Which Of The Following Are Characteristics Of Raw Data
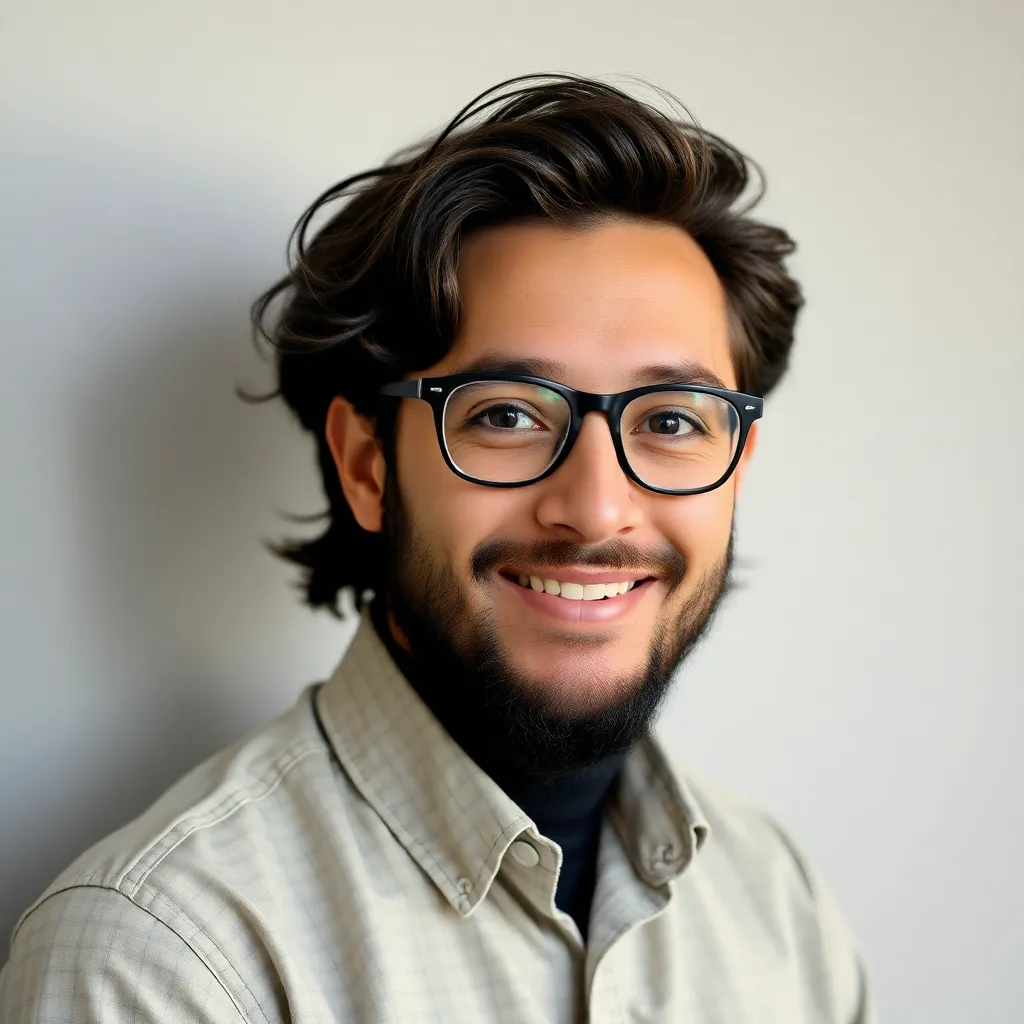
Onlines
Apr 20, 2025 · 6 min read

Table of Contents
Which of the Following are Characteristics of Raw Data? A Deep Dive into Data's Unrefined State
Raw data. The term itself conjures images of unorganized chaos, a sprawling mess of numbers, text, and symbols waiting to be tamed. But understanding the characteristics of raw data is fundamental to anyone working with data, from data scientists and analysts to business owners and marketers. This comprehensive guide will explore the key traits that define raw data and why understanding these characteristics is crucial for effective data analysis and decision-making.
Defining Raw Data: The Untouched Foundation
Before diving into the specific characteristics, let's establish a clear definition. Raw data refers to information that has not been processed, organized, structured, or interpreted in any way. It’s the initial form of data collected, typically in its most basic and unrefined state. Think of it as the raw ingredients before they're transformed into a delicious meal. Without processing, this data lacks context, meaning, and readily usable insights.
Key Characteristics of Raw Data
Raw data possesses several defining characteristics:
1. Unorganized and Unstructured: The Untamed Wilderness
Perhaps the most prominent characteristic is its lack of organization and structure. Raw data often arrives in a disorganized manner, lacking a predefined format or schema. This could manifest as:
- Scattered files: Data might be spread across multiple files, spreadsheets, or databases, making integration and analysis challenging.
- Inconsistent formats: Different files might use varying data formats, making it difficult to combine them seamlessly. Imagine one file using comma separation, another using tabs, and yet another using a proprietary format.
- Missing values: Gaps in the data are common. Some values might be missing entirely, creating incomplete records.
- Inaccurate or inconsistent entries: Human error during data entry can lead to inconsistencies and inaccuracies, further complicating the analysis process.
