Which Of The Following Describes The Probability Distribution Below
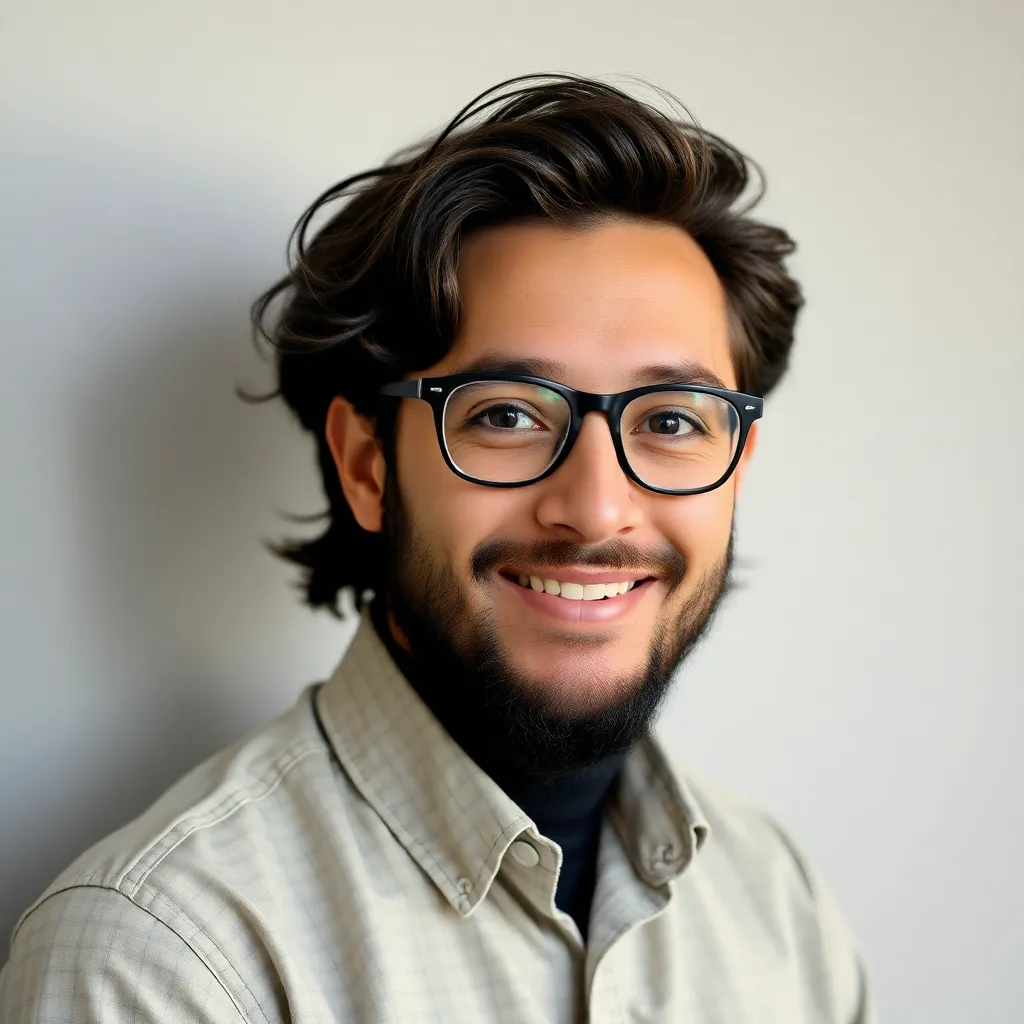
Onlines
May 11, 2025 · 6 min read
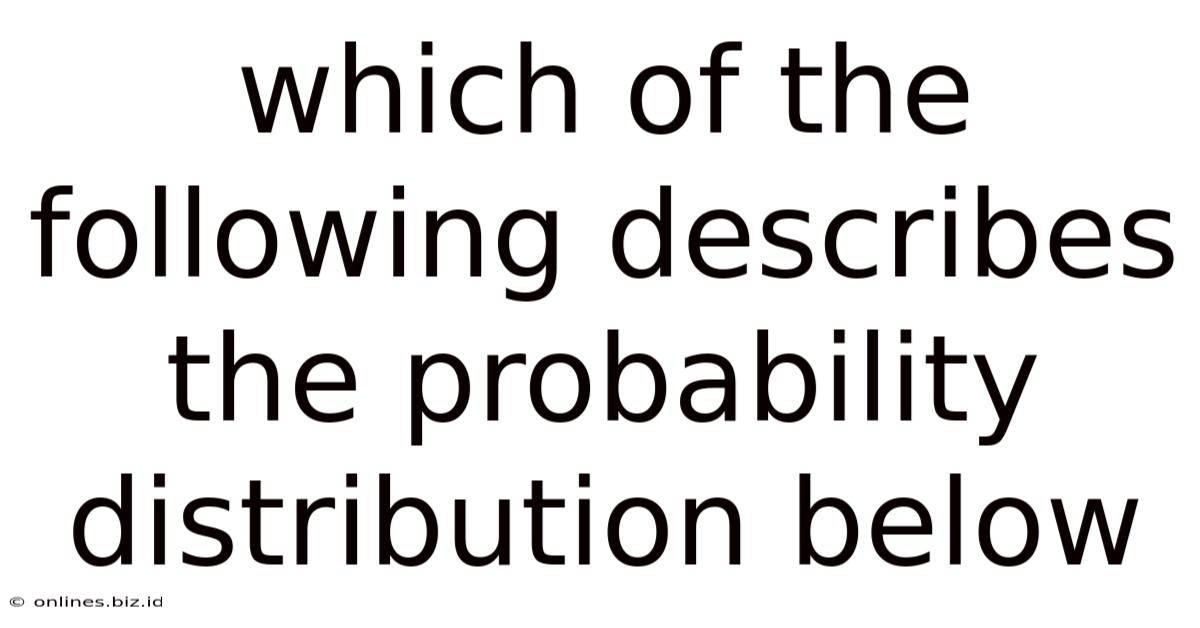
Table of Contents
Decoding Probability Distributions: A Comprehensive Guide
Understanding probability distributions is crucial in various fields, from statistics and data science to finance and engineering. This comprehensive guide delves into the characteristics of different probability distributions, equipping you with the knowledge to identify and interpret them effectively. We will explore key concepts and techniques to help you confidently determine which distribution best describes a given dataset or scenario.
What is a Probability Distribution?
A probability distribution is a mathematical function that provides the probabilities of occurrence of different possible outcomes for a random variable. It essentially maps each possible outcome of a random variable to its probability. These distributions can be either discrete or continuous.
-
Discrete Probability Distributions: These deal with discrete random variables, which can only take on a finite number of values or a countably infinite number of values. Examples include the binomial distribution, Poisson distribution, and geometric distribution.
-
Continuous Probability Distributions: These deal with continuous random variables, which can take on any value within a given range. Examples include the normal distribution, exponential distribution, and uniform distribution.
Key Characteristics of Probability Distributions
Several key characteristics help us distinguish between different probability distributions:
-
Mean (Expected Value): The average value of the random variable. It represents the central tendency of the distribution.
-
Variance: A measure of how spread out the distribution is. A higher variance indicates greater variability.
-
Standard Deviation: The square root of the variance. It provides a more interpretable measure of the spread in the same units as the random variable.
