Which Of The Following Is True About Outliers
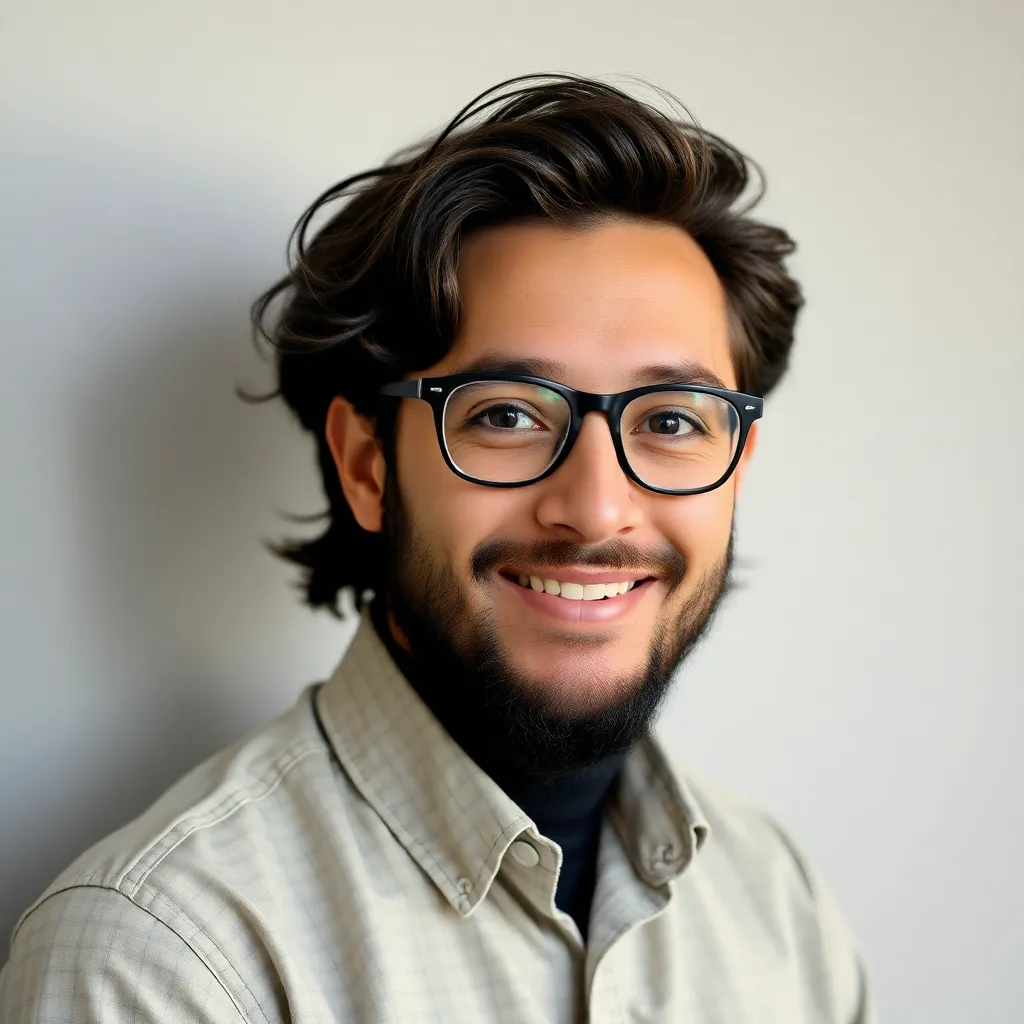
Onlines
May 10, 2025 · 6 min read
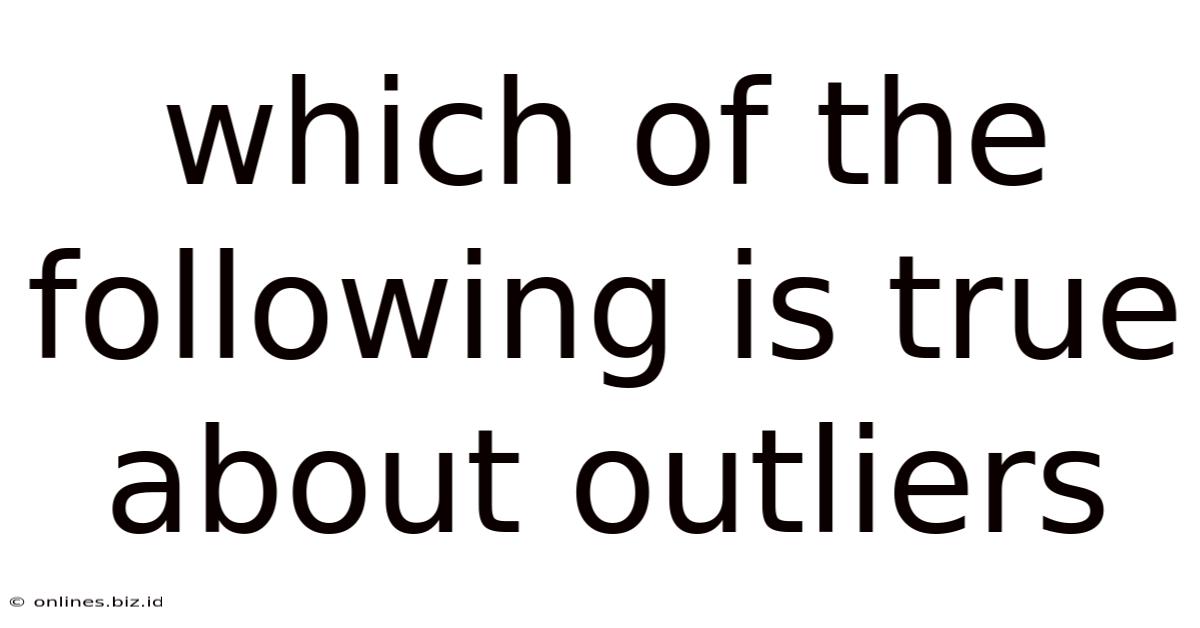
Table of Contents
Which of the Following is True About Outliers? Unveiling the Nature of Extreme Values
Outliers. Those data points that stubbornly refuse to conform to the general trend. They're the mischievous gnomes of the statistical world, sometimes causing chaos, sometimes revealing hidden truths. Understanding outliers is crucial for accurate data analysis and informed decision-making. This comprehensive guide delves deep into the nature of outliers, exploring their identification, impact, and appropriate handling.
What are Outliers? A Definition
Outliers are data points that significantly deviate from other observations in a dataset. They lie an unusual distance from other values in a distribution. It's important to note that "significantly deviate" isn't a precise mathematical definition; it depends heavily on the context and the specific dataset. A value that's an outlier in one dataset might be perfectly normal in another. This context-dependent nature makes identifying and handling outliers a nuanced task.
Identifying Outliers: Methods and Techniques
Several methods exist for identifying outliers, each with its strengths and weaknesses. Choosing the right method depends on the nature of your data and the research question.
1. Visual Inspection: The Power of Plots
The simplest method is visual inspection. Creating plots like box plots, scatter plots, and histograms allows you to visually identify data points that fall far outside the main cluster of data. Box plots are especially useful because they clearly show the quartiles and the interquartile range (IQR), highlighting values that lie beyond the whiskers (typically 1.5 times the IQR from the box edges).
