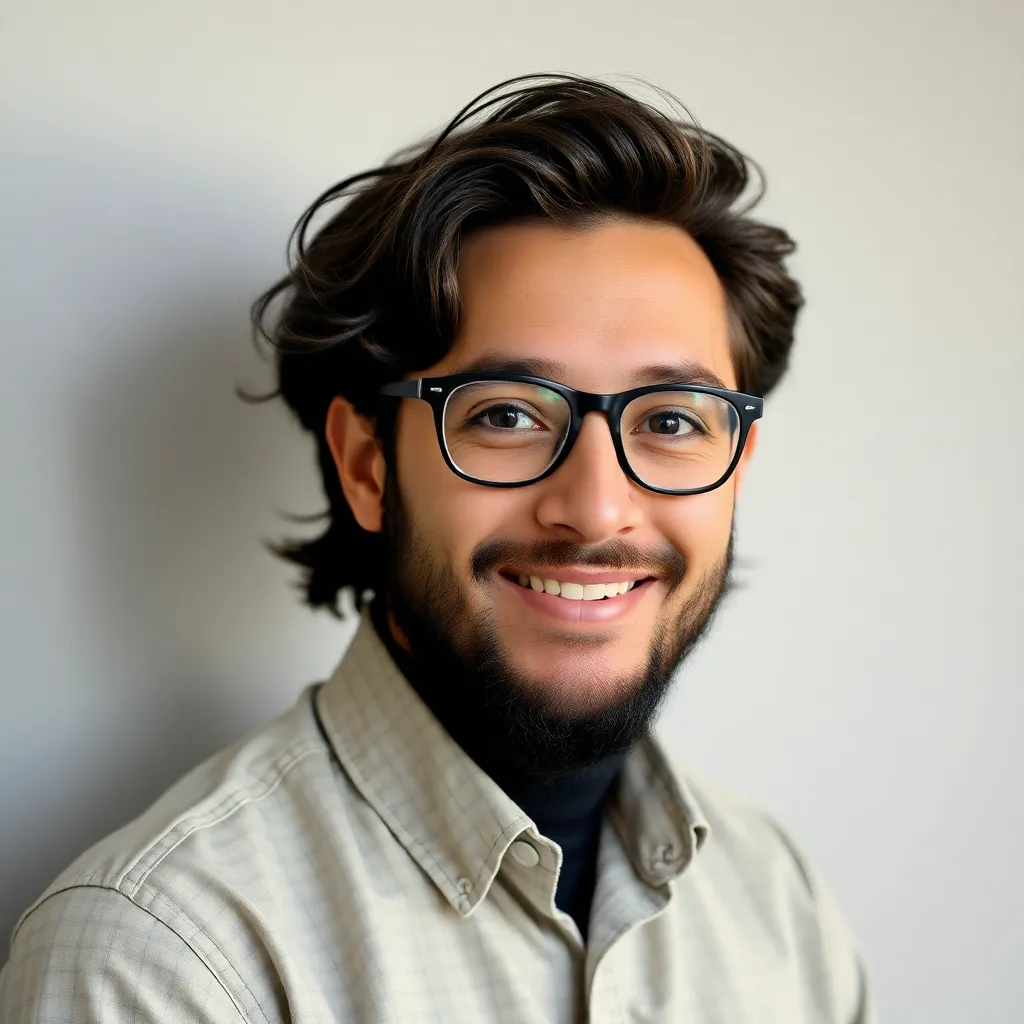
Onlines
May 09, 2025 · 6 min read
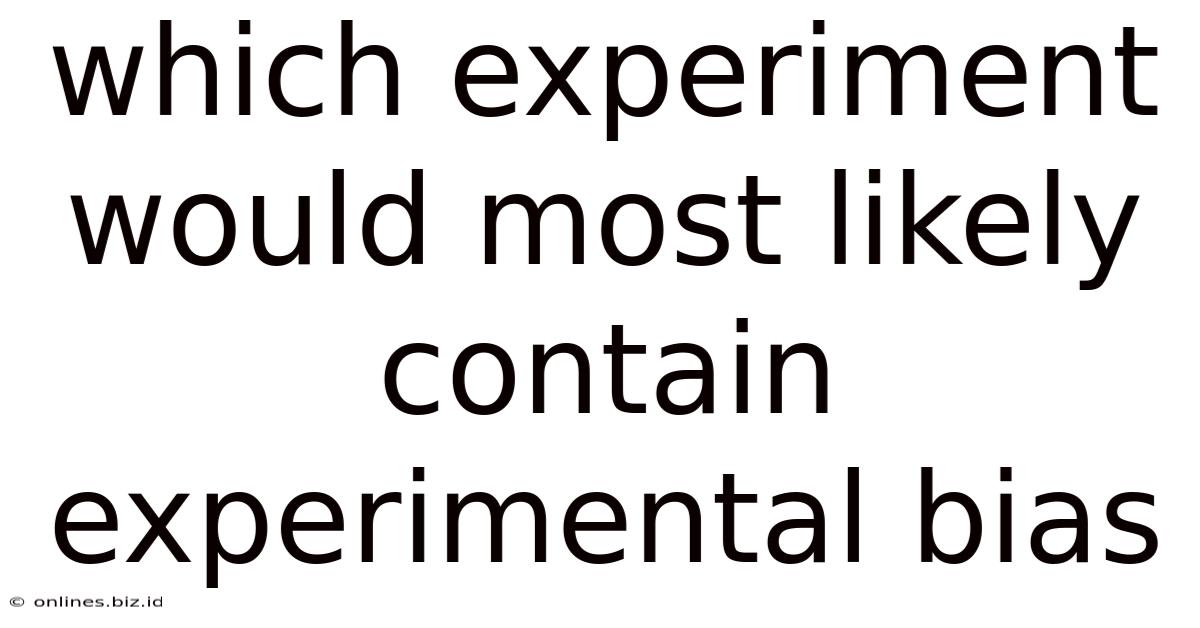
Table of Contents
Which Experiment Would Most Likely Contain Experimental Bias?
Experimental bias, also known as researcher bias, is a significant threat to the validity of research findings. It occurs when the researcher's expectations or preferences influence the results of the study, either consciously or unconsciously. This can manifest in various ways, from subtle influences on participant behavior to outright manipulation of data. Understanding the factors that increase the likelihood of experimental bias is crucial for designing robust and reliable experiments. This article explores various experimental designs and methodologies, highlighting those most susceptible to bias and providing strategies for mitigation.
Understanding the Roots of Experimental Bias
Before delving into specific experiments, it's essential to understand the mechanisms that drive experimental bias. These mechanisms are multifaceted and often interconnected:
1. Confirmation Bias:
This is arguably the most pervasive form of bias. Researchers, consciously or unconsciously, might favor data that supports their pre-existing hypotheses and downplay or ignore contradictory evidence. This can involve selectively choosing participants, manipulating experimental procedures, or interpreting data in a biased manner.
2. Observer Bias:
This occurs when the researcher's observations are influenced by their expectations. For instance, in a study evaluating a new treatment, a researcher expecting positive results might unintentionally interpret ambiguous observations favorably, while overlooking negative outcomes. This is particularly problematic in observational studies where subjective interpretations are involved.
3. Experimenter Expectancy Effect:
Researchers can inadvertently communicate their expectations to participants, influencing their behavior. This can be through subtle cues like body language, tone of voice, or even the phrasing of instructions. Participants might subconsciously try to please the researcher by behaving in a way that aligns with the expected results.
4. Sampling Bias:
The selection of participants can significantly impact the results. If the sample isn't representative of the target population, the findings might not be generalizable. For example, recruiting participants primarily from one demographic group could lead to biased conclusions.
5. Measurement Bias:
The tools and methods used to collect data can introduce bias. Poorly designed questionnaires, subjective rating scales, or unreliable measurement instruments can lead to inaccurate and biased results.
Experiments Prone to Experimental Bias:
Several types of experiments are inherently more susceptible to experimental bias than others. Let's examine some key examples:
1. Single-Blind Studies:
In single-blind studies, participants are unaware of their assigned condition (e.g., treatment or control group). While this reduces participant bias, it doesn't eliminate experimenter bias. The researcher, knowing the group assignments, might still inadvertently influence the participants' behavior or the data interpretation. This makes single-blind studies relatively vulnerable to bias compared to double-blind designs.
Example: A study testing the effectiveness of a new pain relief medication. The researcher, knowing which participants receive the medication and which receive a placebo, might unconsciously treat the medication group differently, impacting the results.
2. Studies with Subjective Outcomes:
Experiments relying on subjective measures, such as self-reported questionnaires or observational assessments of behavior, are inherently prone to bias. The researcher's interpretation of the data can be heavily influenced by their expectations, leading to skewed results.
