Which Histograms Shown Below Are Skewed To The Left
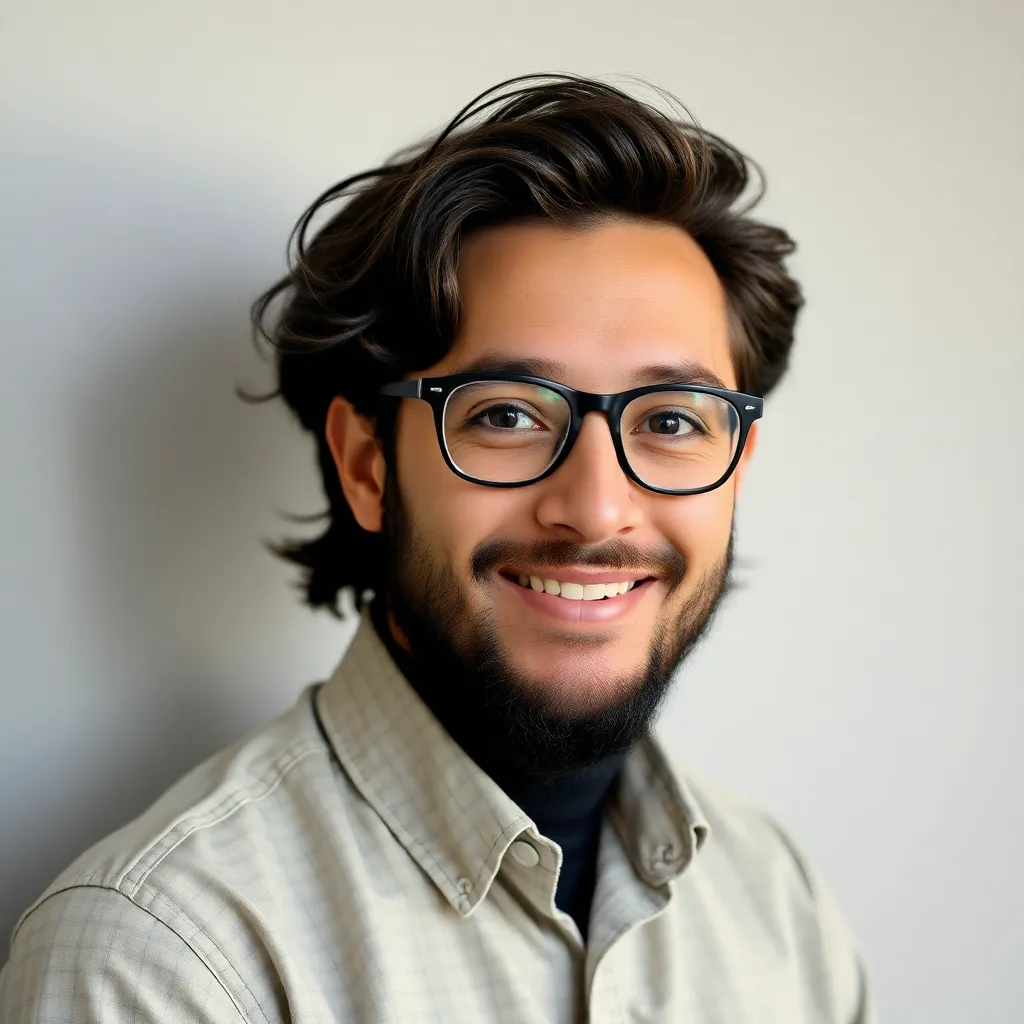
Onlines
Apr 08, 2025 · 6 min read

Table of Contents
Which Histograms Shown Below Are Skewed to the Left? A Comprehensive Guide
Understanding data distribution is crucial in statistics and data analysis. One of the key aspects of analyzing data distribution is identifying the skewness, which indicates the asymmetry of the distribution. This article will delve into the concept of left-skewed histograms, explaining what they are, how to identify them, and providing examples to clarify the concept. We will also explore the implications of left skewness and how it impacts statistical analysis.
Understanding Skewness in Histograms
A histogram is a graphical representation of the distribution of numerical data. It displays data using bars of varying heights, where the height of each bar corresponds to the frequency of data points falling within a specific range or bin. Skewness measures the asymmetry of this distribution.
There are three primary types of skewness:
- Symmetrical: The data is evenly distributed around the mean. The left and right sides of the histogram are mirror images of each other.
- Right-Skewed (Positive Skewness): The tail of the distribution extends to the right. The mean is typically greater than the median. This often indicates that there are a few extremely high values influencing the mean.
- Left-Skewed (Negative Skewness): The tail of the distribution extends to the left. The mean is typically less than the median. This indicates the presence of a few extremely low values pulling the mean downwards.
Identifying Left-Skewed Histograms: Key Characteristics
A left-skewed histogram, also known as negatively skewed, is characterized by several key features:
- Long Left Tail: The most prominent feature is a long tail extending to the left. This tail represents a smaller number of observations with unusually low values.