Advantages: Simple, intuitive, and readily reveals potential outliers.
Disadvantages: Subjective; relies on visual interpretation, which can be biased. Less effective for high-dimensional data.
2. Z-score: Standard Deviation Approach
The Z-score measures how many standard deviations a data point is away from the mean. Data points with absolute Z-scores exceeding a certain threshold (often 2 or 3) are flagged as potential outliers. A Z-score of 2 indicates the data point is two standard deviations above the mean, while a Z-score of -2 indicates it's two standard deviations below.
Advantages: Objective, mathematically defined, easily calculated.
Disadvantages: Sensitive to non-normal distributions. Extreme values in a dataset can inflate the standard deviation, making it less effective in identifying outliers.
3. Interquartile Range (IQR): A Robust Method
The IQR method uses the difference between the third quartile (Q3) and the first quartile (Q1) of the data. Data points falling below Q1 - 1.5 * IQR or above Q3 + 1.5 * IQR are identified as outliers. This method is less sensitive to extreme values than the Z-score method because it only considers the middle 50% of the data.
Advantages: Robust to non-normal distributions, less influenced by extreme values than the Z-score.
Disadvantages: Can still be affected by skewed data; may miss outliers if the distribution is highly skewed.
4. Modified Z-score: Adapting to Non-normality
The modified Z-score addresses the limitations of the standard Z-score by using the median absolute deviation (MAD) instead of the standard deviation. This makes it more robust to non-normal distributions.
