Which Of The Following Statements About Forecasting Is True
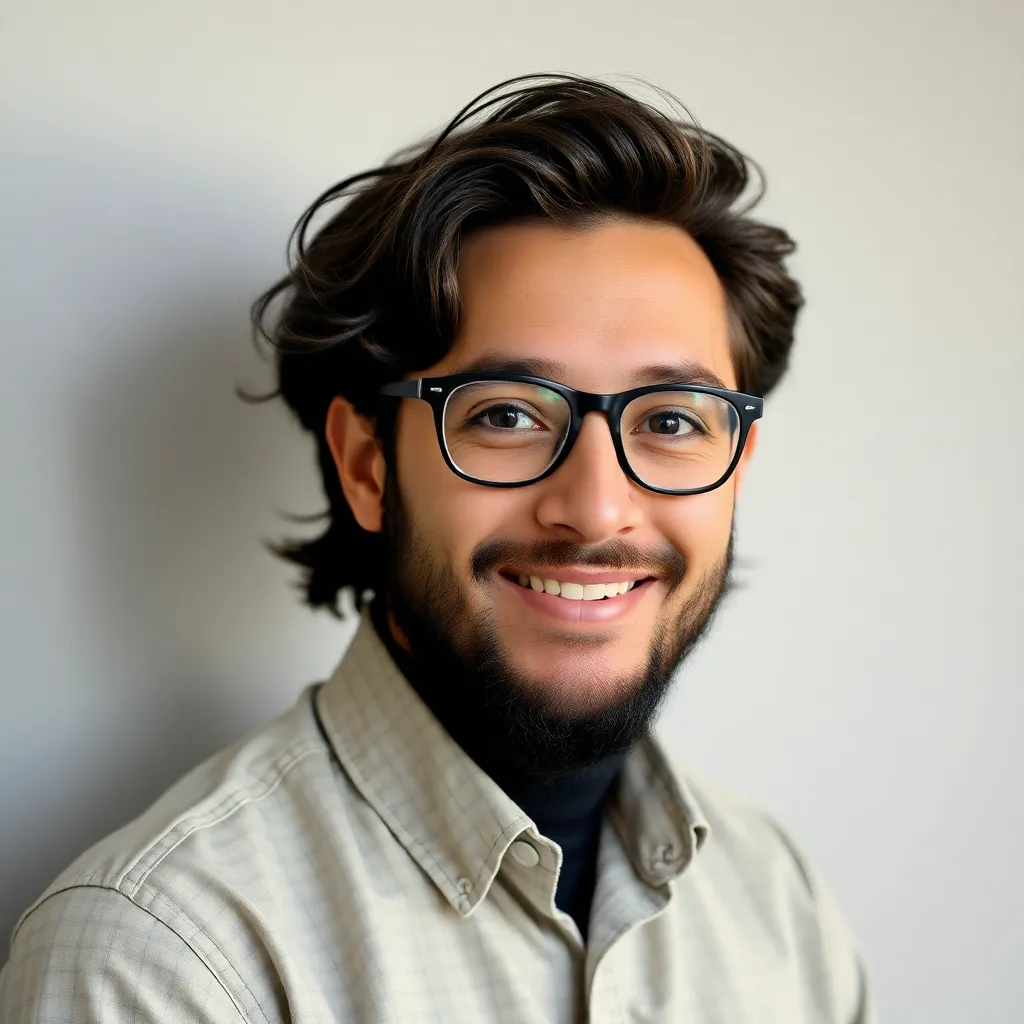
Onlines
May 11, 2025 · 6 min read

Table of Contents
Which of the following statements about forecasting is true? A Deep Dive into Forecasting Accuracy and Methodology
Forecasting, the art and science of predicting future outcomes, is crucial across diverse fields. From predicting stock prices to estimating future sales, accurate forecasting significantly impacts decision-making. But which statements about forecasting hold true? Let's delve into the nuances of forecasting accuracy and the methodologies employed, examining common misconceptions and highlighting key principles.
Understanding the Nature of Forecasting: It's Not Crystal Ball Gazing
Before tackling specific statements, it's vital to grasp the fundamental nature of forecasting. It's not about predicting the future with absolute certainty; rather, it's about making informed estimations based on available data and employing appropriate statistical techniques. Uncertainty is inherent in forecasting. The further into the future we project, the greater the uncertainty becomes. This is why short-term forecasts are generally more accurate than long-term forecasts.
Evaluating Forecasting Statements: A Critical Approach
Let's analyze common statements regarding forecasting and determine their validity:
Statement 1: All forecasting methods are equally accurate.
FALSE. This is a significant misconception. The accuracy of a forecasting method depends heavily on several factors:
- The nature of the data: Time series data exhibiting clear trends and seasonality might be well-suited to exponential smoothing or ARIMA models. Data with complex patterns might require more advanced techniques like machine learning algorithms.
- The forecasting horizon: Short-term forecasts are generally more accurate than long-term forecasts. Long-term forecasts are often subject to greater uncertainty due to unforeseen events and changes in underlying conditions.
