Which Of The Following Is Correct About A Probability Distribution
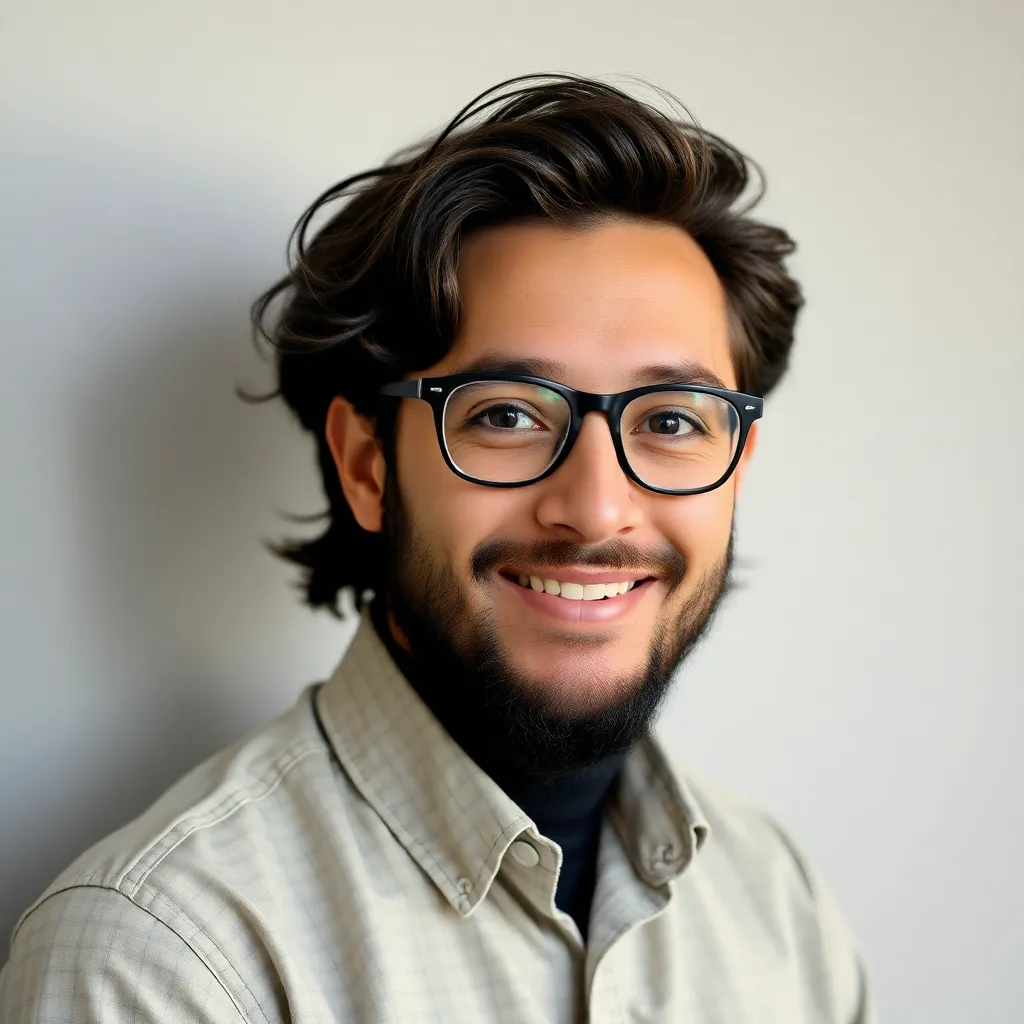
Onlines
May 10, 2025 · 6 min read
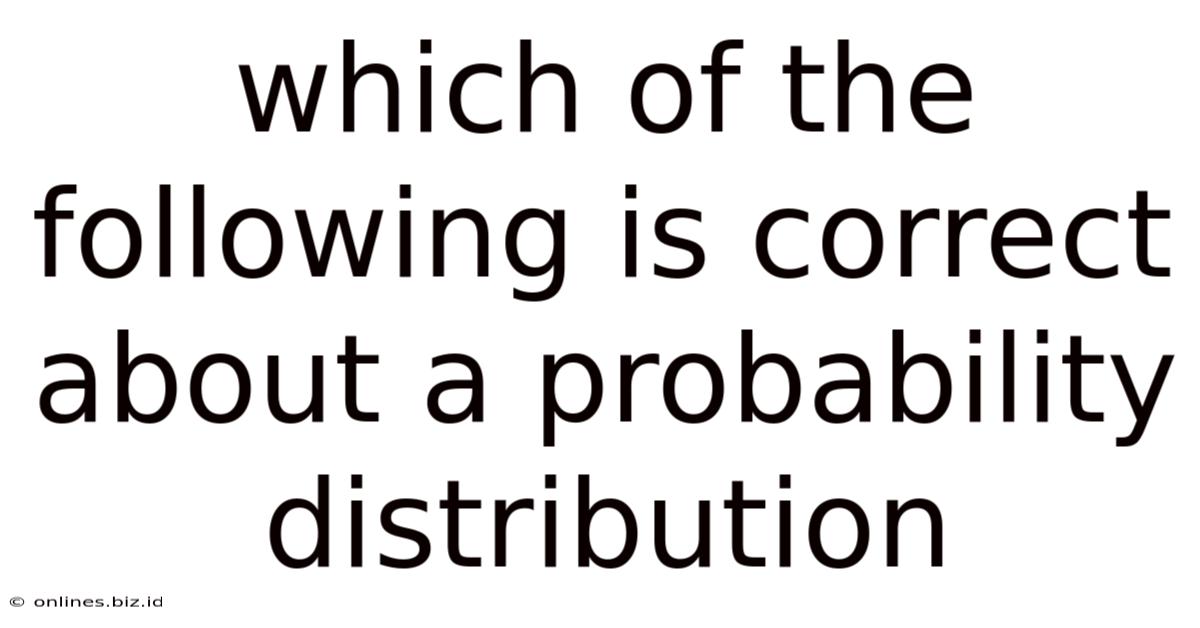
Table of Contents
Which of the Following is Correct About a Probability Distribution? A Comprehensive Guide
Understanding probability distributions is fundamental to numerous fields, from statistics and machine learning to finance and physics. This comprehensive guide delves into the core concepts of probability distributions, clarifying common misconceptions and providing a robust understanding of their properties. We'll explore various types of probability distributions and address the crucial question: which statements about probability distributions are correct?
What is a Probability Distribution?
A probability distribution describes the likelihood of obtaining possible values for a random variable. Instead of simply stating the probability of a single event, it provides a complete picture of all possible outcomes and their associated probabilities. This is crucial because it allows us to make predictions about future events and understand the inherent uncertainty associated with them. Think of it as a roadmap of probabilities, guiding our understanding of random phenomena.
There are two main types of probability distributions:
1. Discrete Probability Distributions
These distributions deal with discrete random variables – variables that can only take on a finite number of values or a countably infinite number of values. Examples include:
- The Binomial Distribution: Models the probability of a certain number of successes in a fixed number of independent Bernoulli trials (e.g., coin flips). Key parameters are the number of trials (n) and the probability of success in a single trial (p).
- The Poisson Distribution: Describes the probability of a given number of events occurring in a fixed interval of time or space, given the average rate of occurrence (λ). It's often used to model rare events.
