Which Statement About Big Data Is False
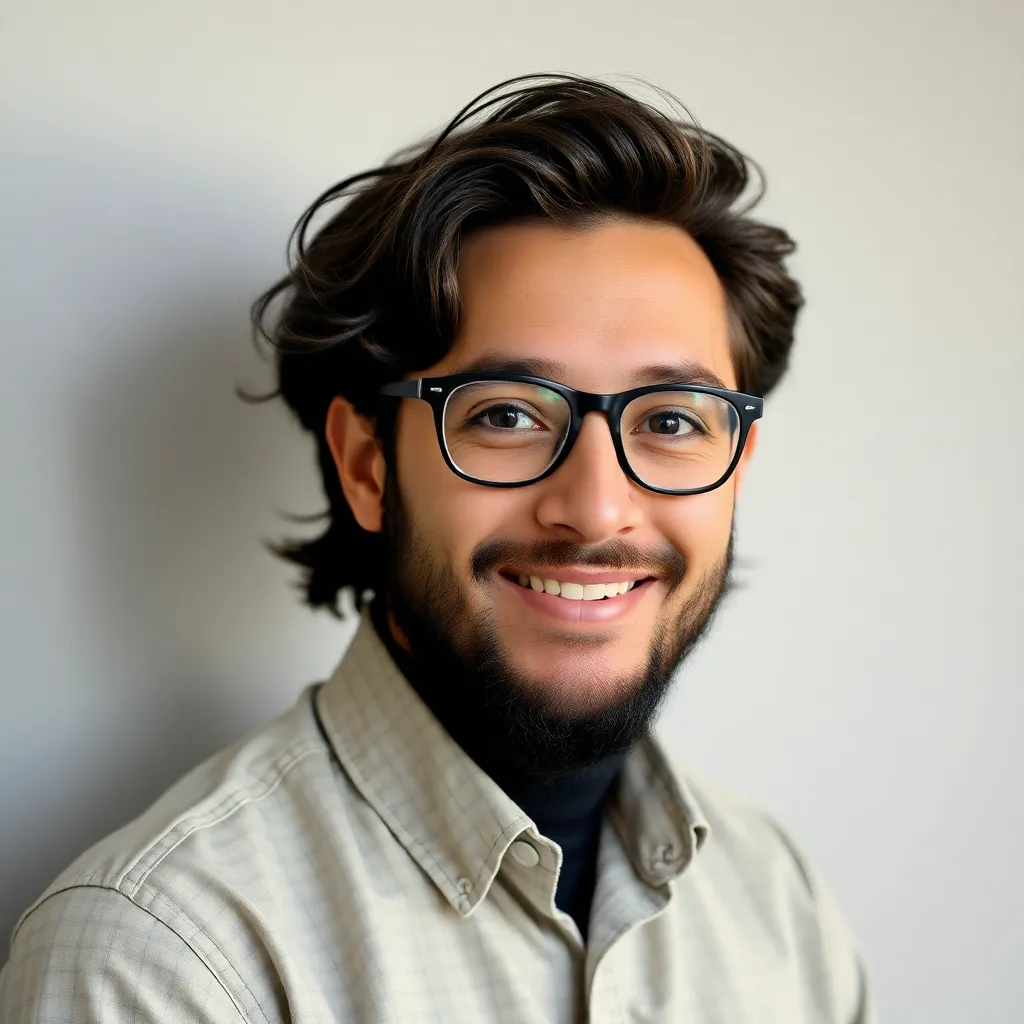
Onlines
May 04, 2025 · 7 min read

Table of Contents
Which Statement About Big Data is False? Debunking Common Myths
Big data. The term itself conjures images of massive datasets, powerful analytics, and transformative insights. But the hype surrounding big data often overshadows the nuances and complexities of actually working with it. Many misunderstandings and misconceptions persist, leading to inaccurate statements and flawed strategies. This article aims to clarify some of these misconceptions by identifying and debunking false statements about big data. We'll explore the reality behind the rhetoric and provide a clearer understanding of what big data truly entails.