2. Unprocessed and Unanalyzed: The Untapped Potential
Raw data is, by definition, unprocessed and unanalyzed. It hasn't undergone any transformations, calculations, or interpretations. This means it lacks the context and insights that processing can provide. For instance:
- No calculations performed: Summations, averages, or other statistical calculations haven't been applied.
- No data cleaning: Errors, outliers, or inconsistencies haven't been identified and corrected.
- No transformations: Data hasn't been converted into a more usable format, such as aggregation or normalization.
- No filtering or selection: No specific subsets of data have been isolated for analysis.
This untouched state is both a challenge and an opportunity. The challenge lies in the effort required to clean and prepare it. The opportunity lies in the potential for undiscovered insights hidden within its raw form.
3. Voluminous and Diverse: Big Data's Humble Beginnings
In today's digital age, raw data is often voluminous and diverse. We generate massive amounts of data from various sources:
- Transactional data: Sales records, financial transactions, website activity.
- Sensor data: Measurements from environmental sensors, medical devices, industrial equipment.
- Social media data: Posts, comments, likes, shares, and other user interactions.
- Image and video data: Photos, videos, and other multimedia content.
- Textual data: Emails, documents, reports, and other written materials.
This diversity in data types presents unique challenges in storage, processing, and analysis. Handling large volumes of diverse data requires specialized tools and techniques.
4. Potentially Inaccurate and Incomplete: The Need for Data Cleaning
Raw data is inherently prone to inaccuracies and incompleteness. Human error, faulty equipment, or limitations in data collection methods can all contribute to problems:
- Data entry errors: Typos, incorrect values, or missing entries.
- Measurement errors: Faulty instruments or inconsistent measurement techniques.
- Sampling bias: Data collected from a non-representative sample may not accurately reflect the population.
- Data corruption: Data may be corrupted during storage or transmission.
This necessitates careful data cleaning and validation procedures to ensure the accuracy and reliability of the analysis results.
5. Context-Dependent: Understanding the "Why"
Raw data, in its purest form, lacks inherent meaning. Its value lies in its context. Understanding where the data originated, how it was collected, and what it represents is crucial for correct interpretation. Consider these aspects:
- Data source: Knowing the source of the data helps determine its reliability and validity.
- Data collection methods: Understanding the methods used to collect the data can highlight potential biases or limitations.
- Data definitions: Clear definitions of variables and their units are necessary to prevent misinterpretations.
- Data limitations: Awareness of any limitations or constraints in the data is essential for drawing valid conclusions.
Without understanding the context, raw data is essentially meaningless. It's like having a puzzle without the picture on the box – you have the pieces, but you don't know what the final image should look like.
The Importance of Understanding Raw Data Characteristics
Understanding the characteristics of raw data is crucial for several reasons:
- Effective data cleaning: Recognizing the presence of inconsistencies, errors, and missing values allows for targeted data cleaning strategies.
- Appropriate data transformation: Knowing the data's structure and format helps select the appropriate transformation techniques to prepare it for analysis.
- Reliable data analysis: Understanding the data's limitations and biases enables more accurate and reliable analytical results.
- Meaningful insights: Correct interpretation of data requires a solid understanding of its context and origins.
- Data-driven decision-making: Only through careful analysis of properly prepared data can informed and effective decisions be made.
From Raw Data to Valuable Insights: The Data Transformation Journey
The journey from raw data to valuable insights is a process of transformation and refinement. This process typically involves several stages:
- Data Collection: Gathering data from various sources.
- Data Cleaning: Addressing missing values, inconsistencies, and errors.
- Data Transformation: Converting data into a suitable format for analysis, such as normalization or aggregation.
- Data Exploration: Examining the data to identify patterns and relationships.
- Data Analysis: Applying statistical methods or machine learning algorithms to extract insights.
- Data Visualization: Presenting the findings in a clear and understandable way using charts and graphs.
- Interpretation and Conclusion: Drawing meaningful conclusions and making informed decisions based on the analysis results.
Conclusion: Embracing the Power of Raw Data
Raw data, while seemingly chaotic in its initial state, holds immense potential for unlocking valuable insights and informing strategic decisions. By understanding its fundamental characteristics – its unorganized nature, unprocessed state, potential for inaccuracies, and context dependency – we can effectively navigate the process of transforming it into actionable intelligence. Mastering this process is key to harnessing the power of data in today's increasingly data-driven world. Remember, the journey from raw data to valuable insights is not just about the destination, but also the careful and methodical steps taken along the way. A thorough understanding of the characteristics of raw data is the essential first step in that journey.
Latest Posts
Latest Posts
-
Experiment 34 An Equilibrium Constant Pre Lab Answers
Apr 20, 2025
-
Unit 5 Relationships In Triangles Homework 4
Apr 20, 2025
-
Catcher In The Rye Summary Chapter 9
Apr 20, 2025
-
Overall You Need To Take Responsibility For Your Physical Fitness
Apr 20, 2025
-
Create A Scaled Annotated Drawing Of The First Class Lever
Apr 20, 2025
Related Post
Thank you for visiting our website which covers about Which Of The Following Are Characteristics Of Raw Data . We hope the information provided has been useful to you. Feel free to contact us if you have any questions or need further assistance. See you next time and don't miss to bookmark.