- The Bernoulli Distribution: This is the simplest discrete distribution, representing the probability of success or failure in a single trial.
2. Continuous Probability Distributions
These distributions describe continuous random variables – variables that can take on any value within a given range. Examples include:
- The Normal Distribution (Gaussian Distribution): The most widely used continuous distribution, characterized by its bell-shaped curve. It's defined by its mean (μ) and standard deviation (σ). Many natural phenomena approximately follow a normal distribution.
- The Exponential Distribution: Models the time until an event occurs in a Poisson process (e.g., time between customer arrivals). It's characterized by a single parameter, λ, representing the rate of events.
- The Uniform Distribution: Assigns equal probability to all values within a specified range.
- The Beta Distribution: A flexible distribution defined on the interval [0, 1], often used to model probabilities or proportions.
- The Gamma Distribution: A versatile distribution used to model positive, continuous random variables; it generalizes the exponential distribution.
Key Properties of Probability Distributions
Regardless of whether a distribution is discrete or continuous, several key properties define it:
- Probability Mass Function (PMF) (for discrete distributions): This function assigns a probability to each possible value of the random variable. The sum of all probabilities must equal 1.
- Probability Density Function (PDF) (for continuous distributions): This function describes the relative likelihood of the random variable taking on a given value. The area under the PDF curve over a given interval represents the probability of the random variable falling within that interval. The total area under the curve must equal 1.
- Cumulative Distribution Function (CDF) (for both discrete and continuous distributions): This function gives the probability that the random variable is less than or equal to a given value. The CDF is always non-decreasing and ranges from 0 to 1.