Myth 1: Bigger is Always Better: The Falsehood of Sheer Volume
A common misconception is that the sheer volume of data is the defining characteristic of big data and the key to success. While volume is certainly a characteristic (one of the four Vs: Volume, Velocity, Variety, and Veracity), it's not the only one, nor is it automatically indicative of value. The statement "More data always equals better insights" is FALSE.
The Reality: Quality Over Quantity
The focus should be on data quality and relevance. Having terabytes of irrelevant or inaccurate data is not only useless but can actively hinder analysis and lead to flawed conclusions. Effective big data strategies prioritize data cleansing, validation, and the selection of relevant datasets. A smaller, well-curated dataset can often yield far more valuable insights than a massive, unrefined one. The key is to focus on the information that directly addresses specific business questions and objectives. This involves meticulous data governance and a clear understanding of the problem you're trying to solve. Investing time and resources in data quality control is paramount, far exceeding the importance of simply accumulating more raw data.
Myth 2: Big Data Requires Specialized, Expensive Technology: The Falsehood of Infrastructure Costs
Another common misconception is that processing big data necessitates expensive, specialized hardware and software solutions beyond the reach of most organizations. The statement "Big data analytics requires massive capital investment in cutting-edge technology" is FALSE.
The Reality: Cloud Computing and Scalability
The rise of cloud computing has dramatically democratized big data analytics. Cloud platforms offer scalable, cost-effective solutions that allow organizations of all sizes to leverage big data technologies without the burden of significant upfront infrastructure investments. Furthermore, open-source tools and frameworks are readily available, reducing reliance on proprietary and expensive software. While sophisticated solutions exist for extremely large datasets, many organizations can effectively analyze their data using readily available, relatively affordable cloud-based services. The choice of technology should be driven by specific needs and scale, not by the perceived necessity of top-of-the-line, high-cost hardware. A phased approach, starting with smaller-scale solutions and scaling up as needed, is often the most practical and financially sound strategy.
Myth 3: Big Data Solves All Problems: The Falsehood of Universal Applicability
Many believe that big data is a silver bullet solution for all business challenges. The statement "Big data automatically provides answers to all business questions" is FALSE.
The Reality: Context and Strategic Alignment
Big data is a powerful tool, but it's not a magical solution. Its effectiveness depends heavily on the clarity of the problem being addressed, the quality of the data, and the expertise of the analysts interpreting the results. Big data doesn't inherently provide answers; it provides the potential to discover answers. Successful application requires a clear understanding of the business problem, the selection of relevant data sources, and the application of appropriate analytical techniques. Poorly defined objectives, inadequate data preparation, and a lack of analytical expertise can easily lead to meaningless results. A well-defined strategy, aligned with specific business goals, is critical for leveraging the true potential of big data. The data itself needs to be interpreted within a broader business context to extract meaningful and actionable insights.
Myth 4: Big Data is Only for Large Corporations: The Falsehood of Size Restrictions
It's often assumed that big data analytics is only relevant to large, multinational corporations with extensive resources. The statement "Big data analytics is only feasible for large enterprises with significant budgets" is FALSE.
The Reality: Small and Medium-Sized Enterprises (SMEs) and Big Data
The accessibility of cloud-based solutions and open-source tools has opened up the world of big data analytics to SMEs. Smaller businesses can leverage readily available platforms and cost-effective solutions to gain valuable insights from their data. The key is to focus on specific, measurable business objectives and to leverage the data that is most readily available and relevant to those objectives. While the scale of data may be smaller than in large corporations, the potential for insightful analysis remains significant, especially in areas like customer relationship management, marketing optimization, and operational efficiency. Start small, focus on immediate needs, and gradually scale up as the value of big data analytics becomes evident.
