Which Of These Are Examples Of Business Analytics
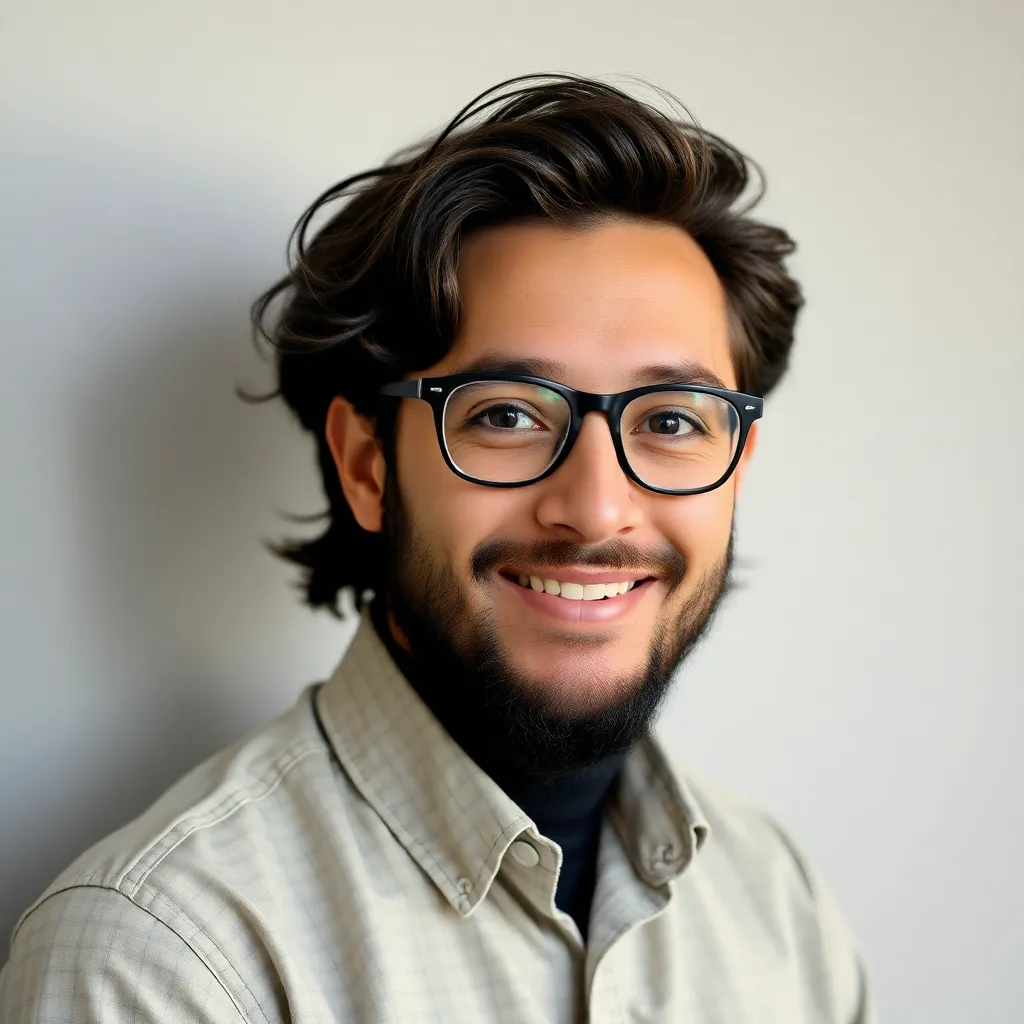
Onlines
Apr 04, 2025 · 6 min read

Table of Contents
Which of These Are Examples of Business Analytics? A Comprehensive Guide
Business analytics is transforming how companies operate, make decisions, and gain a competitive edge. But understanding what truly constitutes business analytics can be challenging. This comprehensive guide will delve into various examples, clarifying the nuances and demonstrating how different techniques contribute to effective business analytics. We'll explore the core components and highlight the key differences between descriptive, diagnostic, predictive, and prescriptive analytics. By the end, you'll be equipped to identify and understand the multifaceted applications of business analytics within a variety of industries.
Defining Business Analytics
Before diving into examples, let's establish a solid definition. Business analytics is the process of using data analysis, statistical methods, and other quantitative techniques to gain insights from raw data. These insights inform strategic and operational decision-making within an organization. It's about uncovering patterns, trends, and anomalies to improve efficiency, profitability, and overall business performance. It's not just about crunching numbers; it's about translating those numbers into actionable intelligence.
Core Components of Business Analytics
Effective business analytics relies on several key components working in harmony:
1. Data Collection:
This is the foundational step. It involves gathering data from various sources, including internal systems (CRM, ERP, marketing automation), external databases, social media, and market research. The quality and completeness of data are paramount.
2. Data Cleaning and Preparation:
Raw data is rarely ready for analysis. This stage involves cleaning, transforming, and preparing the data to ensure accuracy and consistency. This may include handling missing values, removing duplicates, and standardizing formats.
3. Data Analysis:
This is where the real magic happens. A variety of techniques are used, including statistical modeling, machine learning algorithms, data visualization, and data mining to analyze the prepared data and extract meaningful insights.
4. Interpretation and Visualization:
The insights gained from data analysis need to be communicated effectively. Data visualization tools are crucial for transforming complex data into easily understandable charts, graphs, and dashboards. Clear interpretation and communication are vital for informing decision-making.
5. Actionable Insights and Decision-Making:
The ultimate goal is to translate insights into actionable strategies. The analyses should guide decisions that improve business processes, optimize resource allocation, and drive revenue growth.
