Which Statement Is Not True About Processing
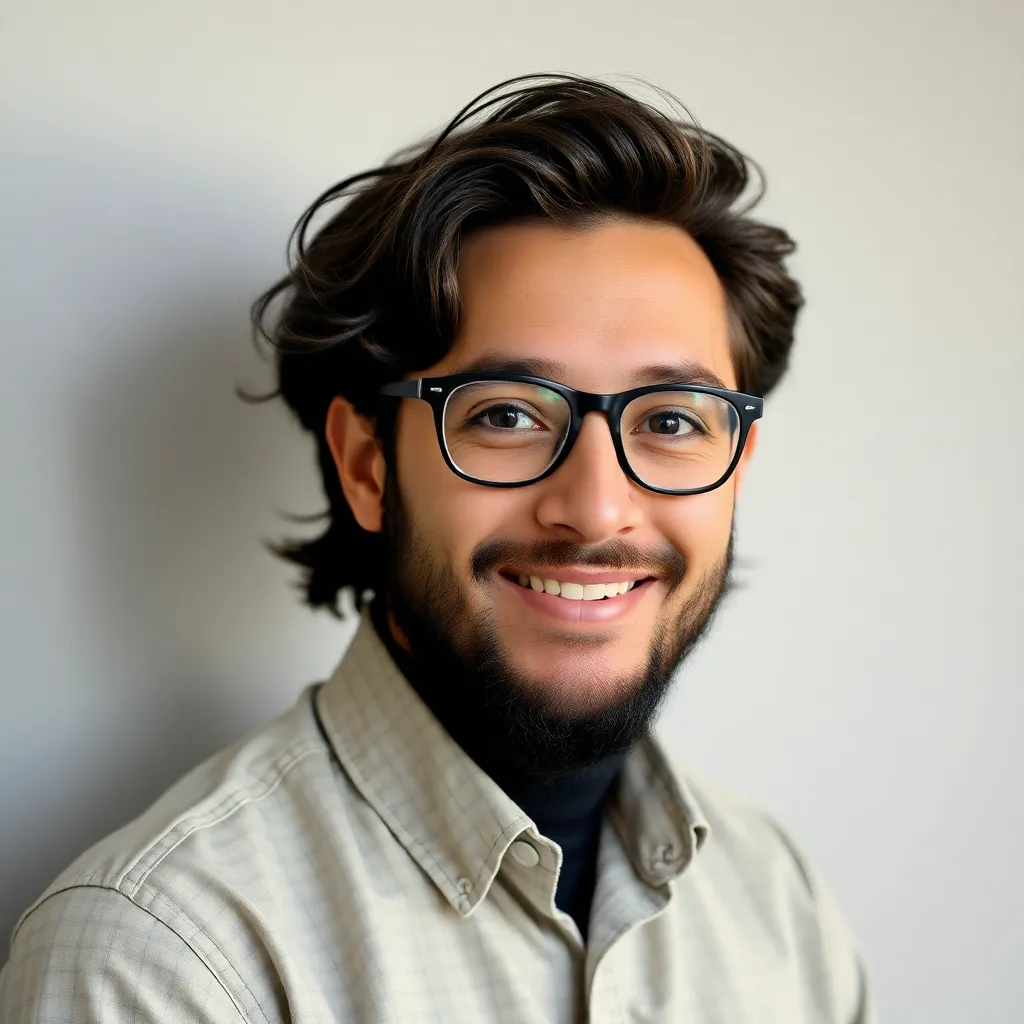
Onlines
Apr 13, 2025 · 6 min read

Table of Contents
Which Statement is Not True About Processing? Deconstructing Common Misconceptions
Understanding data processing is crucial in today's digital world. From simple calculations to complex machine learning algorithms, processing forms the backbone of countless applications. However, many misconceptions surround the nature of data processing, leading to confusion and inefficient practices. This comprehensive article will delve into common statements about processing and identify the ones that are not true. We'll explore various aspects, including speed, accuracy, cost, and the role of human intervention, to provide a clearer picture of this vital technological domain.
Misconception 1: "Faster Processing Always Means Better Processing"
This statement is not true. While speed is a desirable attribute in processing, it's not the sole indicator of quality. A faster process might be achieved by sacrificing accuracy, reliability, or security. For instance, a high-speed algorithm that delivers inaccurate results is ultimately useless. Similarly, prioritizing speed could lead to neglecting data integrity checks, resulting in corrupted or unreliable data. Effective processing requires a balance between speed, accuracy, and robustness. A slower, more precise process might be far superior to a faster, error-prone one, especially in contexts like medical imaging analysis or financial transactions where accuracy is paramount. The optimal processing speed depends entirely on the specific application and its requirements. Some applications, like real-time gaming, demand high speed, while others, like scientific simulations, prioritize accuracy over raw speed.
Misconception 2: "Processing is Always a Completely Automated Process"
False. While many processing tasks are automated, human intervention often plays a crucial, sometimes indispensable, role. Data preprocessing, for instance, often involves human experts cleaning, validating, and labeling data. This manual intervention ensures data quality and removes biases or inconsistencies that automated systems might miss. Furthermore, interpreting the results of complex processing tasks, such as those performed by machine learning algorithms, frequently requires human expertise. Analysts need to understand the context of the data, validate the findings, and make informed decisions based on the processed information. Therefore, thinking of processing as solely an automated procedure ignores the significant contributions of human intelligence and expertise.
Misconception 3: "All Processing is Equally Expensive"
This is absolutely false. The cost of processing varies drastically depending on several factors:
- Data volume: Processing larger datasets naturally costs more, requiring greater computational resources and potentially longer processing times.