Myth 5: Big Data Guarantees Improved Decision Making: The Falsehood of Automated Insight
A common belief is that deploying big data analytics automatically translates to better decision-making. The statement "Big data analytics always leads to better business decisions" is FALSE.
The Reality: Human Interpretation and Context
Big data provides data-driven insights, but it doesn't make decisions on its own. The interpretation of results requires human expertise, critical thinking, and consideration of factors beyond the scope of the data. Human judgment is crucial in validating findings, contextualizing results, and integrating them into broader strategic decision-making processes. Biases in data collection, analytical limitations, and unforeseen external factors can all influence the accuracy and applicability of the insights derived from big data. Effective implementation requires a combination of technical expertise and strong business acumen to ensure that the insights generated are both accurate and actionable. Blind faith in data without human oversight can lead to flawed decisions.
Myth 6: Big Data is Primarily About Technology: The Falsehood of a Sole Focus on Technology
Many discussions about big data center solely on the technical aspects—the hardware, software, and algorithms. The statement "Big data is primarily a technological challenge" is FALSE.
The Reality: People, Processes, and Governance
Successfully implementing big data analytics requires a holistic approach that encompasses people, processes, and governance, in addition to technology. This includes:
- Data Governance: Establishing clear policies and procedures for data collection, storage, and use.
- Data Security: Implementing robust measures to protect sensitive data from unauthorized access.
- Data Quality: Prioritizing data cleansing, validation, and ensuring accuracy.
- Skills Development: Training employees to effectively utilize big data tools and interpret the results.
- Change Management: Adapting organizational processes and workflows to integrate big data insights.
Investing in these non-technical aspects is just as critical as investing in technology. Without a robust framework for data governance, security, and interpretation, the technical capabilities of big data are significantly diminished. Ultimately, the success of any big data initiative hinges on the people and processes that support it.
Myth 7: Big Data is Only About Predicting the Future: The Falsehood of Predictive Power Only
A prevalent misconception is that the primary purpose of big data is to predict future outcomes. The statement "Big data is solely focused on predictive analytics" is FALSE.
The Reality: Descriptive, Diagnostic, and Prescriptive Analytics
While predictive analytics is a valuable application of big data, it's not the only one. Big data also enables:
- Descriptive Analytics: Summarizing historical data to understand past trends and patterns.
- Diagnostic Analytics: Identifying the root causes of past events or outcomes.
- Prescriptive Analytics: Recommending actions to optimize future outcomes.
A comprehensive big data strategy utilizes all these types of analytics, not just predictive modeling. Understanding past performance, diagnosing underlying issues, and then using predictive modeling to forecast future trends provides a more complete and nuanced picture. The focus should be on utilizing the full spectrum of analytical capabilities to gain a holistic understanding of the business landscape.
Conclusion: Navigating the Big Data Landscape
Understanding the realities behind common misconceptions surrounding big data is crucial for successful implementation. By dispelling these myths and embracing a data-driven approach grounded in clear objectives, quality data, and a holistic strategy, organizations of all sizes can unlock the transformative potential of big data and gain a competitive edge. Remember that big data is a powerful tool, but its effectiveness relies on careful planning, robust execution, and a clear understanding of its limitations. It's not a magic bullet, but a potent resource when harnessed correctly.
Latest Posts
Latest Posts
-
6 Variables That Affect Fuel As It Relates To Fire
May 04, 2025
-
A Model For Circuits Part 1 Current And Resistance
May 04, 2025
-
History And Trends Of Healthcare Chapter 1
May 04, 2025
-
Explain The Key Issue Dividing Mugwumps Halfbreeds
May 04, 2025
-
Their Eyes Were Watching God Chapter 17 Summary
May 04, 2025
Related Post
Thank you for visiting our website which covers about Which Statement About Big Data Is False . We hope the information provided has been useful to you. Feel free to contact us if you have any questions or need further assistance. See you next time and don't miss to bookmark.