-
Skewness: A measure of the asymmetry of the distribution. A positive skew indicates a longer tail on the right, while a negative skew indicates a longer tail on the left.
-
Kurtosis: A measure of the "tailedness" of the probability distribution. High kurtosis indicates heavy tails and sharp peaks, while low kurtosis indicates light tails and a flat distribution.
Common Probability Distributions and Their Applications
Let's explore some of the most commonly encountered probability distributions:
1. Normal Distribution (Gaussian Distribution)
The normal distribution, often depicted by its bell curve, is arguably the most important distribution in statistics. It's characterized by its symmetry, with the mean, median, and mode all coinciding at the center.
Characteristics:
- Symmetrical: The distribution is perfectly symmetrical around its mean.
- Unimodal: It has a single peak.
- Defined by Mean (μ) and Standard Deviation (σ): These two parameters completely define the shape and location of the distribution.
Applications:
The normal distribution is widely used to model numerous real-world phenomena, including:
- Heights and weights of individuals: Human height and weight often follow a normal distribution.
- Measurement errors: Errors in measurement processes are frequently normally distributed.
- Test scores: Standardized test scores often approximate a normal distribution.
- Financial data: Stock prices and returns can sometimes be approximated by a normal distribution (though this is often debated).
Identifying a Normal Distribution:
Visually inspecting a histogram or Q-Q plot can help determine if a dataset follows a normal distribution. Statistical tests like the Shapiro-Wilk test or Kolmogorov-Smirnov test can provide a more formal assessment.
2. Binomial Distribution
The binomial distribution models the probability of getting a certain number of successes in a fixed number of independent Bernoulli trials (trials with only two possible outcomes, success or failure).
