Which Two Hypotheses Can Be Supported With Quantitative Data
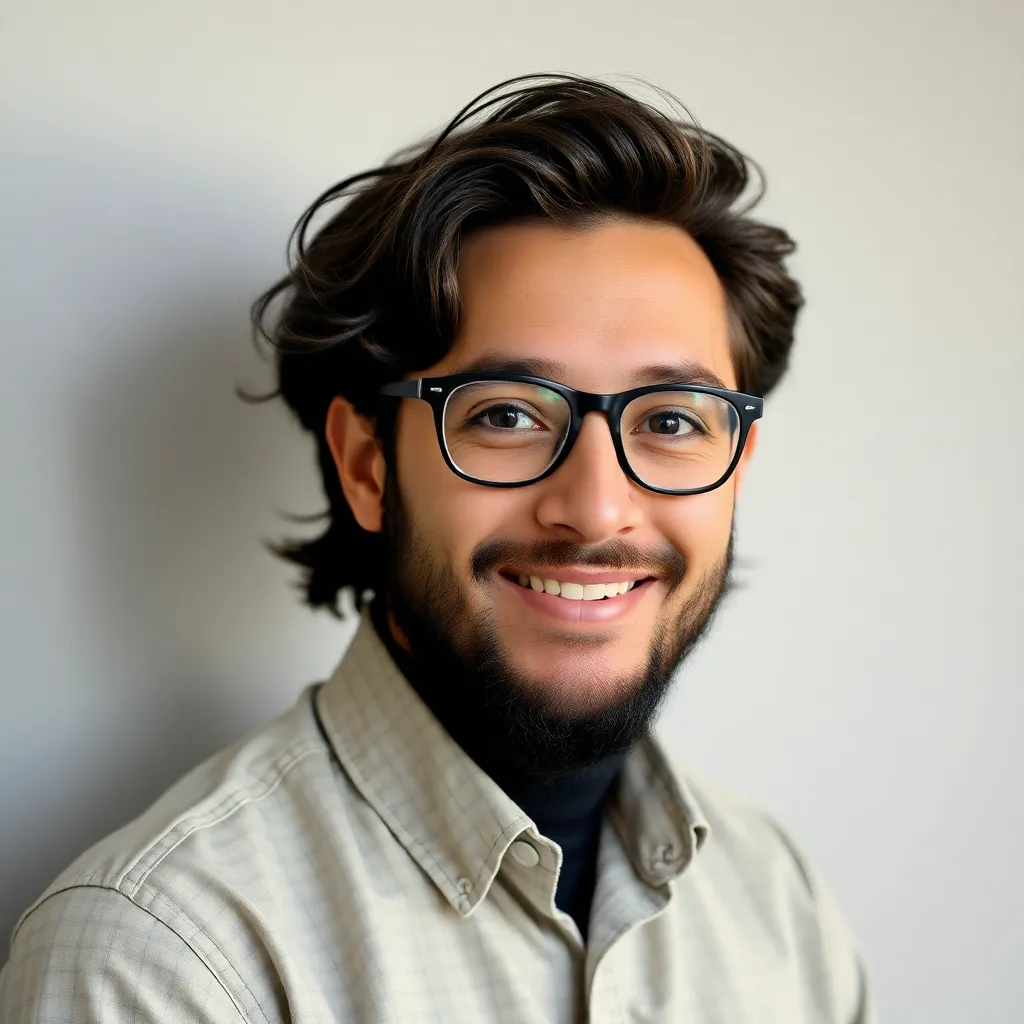
Onlines
May 10, 2025 · 6 min read
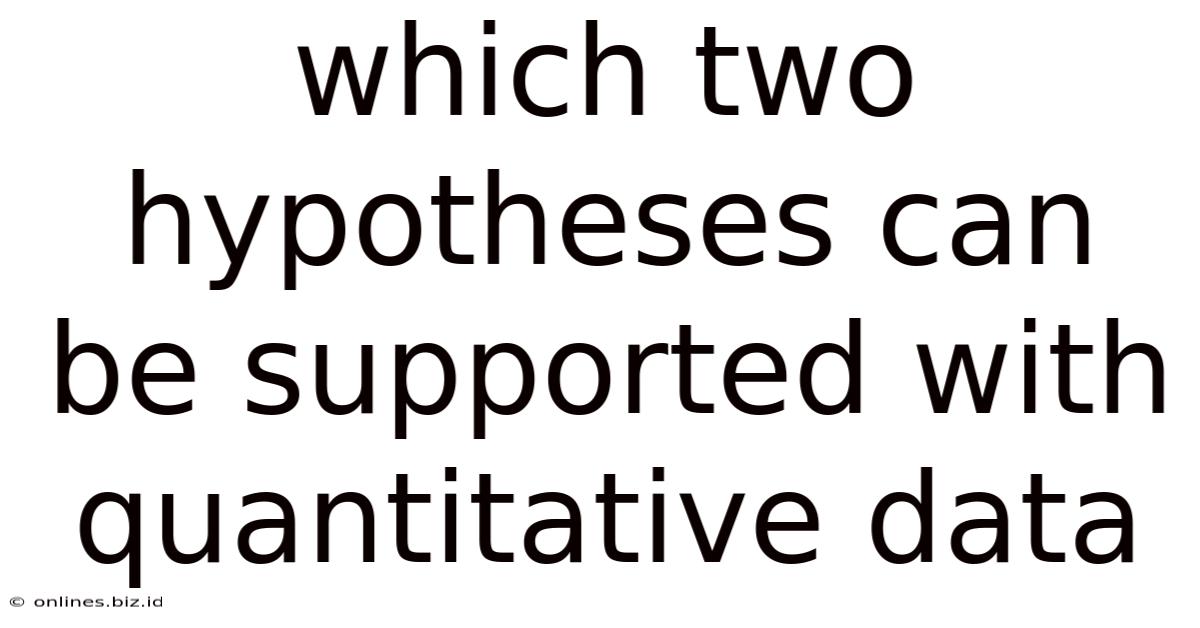
Table of Contents
Which Two Hypotheses Can Be Supported With Quantitative Data? A Deep Dive into Research Methodology
Quantitative research, characterized by its numerical data and statistical analysis, is a powerful tool for testing hypotheses and drawing robust conclusions. But not all hypotheses are suited to this approach. This article explores two types of hypotheses ideally suited for quantitative analysis, delving into their characteristics, the data collection methods used to support them, and the statistical techniques employed for analysis. We'll also examine the crucial role of appropriate research design in ensuring the validity and reliability of the findings.
Hypothesis 1: Examining the Relationship Between Two or More Variables
This type of hypothesis focuses on exploring the correlation or causation between variables. It often involves predicting the direction and strength of the relationship. These hypotheses are particularly well-suited to quantitative methods because they allow for the precise measurement and comparison of variables.
Characteristics of Relationship Hypotheses
- Measurable Variables: The variables involved must be clearly defined and measurable using quantitative metrics. This means they can be assigned numerical values, allowing for statistical analysis. Examples include age, income, test scores, or frequency of a behavior.
- Predictive Nature: The hypothesis proposes a relationship, either positive (as one variable increases, the other increases), negative (as one variable increases, the other decreases), or no relationship. The prediction is testable through statistical analysis.