Types of Business Analytics: Examples Galore
Business analytics is often categorized into four main types:
1. Descriptive Analytics: What Happened?
Descriptive analytics focuses on summarizing historical data to understand past performance. It answers the "what happened?" question. Examples:
- Sales reports: Analyzing past sales data to identify top-selling products, geographic regions with high sales, and seasonal trends.
- Website analytics: Tracking website traffic, bounce rates, conversion rates, and user behavior to understand website performance and identify areas for improvement.
- Customer segmentation: Grouping customers based on demographic, behavioral, and purchase data to tailor marketing efforts.
- Financial statements: Analyzing income statements, balance sheets, and cash flow statements to track financial performance and identify trends.
- Operational dashboards: Displaying key performance indicators (KPIs) such as production output, customer satisfaction scores, and inventory levels in real-time.
Key Techniques: Data aggregation, summarization, data visualization (charts, graphs, dashboards).
2. Diagnostic Analytics: Why Did It Happen?
Diagnostic analytics goes beyond simply describing past events; it digs deeper to understand the underlying reasons. It answers the "why did it happen?" question. Examples:
- Root cause analysis: Investigating the reasons behind declining sales or increased customer churn. This might involve analyzing customer feedback, sales data, and market trends.
- Drill-down analysis: Examining sales data in detail to identify specific products, regions, or customer segments contributing to poor performance.
- Data mining: Uncovering hidden patterns and relationships in large datasets to identify potential causes of unexpected events.
- Exception reporting: Identifying unusual or unexpected events that deviate from established norms or patterns. For instance, sudden spikes or drops in website traffic or sales figures.
- Customer journey mapping: Visualizing the steps a customer takes during their interaction with the business to identify pain points and areas for improvement.
Key Techniques: Data mining, drill-down analysis, correlation analysis, data visualization.
3. Predictive Analytics: What Will Happen?
Predictive analytics uses historical data and statistical modeling to forecast future outcomes. It answers the "what will happen?" question. Examples:
- Sales forecasting: Predicting future sales based on historical sales data, economic indicators, and marketing campaigns.
- Customer churn prediction: Identifying customers at high risk of churning to implement retention strategies.
- Fraud detection: Predicting fraudulent transactions based on patterns and anomalies in transaction data.
- Inventory management: Predicting future demand to optimize inventory levels and minimize storage costs.
- Risk assessment: Assessing the likelihood of various risks, such as credit risk or operational risk.
- Demand forecasting: Anticipating customer demand for a product or service to optimize production and supply chain management.
Key Techniques: Regression analysis, time series analysis, machine learning algorithms (decision trees, random forests, neural networks).
4. Prescriptive Analytics: What Should We Do?
Prescriptive analytics goes beyond prediction; it recommends actions to optimize outcomes. It answers the "what should we do?" question. Examples:
- Optimization of pricing strategies: Determining the optimal pricing strategy to maximize revenue and profit, considering factors such as competitor pricing, demand elasticity, and cost structure.
- Supply chain optimization: Optimizing the supply chain to minimize costs and delivery times while meeting customer demand.
- Personalized recommendations: Recommending products or services to customers based on their individual preferences and past behavior. This could leverage techniques like collaborative filtering or content-based filtering.
- Resource allocation: Optimizing the allocation of resources, such as budget, personnel, and equipment, to maximize efficiency and effectiveness.
- Dynamic pricing: Adjusting prices in real-time based on factors such as demand, competition, and inventory levels.
Key Techniques: Optimization algorithms, simulation, decision support systems, expert systems.
Examples Across Industries
Business analytics is applicable across a wide range of industries. Here are some examples:
- Retail: Analyzing sales data to understand customer preferences, optimize inventory management, and personalize marketing campaigns.
- Finance: Detecting fraudulent transactions, assessing credit risk, and managing investments.
- Healthcare: Improving patient outcomes, managing hospital resources, and predicting disease outbreaks.
- Manufacturing: Optimizing production processes, predicting equipment failures, and improving quality control.
- Marketing: Personalizing marketing messages, optimizing advertising spend, and measuring campaign effectiveness.
- Transportation: Optimizing routes, scheduling, and fleet management.
Challenges and Considerations
While the potential benefits of business analytics are significant, several challenges need to be addressed:
- Data quality: Inaccurate or incomplete data can lead to flawed insights and poor decisions.
- Data security and privacy: Protecting sensitive data is crucial, especially in regulated industries.
- Data integration: Combining data from different sources can be complex and time-consuming.
- Skill gap: A shortage of skilled data analysts and data scientists can hinder the effective implementation of business analytics.
- Cost: Investing in the necessary technology and expertise can be expensive.
Conclusion
Business analytics encompasses a broad range of techniques and applications. By understanding the different types of business analytics – descriptive, diagnostic, predictive, and prescriptive – and their respective examples, organizations can harness the power of data to drive informed decision-making, improve operational efficiency, and gain a competitive advantage in today's data-driven world. Remember that success depends on the quality of data, skilled analysts, and a clear understanding of the business objectives. The examples provided offer a starting point for exploring the limitless possibilities of using business analytics to unlock business value.
Latest Posts
Latest Posts
-
True Or False The Painting Above Is By Diego Rivera
Apr 12, 2025
-
Match The Description To The Correct Vlan Type
Apr 12, 2025
-
With Respect To Cultural Differences In Supervision
Apr 12, 2025
-
Things Fall Apart Summary Chapter 14
Apr 12, 2025
-
Coral Reefs 2 Gizmo Answer Key
Apr 12, 2025
Related Post
Thank you for visiting our website which covers about Which Of These Are Examples Of Business Analytics . We hope the information provided has been useful to you. Feel free to contact us if you have any questions or need further assistance. See you next time and don't miss to bookmark.