- Data quality: Inaccurate or incomplete data will lead to inaccurate forecasts, regardless of the sophistication of the method used. Data cleaning and preprocessing are crucial steps in any forecasting process.
- Model selection: The choice of forecasting method is critical. A poorly chosen method will yield poor results, even with high-quality data. Consider the characteristics of your data and select a suitable model. Experimentation with multiple models is often beneficial.
Statement 2: Qualitative forecasting methods are always inferior to quantitative methods.
FALSE. While quantitative methods (those relying on numerical data and statistical techniques) are often preferred for their objectivity, qualitative methods have their strengths. Qualitative methods, such as expert opinions, Delphi techniques, and market research, are valuable when historical data is scarce, unavailable, or unreliable. They can provide insights that quantitative methods might miss. For example, in forecasting the impact of a new technology or a major regulatory change, qualitative methods are often indispensable. The best approach often involves combining both quantitative and qualitative methods for a more comprehensive forecast. This is known as hybrid forecasting.
Statement 3: A complex forecasting model is always better than a simple one.
FALSE. This is another crucial point. While complex models might offer greater potential accuracy, they also come with increased complexity, computational demands, and the risk of overfitting. Overfitting occurs when a model fits the historical data too well but performs poorly on new data. Occam's Razor applies here: The simplest model that adequately explains the data is often the best. Start with simpler models and only increase complexity if necessary. Evaluate the model's performance using appropriate metrics (e.g., Mean Absolute Error (MAE), Root Mean Squared Error (RMSE), Mean Absolute Percentage Error (MAPE)) and consider the trade-off between accuracy and complexity.
Statement 4: Forecasting accuracy is the only important factor.
FALSE. While accuracy is undeniably important, it's not the sole criterion for evaluating a forecasting method. Other factors include:
- Cost: Complex models can be computationally expensive and require specialized expertise.
- Timeliness: Some forecasting methods might require substantial time for computation, potentially rendering the forecast obsolete by the time it's available. Real-time forecasting is crucial in some applications.
- Understandability: A model that is too complex might be difficult to interpret and explain to stakeholders. Transparency and explainability are crucial, especially when making critical business decisions.
- Adaptability: The ability of a model to adapt to changing conditions and incorporate new data is essential for long-term forecasting.
Statement 5: Forecasting is a one-time process.
FALSE. Effective forecasting is an iterative process. Forecasts should be regularly reviewed and updated as new data becomes available. Monitoring forecast errors and making adjustments are vital for maintaining accuracy. The feedback loop between forecast generation, actual results, and model refinement is crucial for continuous improvement.
Key Forecasting Methodologies: A Brief Overview
Several widely used forecasting methodologies exist, each with its strengths and weaknesses:
1. Moving Average: This simple method averages data over a specific period. It's useful for smoothing out short-term fluctuations but can lag behind significant trends.
2. Exponential Smoothing: This technique assigns exponentially decreasing weights to older data, giving more importance to recent observations. Variations include single, double, and triple exponential smoothing, addressing different levels of trend and seasonality.
3. ARIMA (Autoregressive Integrated Moving Average): ARIMA models are powerful statistical techniques that capture autocorrelations in time series data. They can handle trends and seasonality effectively. However, they can be complex to implement and require careful parameter tuning.
4. Regression Analysis: Regression models use independent variables to predict a dependent variable. They are useful when there's a clear relationship between the variable being forecast and other factors.
5. Machine Learning Algorithms: Advanced machine learning algorithms, such as neural networks, support vector machines, and random forests, can handle complex patterns and large datasets. They often offer high accuracy but require substantial computational resources and expertise.
Improving Forecasting Accuracy: Best Practices
Several strategies can enhance forecasting accuracy:
- Data quality: Ensure data accuracy, completeness, and consistency. Address missing values and outliers appropriately.
- Feature engineering: Carefully select and transform variables to improve model performance.
- Model selection: Choose a suitable model based on data characteristics and forecasting horizon.
- Model evaluation: Use appropriate metrics to assess model accuracy and identify areas for improvement.
- Regular monitoring and updating: Continuously monitor forecast errors and update the model as new data becomes available.
- Collaboration and expertise: Involve experts from relevant fields to incorporate qualitative insights and domain knowledge.
Conclusion: Forecasting as an Ongoing Process
Accurate forecasting is not a simple task. It's an ongoing iterative process that demands a thorough understanding of the data, appropriate model selection, and continuous monitoring. Dismissing the importance of any aspect – data quality, model selection, or iterative refinement – can drastically reduce the effectiveness and accuracy of forecasting efforts. While absolute certainty is unattainable, using sound methodology and continuously refining techniques can significantly improve the reliability of predictions, empowering better decision-making in a wide range of applications. Understanding the limitations of forecasting and embracing a holistic approach, integrating quantitative and qualitative insights, is paramount to success.
Latest Posts
Related Post
Thank you for visiting our website which covers about Which Of The Following Statements About Forecasting Is True . We hope the information provided has been useful to you. Feel free to contact us if you have any questions or need further assistance. See you next time and don't miss to bookmark.