- Testable with Statistical Tests: Appropriate statistical tests, such as correlation analysis, regression analysis, or ANOVA (Analysis of Variance), can be used to determine the strength and significance of the relationship.
Example: The Impact of Social Media Use on Self-Esteem
Hypothesis: Increased daily social media usage is negatively correlated with self-esteem among adolescents.
This hypothesis proposes a relationship between two measurable variables: daily social media usage (measured in minutes or hours) and self-esteem (measured using a standardized self-esteem scale). A quantitative study could collect data through surveys and questionnaires, analyzing the correlation between the two variables using statistical tests. A negative correlation would support the hypothesis, suggesting that higher social media usage is associated with lower self-esteem.
Data Collection Methods for Relationship Hypotheses
- Surveys: Surveys are efficient for gathering large amounts of data from a diverse sample. Questions should be structured to elicit numerical responses or scores.
- Experiments: Experiments allow for the manipulation of one variable (independent variable) to observe its effect on another (dependent variable). This approach allows for stronger conclusions about causation.
- Existing Datasets: Analyzing readily available quantitative datasets (e.g., census data, government statistics) can be a cost-effective method for testing hypotheses about relationships between variables.
Statistical Techniques for Analysis
- Correlation Analysis: Measures the strength and direction of the linear relationship between two variables.
- Regression Analysis: Predicts the value of a dependent variable based on the values of one or more independent variables.
- ANOVA: Compares the means of two or more groups to determine if there are statistically significant differences.