- Mean (Expected Value): The average value of the random variable, weighted by its probability.
- Variance: A measure of the spread or dispersion of the distribution around its mean. The square root of the variance is the standard deviation.
- Skewness: A measure of the asymmetry of the distribution. A positive skew indicates a longer tail on the right, while a negative skew indicates a longer tail on the left.
- Kurtosis: A measure of the "tailedness" of the probability distribution. High kurtosis indicates heavy tails and sharp peaks, while low kurtosis indicates light tails and a flatter distribution.
Correct Statements About Probability Distributions
Now, let's address the central question: which statements about probability distributions are correct? To answer this, we need to consider various potential statements and evaluate their validity based on the properties outlined above. Here are some examples and explanations:
Correct Statement 1: The sum of probabilities for all possible outcomes in a discrete probability distribution must equal 1.
This is a fundamental axiom of probability. The total probability of all possible events must add up to 1, representing certainty. This ensures the distribution is properly normalized.
Correct Statement 2: The area under the probability density function (PDF) of a continuous probability distribution over its entire range must equal 1.
Similar to the discrete case, the total probability must sum to 1. For continuous distributions, this is represented by the area under the PDF curve.
Correct Statement 3: The cumulative distribution function (CDF) is always non-decreasing.
The CDF represents the accumulated probability up to a certain point. As we move along the x-axis, the probability can only increase or remain the same; it cannot decrease.
Correct Statement 4: The mean of a probability distribution represents the expected value of the random variable.
The mean is the weighted average of all possible values, where the weights are the corresponding probabilities. It's the value we would expect to observe on average over many repetitions of the experiment.
Correct Statement 5: The variance of a probability distribution measures the spread or dispersion of the data around the mean.
A larger variance indicates greater variability, while a smaller variance indicates data clustered tightly around the mean.
Incorrect Statement Examples (with explanations):
Incorrect Statement 1: All probability distributions are symmetrical.
Many distributions are symmetrical (like the normal distribution), but many others are not (e.g., exponential, chi-squared). Symmetry is a specific property, not a requirement for all probability distributions.
Incorrect Statement 2: The probability density function (PDF) can take values greater than 1.
While the probability cannot exceed 1, the value of the PDF at a specific point can be greater than 1 for continuous distributions. The integral (area under the curve) must equal 1. This is a common point of confusion.
Incorrect Statement 3: The cumulative distribution function (CDF) can be decreasing.
As explained earlier, the CDF is always non-decreasing. It represents accumulated probability, which can only increase or stay the same as we consider larger values of the random variable.
Incorrect Statement 4: All discrete probability distributions have a finite number of possible outcomes.
While many discrete distributions have a finite number of outcomes, some can have a countably infinite number of outcomes (e.g., the number of coin flips until the first heads).
Choosing the Correct Statements: A Practical Approach
When faced with multiple statements about probability distributions, systematically analyze each one:
- Identify the type of distribution: Is it discrete or continuous?
- Recall the key properties: Consider PMF, PDF, CDF, mean, variance, skewness, and kurtosis.
- Check for consistency: Does the statement align with the fundamental properties of probability distributions?
- Consider counterexamples: Can you think of a distribution that violates the statement?
By applying this methodical approach, you can confidently determine which statements accurately reflect the characteristics of probability distributions.
Conclusion
Understanding probability distributions is crucial for anyone working with data or modeling uncertainty. This guide has provided a comprehensive overview of key concepts, properties, and common misconceptions. By mastering these concepts, you can confidently analyze data, make predictions, and tackle complex problems involving randomness and uncertainty. Remember to always rigorously evaluate statements about probability distributions, using your knowledge of their fundamental properties and considering potential counterexamples. This critical thinking approach will solidify your understanding and enhance your ability to work effectively with probabilistic models.
Latest Posts
Latest Posts
-
Choose The Correct Translation For The Roots Adren O Adrenal O
May 10, 2025
-
Which Of The Following Best Describes A Symbiotic Relationship
May 10, 2025
-
Which Of The Following Setups Would Convert Centimeters To Feet
May 10, 2025
-
Which Of The Following Best Characterizes Jit Systems
May 10, 2025
-
If A Companys Social Media Followers Exhibit Greater Purchase Frequency
May 10, 2025
Related Post
Thank you for visiting our website which covers about Which Of The Following Is Correct About A Probability Distribution . We hope the information provided has been useful to you. Feel free to contact us if you have any questions or need further assistance. See you next time and don't miss to bookmark.