Example: A study evaluating the effectiveness of a therapy program based solely on therapists' subjective ratings of patient improvement. The therapists' belief in the program's effectiveness might influence their assessment of patient progress.
3. Studies with Small Sample Sizes:
Studies with limited participants are more vulnerable to bias because a few outliers or anomalies can disproportionately affect the results. A small sample size reduces the statistical power of the study, making it more difficult to detect true effects and more susceptible to the influence of extraneous factors.
Example: A study investigating the effects of a new teaching method on student achievement with only ten participants in each group. One or two unusually high or low performing students in either group could significantly skew the overall results.
4. Studies Involving Inter-Rater Reliability:
When multiple researchers are involved in data collection or analysis, discrepancies in their interpretation or application of methods can lead to bias. Lack of clear guidelines or insufficient training can amplify this problem, leading to inconsistent and unreliable results.
Example: A study analyzing video recordings of children's play behavior, where multiple observers independently code the behavior. Differences in their interpretation of the coding scheme can introduce bias, affecting the study's conclusions.
5. Quasi-Experimental Designs:
These designs lack the random assignment of participants to groups, a cornerstone of rigorous experimental design. The absence of random assignment increases the likelihood of pre-existing differences between groups that could confound the results and be misinterpreted as treatment effects.
Example: A study comparing student achievement in two different schools, one employing a new teaching method and the other using the traditional method. Differences in student demographics, teacher experience, or school resources could influence the results, making it difficult to isolate the impact of the teaching method.
Minimizing Experimental Bias:
While some experiments are inherently more vulnerable to bias, researchers can take proactive steps to minimize its impact. Key strategies include:
-
Double-Blind Studies: In double-blind studies, neither the participants nor the researchers know the group assignments. This effectively eliminates both participant and experimenter expectancy effects, providing a strong safeguard against bias.
-
Standardized Procedures: Establishing clear and detailed procedures for every step of the experiment, from participant recruitment to data analysis, ensures consistency and minimizes the opportunity for subjective interpretations.
-
Objective Measurement Tools: Using validated and reliable instruments to collect data reduces the influence of subjective judgment. This is especially crucial for studies involving subjective outcomes.
-
Large Sample Sizes: Larger samples increase the statistical power of the study, making it less vulnerable to the influence of outliers or chance variations.
-
Blinding of Data Analysts: If possible, data analysts should be blinded to the group assignments of participants to avoid biased interpretation of the results.
-
Randomization: Randomly assigning participants to groups helps to ensure that any pre-existing differences between the groups are evenly distributed, minimizing the risk of confounding variables.
-
Triangulation: Employing multiple methods or measures to assess the same outcome can help to identify and minimize bias. If consistent results are obtained using different methods, confidence in the findings increases.
-
Peer Review: Seeking feedback from independent reviewers helps to identify potential sources of bias and ensure the rigor of the study.
Conclusion:
Experimental bias is a significant concern in research, potentially undermining the validity of findings. Experiments involving subjective measures, small sample sizes, single-blind designs, and quasi-experimental setups are particularly susceptible to bias. By implementing rigorous methodological strategies such as double-blinding, standardized procedures, objective measurements, and large sample sizes, researchers can significantly reduce the risk of bias and enhance the credibility of their research. A vigilant and critical approach to experimental design is crucial for generating reliable and trustworthy results that advance scientific understanding. The awareness and proactive mitigation of bias are not just methodological niceties; they are essential for the integrity of the scientific process and the responsible application of research findings. Continuous reflection on potential sources of bias, coupled with a commitment to methodological rigor, remains paramount in the pursuit of robust and meaningful research.
Latest Posts
Latest Posts
-
1 H Relationships Between Position Velocity And Acceleration
May 09, 2025
-
Which Of The Following Is True Of Disparate Impact Discrimination
May 09, 2025
-
Select The Phrases That Describe The Plasma Membrane
May 09, 2025
-
Which Food Truck Has The Absolute Advantage
May 09, 2025
-
Utilitarians Can Be Criticized For Which Of The Following
May 09, 2025
Related Post
Thank you for visiting our website which covers about Which Experiment Would Most Likely Contain Experimental Bias . We hope the information provided has been useful to you. Feel free to contact us if you have any questions or need further assistance. See you next time and don't miss to bookmark.