Hypothesis 2: Comparing Group Means or Proportions
This type of hypothesis focuses on comparing differences between two or more groups. It often involves testing whether there is a statistically significant difference in the means (average values) or proportions (percentage of individuals with a certain characteristic) of a variable across different groups.
Characteristics of Group Comparison Hypotheses
- Distinct Groups: The hypothesis involves comparing distinct groups based on a categorical variable (e.g., gender, age group, treatment condition).
- Measurable Variable: A continuous or categorical variable is measured for each group.
- Testable with Statistical Tests: Statistical tests, such as t-tests, ANOVA, or chi-square tests, are used to determine if the differences between group means or proportions are statistically significant.
Example: The Effectiveness of a New Teaching Method
Hypothesis: Students taught using the new teaching method will score significantly higher on a standardized test than students taught using the traditional method.
This hypothesis compares the average test scores of two groups: students exposed to a new teaching method and students taught using the traditional method. The data collected would be the test scores of students in each group. A t-test or ANOVA could be employed to determine if there's a statistically significant difference in the mean test scores between the two groups. A significant difference would support the hypothesis.
Data Collection Methods for Group Comparison Hypotheses
- Experiments: Randomly assigning participants to different groups (treatment and control) ensures that any observed differences are attributable to the manipulation of the independent variable (the teaching method).
- Observational Studies: Observing pre-existing groups and measuring the variable of interest. This approach is useful when manipulating the independent variable is not feasible or ethical.
- Secondary Data Analysis: Analyzing existing data collected by others, such as educational achievement data from a school district.
Statistical Techniques for Analysis
- t-test: Compares the means of two groups.
- ANOVA: Compares the means of three or more groups.
- Chi-square test: Compares proportions or frequencies of categorical data across groups.
The Importance of Research Design in Quantitative Research
The success of quantitative research hinges on a well-defined research design. This involves several crucial aspects:
- Sampling: The selection of participants must be representative of the population of interest to ensure generalizability of the findings. Random sampling techniques are preferred to minimize bias.
- Measurement: Reliable and valid instruments (e.g., standardized tests, questionnaires) must be used to measure the variables accurately. Validity refers to whether the instrument measures what it intends to measure, while reliability refers to the consistency of the measurement.
- Control of Confounding Variables: Researchers must identify and control for confounding variables – variables that could influence the relationship between the variables of interest, leading to misleading results. Random assignment in experiments helps control for confounding variables.
- Data Analysis Plan: A clear plan for data analysis should be established before data collection. This ensures that the appropriate statistical tests are selected and that the results are interpreted correctly.
Ensuring Validity and Reliability of Quantitative Research
Several strategies contribute to ensuring the validity and reliability of quantitative research:
- Pilot Testing: Conducting a pilot study with a small sample before the main study helps identify potential problems with the research design, data collection instruments, or analysis procedures.
- Triangulation: Employing multiple methods or data sources to measure the same variable can enhance the validity of the findings.
- Inter-rater Reliability: When multiple raters or observers are involved, inter-rater reliability checks assess the consistency of their measurements.
- Statistical Power Analysis: Determining the required sample size before the study begins ensures sufficient power to detect statistically significant effects.
Conclusion
Quantitative research offers a powerful approach for testing hypotheses related to relationships between variables and comparisons of group means or proportions. By carefully selecting appropriate hypotheses, employing rigorous research designs, and utilizing suitable statistical techniques, researchers can generate robust and meaningful insights that contribute to our understanding of the world. Remember, the key is to ensure that the chosen hypotheses are clearly stated, testable, and aligned with the capabilities of quantitative methodologies. By meticulously planning each stage, from hypothesis formulation to data analysis and interpretation, researchers can maximize the validity and reliability of their findings, generating impactful results with significant implications for their respective fields.
Latest Posts
Latest Posts
-
Which Of The Following Documents Must An Agent Submit
May 10, 2025
-
Career Tracking Resource And Advancement Compass
May 10, 2025
-
El Botones Lleva Unas Maletas A La Habitacion
May 10, 2025
-
Racism Tends To Intensify During Periods Of Economic Uncertainty
May 10, 2025
-
What Does Jefferson State Directly As The Reason
May 10, 2025
Related Post
Thank you for visiting our website which covers about Which Two Hypotheses Can Be Supported With Quantitative Data . We hope the information provided has been useful to you. Feel free to contact us if you have any questions or need further assistance. See you next time and don't miss to bookmark.