- Complexity of algorithms: Sophisticated algorithms, like deep learning models, demand far more computational power and energy than simpler algorithms. This translates directly into higher processing costs.
- Hardware infrastructure: The type of hardware used (cloud computing vs. on-premise servers, the type of processors, memory capacity) significantly affects the cost. Cloud computing, while offering scalability, can become expensive for large-scale processing.
- Software licensing: The cost of specialized software and licenses for data processing tools can be substantial.
- Personnel costs: Salaries of data scientists, engineers, and other personnel involved in designing, implementing, and maintaining processing systems represent a major cost component.
Therefore, generalizing about the cost of processing is misleading. The actual expense can range from minimal (for simple tasks on a personal computer) to substantial (for large-scale, complex projects involving high-performance computing).
Misconception 4: "More Data Always Leads to Better Processing Results"
Incorrect. While more data can often improve the accuracy of certain machine learning models, it's not a guaranteed path to better results. "Garbage in, garbage out" remains a relevant principle. Adding noisy, irrelevant, or biased data can actually degrade the quality of processing outcomes. Effective processing hinges on data quality, not just quantity. A smaller, clean, well-structured dataset can yield better results than a massive dataset cluttered with errors and inconsistencies. Furthermore, excessive data can overwhelm processing systems, leading to longer processing times and higher costs without necessarily improving accuracy. Data curation and careful selection are crucial for optimizing processing results.
Misconception 5: "Processing is Only Relevant for Large Organizations"
This statement is not true. Data processing is relevant across all sectors and organizations, regardless of size. While large corporations may utilize more sophisticated and complex processing methods, even small businesses and individuals benefit from data processing techniques.
- Small businesses: Can leverage basic data processing to track sales, manage inventory, and analyze customer behavior. Simple spreadsheet software or cloud-based solutions provide accessible processing tools.
- Individuals: Use data processing implicitly and explicitly through various applications. From photo editing software (processing images) to fitness trackers (processing activity data), everyday life is filled with data processing applications.
Misconception 6: "Processing is a One-Time Activity"
False. In most cases, data processing is an iterative and ongoing process. Data is constantly generated and updated, requiring continuous processing to extract meaningful insights and make informed decisions. For example, a company analyzing customer behavior needs to process new data regularly to keep their insights current. Similarly, scientific research often involves repeated data processing as new experiments generate more data. Effective data management strategies should incorporate continuous processing cycles to ensure ongoing relevance and accuracy.
Misconception 7: "All Processing Techniques Are Interchangeable"
This is incorrect. Different processing techniques are suited for different types of data and tasks. The optimal method depends on factors like data structure, volume, desired outcome, and available resources. For instance, using a computationally intensive algorithm for a small dataset would be inefficient. Similarly, applying a simple algorithm to a large, complex dataset might not provide satisfactory results. Choosing the appropriate processing technique is crucial for achieving accurate and efficient results. Selecting the wrong method can lead to inaccurate outcomes, wasted resources, and missed opportunities.
Misconception 8: "Processing Guarantees Perfect Accuracy"
Untrue. No processing technique guarantees perfect accuracy. Errors can arise from various sources, including:
- Data errors: Inaccurate, incomplete, or inconsistent data can lead to erroneous results.
- Algorithm limitations: Algorithms may have inherent limitations or biases that affect accuracy.
- Hardware malfunctions: Hardware failures can corrupt data or produce inaccurate results.
- Human errors: Mistakes in data entry, algorithm design, or interpretation can introduce errors.
Therefore, it's crucial to understand and manage potential sources of error during the processing stages. Implementing quality control measures, validation checks, and error-handling mechanisms can minimize the impact of inaccuracies.
Misconception 9: "Processing is Only About Numbers"
Incorrect. While numerical data is a frequent target of processing, it extends far beyond numbers. Text, images, audio, video, and sensor data all require specific processing techniques. Natural Language Processing (NLP) analyzes text data, computer vision processes images and videos, and signal processing handles audio and sensor data. The scope of data processing encompasses a vast array of data types and modalities. The techniques and algorithms employed vary greatly depending on the nature of the data.
Misconception 10: "Understanding Processing Requires Advanced Technical Skills"
While advanced technical expertise is necessary for designing and developing sophisticated processing systems, a basic understanding of data processing principles is valuable for everyone. In today's data-driven world, individuals across various professions benefit from understanding how data is processed and interpreted. This knowledge enables better decision-making, more effective communication, and a deeper appreciation for the technology that shapes our lives. Data literacy is becoming increasingly important, and it doesn't require advanced technical skills to grasp the fundamental concepts.
Conclusion
This article has deconstructed several common misconceptions surrounding data processing. It's crucial to recognize that processing is not a monolithic entity but a diverse field encompassing various techniques, applications, and challenges. Understanding these nuances is essential for effectively leveraging the power of data processing across diverse domains, from individual users to large organizations. By dispelling these misconceptions, we can foster a more informed and accurate understanding of this fundamental technological process, paving the way for more effective and efficient data-driven solutions.
Latest Posts
Latest Posts
-
The Biggest Little Farm Questions And Answers
Apr 15, 2025
-
A Chronicle Of A Death Foretold Summary
Apr 15, 2025
-
Dna Evidence Evaluation Who Ate The Cheese Answers
Apr 15, 2025
-
The Ziggurat At Ur Can Best Be Described As A
Apr 15, 2025
-
At January 1 2024 Cafe Med Leased Restaurant Equipment
Apr 15, 2025
Related Post
Thank you for visiting our website which covers about Which Statement Is Not True About Processing . We hope the information provided has been useful to you. Feel free to contact us if you have any questions or need further assistance. See you next time and don't miss to bookmark.