- Mean < Median: The mean (average) will be less than the median (middle value). This is because the few extremely low values pull the mean downwards, while the median is less affected by these outliers.
- Mode > Median > Mean: Typically, the mode (most frequent value) will be greater than the median, which in turn is greater than the mean. This ordering provides a clear indication of left skewness.
- Concentration of Data on the Right: A large proportion of the data will be clustered on the right side of the histogram, creating a peak near the higher values.
- Asymmetrical Shape: The overall shape of the histogram is not symmetrical; it's distinctly asymmetrical, with a longer tail on the left.
Examples of Left-Skewed Histograms
While I cannot display actual histograms here, I can describe several scenarios that would produce left-skewed distributions. Imagine analyzing the following data sets:
-
Test Scores with Easy Questions: If you give a test with very easy questions, many students will score perfectly or near-perfectly. A few students, however, might struggle and score very low. This would result in a left-skewed distribution, with the majority of scores clustered near the high end and a long tail on the left representing the lower scores.
-
Income Distribution in a Wealthy Country: In a country with a significant wealth gap, the income distribution might show left skewness. A vast number of people will have moderately high incomes, while a small number of individuals will have extremely low incomes, leading to a left-leaning tail.
-
Age of Participants in a Marathon: Consider the age distribution of runners in a marathon. The majority of participants might be between 25 and 45 years old. However, you might have a few very young or very old participants. These extreme values on the younger side could lead to a left-skewed distribution if the number of very young runners is considerably smaller than those in their prime running age.
-
Exam Scores on a Very Difficult Exam: If a test is exceedingly difficult, most students will achieve lower scores. A small number might achieve high scores, creating a left-skewed distribution.
-
Customer Satisfaction Ratings for a High-Quality Product: Suppose a company produces a high-quality product. Most customers will likely give high ratings. However, a few dissatisfied customers will give very low ratings, resulting in left skewness.
Differentiating Between Left and Right Skewness
It's crucial to be able to distinguish between left and right skewness. Here’s a table summarizing the key differences:
Feature | Left-Skewed (Negative Skewness) | Right-Skewed (Positive Skewness) |
---|---|---|
Tail Direction | Long tail on the left | Long tail on the right |
Mean vs. Median | Mean < Median | Mean > Median |
Mode vs. Median | Mode > Median | Mode < Median |
Data Concentration | Clustered on the right | Clustered on the left |
Outliers | Low outliers | High outliers |
Implications of Left Skewness
Understanding the presence of left skewness has significant implications for data analysis and interpretation:
-
Mean vs. Median: When dealing with left-skewed data, using the median is generally more representative of the central tendency than the mean. The mean is highly sensitive to the few extreme low values.
-
Statistical Tests: The choice of statistical test might need to be adjusted based on the skewness of the data. Some tests assume a normal (symmetrical) distribution, and using them with severely skewed data can lead to inaccurate results. Transformations, such as logarithmic transformations, might be necessary to normalize the data.
-
Data Interpretation: The presence of left skewness indicates the presence of low-value outliers that might need further investigation. These outliers could be indicative of errors in data collection or unusual circumstances. Understanding their source is crucial for proper interpretation of the overall data.
Real-World Applications
Left skewness appears in various real-world situations beyond those already mentioned:
-
Healthcare: Analyzing patient recovery times after surgery might show left skewness if most patients recover quickly, with a few experiencing significantly longer recovery periods.
-
Finance: Analyzing investment returns could exhibit left skewness if most investments yield moderate gains, with a few experiencing substantial losses.
-
Manufacturing: Analyzing the lifespan of manufactured products might display left skewness if most products have long lifespans, and a small fraction fails prematurely.
Conclusion
Identifying left-skewed histograms is essential for accurate data interpretation and meaningful statistical analysis. By understanding the characteristics of left-skewed distributions, including the presence of a long left tail, the relationship between the mean, median, and mode, and the concentration of data on the right side, we can effectively analyze data and draw meaningful conclusions. Remember that the presence of skewness, whether left or right, indicates that the data deviates from a perfect symmetrical normal distribution, requiring careful consideration during interpretation and analysis. Always remember to consider the context of your data and the implications of skewness on your conclusions.
Latest Posts
Latest Posts
-
Desert Locusts Are Devastating To Agriculture Because
Apr 17, 2025
-
Pedagogy Of The Oppressed Chapter 2
Apr 17, 2025
-
Factoring Trinomials Math Lib Answer Key
Apr 17, 2025
-
Believes In Reincarnation And Prescribes A Vegetarian Diet
Apr 17, 2025
-
What Cause And Effect Relationship Do These Headlines Suggest
Apr 17, 2025
Related Post
Thank you for visiting our website which covers about Which Histograms Shown Below Are Skewed To The Left . We hope the information provided has been useful to you. Feel free to contact us if you have any questions or need further assistance. See you next time and don't miss to bookmark.