Advantages: Robust to non-normality, less sensitive to extreme values.
Disadvantages: Slightly more complex to calculate than the standard Z-score.
The Impact of Outliers: Understanding the Consequences
Outliers can have a significant impact on statistical analyses, particularly those involving measures of central tendency (mean, median) and measures of dispersion (variance, standard deviation).
1. Distortion of the Mean: The Shifting Average
Outliers disproportionately affect the mean. A single extremely high or low value can significantly shift the mean, making it a poor representation of the central tendency of the data. The median, being less sensitive to extreme values, is often a more robust measure of central tendency in the presence of outliers.
2. Inflated Variance and Standard Deviation: Amplified Spread
Outliers inflate the variance and standard deviation, leading to overestimation of the data's variability. This can affect the results of hypothesis tests and other statistical analyses that rely on these measures.
3. Skewed Distributions: Distorted Shapes
Outliers can distort the shape of a distribution, making it skewed. This can complicate the interpretation of the data and affect the choice of appropriate statistical methods.
4. Misleading Conclusions: Erroneous Interpretations
The presence of outliers can lead to misleading conclusions if not properly addressed. A seemingly significant relationship between variables might be solely due to the influence of a few outliers.
Handling Outliers: Strategies and Considerations
Once outliers have been identified, the question arises of how to handle them. There is no single "correct" approach. The best strategy depends on the context, the reason for the outlier, and the goals of the analysis.
1. Investigate the Cause: Understanding the Root Problem
Before making any decisions about outliers, it's crucial to investigate the cause of their existence. Are they due to measurement errors, data entry mistakes, or genuine extreme values? Understanding the origin of outliers can help inform the most appropriate approach.
2. Data Cleaning: Addressing Errors
If outliers are due to errors (e.g., data entry mistakes, equipment malfunction), they should be corrected or removed. This involves carefully reviewing the data and making appropriate adjustments. Documenting all data cleaning steps is essential for maintaining data integrity and transparency.
3. Transformation: Changing the Scale
Transforming the data, such as using logarithmic or square root transformations, can sometimes reduce the influence of outliers. This approach changes the scale of the data, making outliers less extreme.
4. Robust Statistical Methods: Choosing Appropriate Techniques
Employing robust statistical methods, which are less sensitive to outliers, is another strategy. For instance, using the median instead of the mean, or robust regression techniques, minimizes the impact of outliers on the analysis.
5. Winzorizing or Trimming: Data Modification
Winzorizing replaces outliers with less extreme values (e.g., the values at a specified percentile). Trimming involves removing a fixed percentage of the most extreme values from each end of the data.
6. Reporting and Transparency: Openly Addressing Outliers
Even if outliers are removed or transformed, it's crucial to report their existence and how they were handled in the analysis. Transparency is essential for maintaining the credibility and reproducibility of the research.
Outliers: Not Always a Problem
It's crucial to remember that outliers are not always problematic. Sometimes, they represent genuine extreme values that contain valuable information. For example, in fraud detection, outliers might indicate fraudulent activities. In scientific research, outliers could represent groundbreaking discoveries. Therefore, dismissing outliers without proper investigation can lead to the loss of potentially important insights.
Conclusion: A Balanced Approach
The handling of outliers requires a careful and balanced approach. It's not a matter of simply removing them; rather, it's a process of investigating their origins, understanding their potential impact, and choosing the most appropriate method for dealing with them based on the specific context. By combining visual inspection, statistical methods, and careful consideration of the underlying data, researchers can effectively manage outliers and draw reliable conclusions from their data. Always remember to document your methods and justify your decisions, ensuring transparency and reproducibility in your analysis. The key is not to eliminate outliers indiscriminately but to understand their implications and make informed choices that enhance the accuracy and integrity of your findings.
Latest Posts
Latest Posts
-
Mauricio Has A Circus Act That Involves Monkeys On Unicycles
May 11, 2025
-
The Diagnostic Term Dental Caries Literally Means
May 11, 2025
-
Artistic Form Usually Implies A Strong Degree Of Perceptible
May 11, 2025
-
Barbara Is Tiling Her Craft Room
May 11, 2025
-
Repaso De En Nuestra Casa Quiz
May 11, 2025
Related Post
Thank you for visiting our website which covers about Which Of The Following Is True About Outliers . We hope the information provided has been useful to you. Feel free to contact us if you have any questions or need further assistance. See you next time and don't miss to bookmark.