Characteristics:
- Fixed number of trials (n): The number of trials is predetermined.
- Independent trials: The outcome of one trial does not affect the outcome of any other trial.
- Constant probability of success (p): The probability of success remains the same for each trial.
Applications:
- Quality control: Determining the probability of finding a certain number of defective items in a sample.
- Medical research: Assessing the probability of a successful treatment outcome in a clinical trial.
- Polling: Estimating the proportion of individuals who support a particular candidate.
3. Poisson Distribution
The Poisson distribution describes the probability of a given number of events occurring in a fixed interval of time or space if these events occur with a known average rate and independently of the time since the last event.
Characteristics:
- Events are independent: The occurrence of one event doesn't affect the probability of another event.
- Events occur at a constant average rate (λ): The average rate of events is known.
Applications:
- Customer arrivals: Modeling the number of customers arriving at a store in a given hour.
- Website traffic: Predicting the number of website visitors in a specific time period.
- Insurance claims: Estimating the number of insurance claims received in a particular period.
4. Exponential Distribution
The exponential distribution models the time between events in a Poisson process. It's characterized by its memorylessness property – the probability of an event occurring in the next time interval is independent of how much time has already elapsed.
Characteristics:
- Memoryless: The future behavior of the random variable is independent of its past behavior.
- Defined by a single parameter (λ): This parameter represents the rate parameter.
Applications:
- Reliability engineering: Modeling the lifespan of a component or system.
- Queueing theory: Analyzing waiting times in queues.
- Survival analysis: Studying the time until an event (e.g., death, failure) occurs.
5. Uniform Distribution
The uniform distribution assigns equal probability to all outcomes within a given range.
Characteristics:
- Equal probability: Every value within the specified range has the same probability of occurring.
- Defined by a minimum (a) and maximum (b): These parameters determine the range of the distribution.
Applications:
- Random number generation: Generating random numbers within a specified range.
- Simulation: Simulating scenarios where all outcomes are equally likely.
- Modeling uncertainty: Representing situations where there's limited information about the possible outcomes.
Identifying the Correct Probability Distribution: A Step-by-Step Approach
Determining the appropriate probability distribution for a given dataset or scenario involves a combination of visual inspection, statistical tests, and understanding the underlying process generating the data.
1. Visual Inspection:
Begin by creating a histogram or other visual representation of the data. This gives an initial impression of the distribution's shape, including its symmetry, modality, and potential skewness.
2. Statistical Summary:
Calculate key descriptive statistics such as the mean, median, variance, standard deviation, skewness, and kurtosis. These measures provide quantitative information about the distribution's central tendency, variability, and shape.
3. Probability Plots (Q-Q Plots):
Probability plots, particularly quantile-quantile (Q-Q) plots, compare the quantiles of your data to the quantiles of a theoretical distribution. If the data points closely follow a straight line on the Q-Q plot, this suggests a good fit to the theoretical distribution.
4. Statistical Tests:
Formal statistical tests, like the Shapiro-Wilk test for normality or goodness-of-fit tests for other distributions, can help assess the degree to which the data conforms to a specific distribution. Remember that no test is perfect; it's crucial to consider the context and limitations of the test.
5. Understanding the Underlying Process:
Consider the nature of the data-generating process. Is it a series of independent trials? Is there a constant rate of events? Understanding the underlying mechanism can help you choose a distribution that aligns with the data's characteristics.
Conclusion
Identifying the correct probability distribution is crucial for effective data analysis and statistical modeling. By employing a combination of visual inspection, descriptive statistics, probability plots, and appropriate statistical tests, you can confidently determine which distribution best represents your data and draw meaningful conclusions from your analysis. Remember that context and the underlying data generating process are key factors to consider throughout this process. This detailed guide provides a solid foundation for tackling various probability distribution challenges. Further exploration of specific distributions and their applications will enhance your skillset even further.
Latest Posts
Latest Posts
-
In This Excerpt Natural Is Used To Describe People Who
May 12, 2025
-
Use Your Knowledge Of Cost Functions
May 12, 2025
-
Smile Brands Finance Inc Credit Application
May 12, 2025
-
Fitness That Improves Your Ability To Learn Skills Is Called
May 12, 2025
-
Which Of The Following Is Not A Constituency Building Strategy Tool
May 12, 2025
Related Post
Thank you for visiting our website which covers about Which Of The Following Describes The Probability Distribution Below . We hope the information provided has been useful to you. Feel free to contact us if you have any questions or need further assistance. See you next time and don't miss to bookmark.