Worksheet A Topic 2.5 Exponential Data Modeling
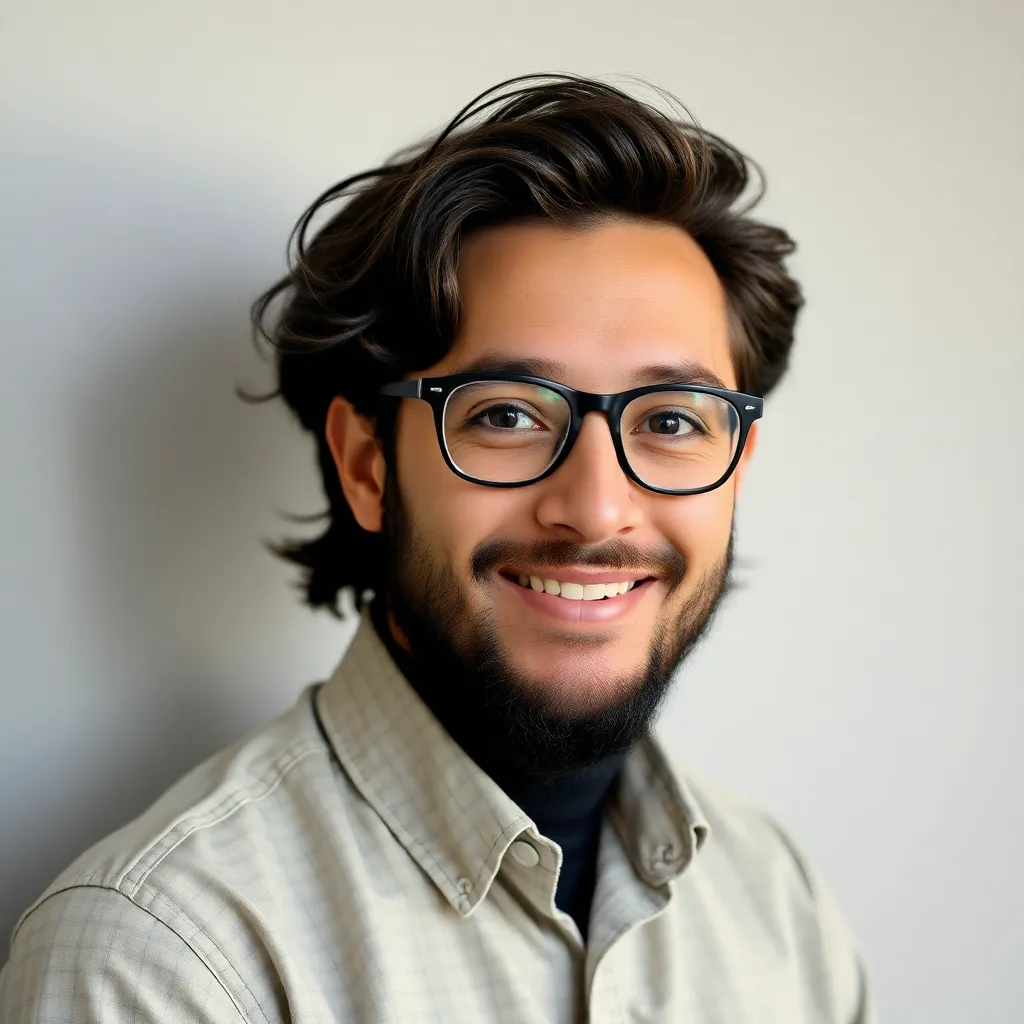
Onlines
Apr 08, 2025 · 5 min read

Table of Contents
Worksheet: Topic 2.5 Exponential Data Modeling
Introduction
Exponential data modeling is a powerful technique used to analyze and predict data exhibiting exponential growth or decay. This worksheet will guide you through the process of building and interpreting exponential models, focusing on practical applications and real-world scenarios. We'll explore various methods, including graphical analysis, linearization, and regression techniques, emphasizing the importance of understanding the underlying assumptions and limitations of each approach. This comprehensive guide will equip you with the skills to effectively analyze exponential data, make accurate predictions, and draw meaningful conclusions.
Understanding Exponential Growth and Decay
Before diving into modeling, it's crucial to grasp the fundamental concepts of exponential growth and decay.
Exponential Growth
Exponential growth describes a situation where a quantity increases at a rate proportional to its current value. The general formula is:
y = ab<sup>x</sup>
Where:
- y represents the final amount
- a represents the initial amount (when x = 0)
- b represents the growth factor (b > 1)
- x represents the time or independent variable
The larger the value of 'b', the faster the growth. Examples of exponential growth include population growth, compound interest, and the spread of viral content.
Exponential Decay
Conversely, exponential decay describes a situation where a quantity decreases at a rate proportional to its current value. The formula remains similar:
y = ab<sup>x</sup>
However, in this case, the growth factor 'b' is between 0 and 1 (0 < b < 1). A smaller 'b' indicates faster decay. Examples include radioactive decay, drug metabolism, and the depreciation of assets.
Methods for Exponential Data Modeling
Several methods can be employed to model exponential data. Let's explore the most common ones.
1. Graphical Analysis
A simple yet effective way to determine if your data exhibits exponential behavior is through visual inspection. Plotting the data on a scatter plot can reveal a characteristic curve indicative of exponential growth or decay. However, graphical analysis alone is rarely sufficient for precise modeling; it serves primarily as a preliminary assessment.
2. Linearization using Logarithms
This powerful technique transforms the exponential equation into a linear one, allowing the use of linear regression techniques. Taking the natural logarithm (ln) of both sides of the exponential equation:
ln(y) = ln(ab<sup>x</sup>) = ln(a) + x ln(b)
This equation is now in the form of a linear equation, Y = mX + c, where:
- Y = ln(y)
- X = x
- m = ln(b)
- c = ln(a)
By plotting ln(y) against x, you obtain a straight line. The slope (m) and y-intercept (c) can be determined using linear regression, and consequently, 'a' and 'b' can be calculated.
3. Regression Analysis (Nonlinear Least Squares)
For more precise modeling, especially when dealing with noisy data, nonlinear least squares regression is preferred. This method directly fits the exponential equation (y = ab<sup>x</sup>) to the data, minimizing the sum of squared errors between the observed and predicted values. Specialized software or statistical packages are typically required for this method.
4. Choosing the Right Model
The choice between linearization and nonlinear regression depends on several factors:
- Data quality: For noisy data or data with outliers, nonlinear regression is often more robust.
- Software availability: Linearization is simpler to implement if you only have basic spreadsheet software.
- Accuracy requirements: Nonlinear regression generally provides more accurate parameter estimates.
Interpreting the Model
Once an exponential model is established, interpreting its parameters and making predictions are crucial steps.
Understanding the Parameters (a and b)
-
a (Initial value): Represents the value of the dependent variable when the independent variable is zero. It's the starting point of the exponential growth or decay process.
-
b (Growth/Decay factor): Determines the rate of growth or decay. If b > 1, it indicates growth; if 0 < b < 1, it indicates decay. The magnitude of b reflects the speed of the process. A larger b (for growth) or a smaller b (for decay) signifies a faster rate.
Making Predictions
Once the values of 'a' and 'b' are known, you can use the exponential model (y = ab<sup>x</sup>) to predict future values of the dependent variable for given values of the independent variable. Remember that the accuracy of these predictions depends heavily on the quality of the data and the goodness of fit of the model.
Real-World Applications of Exponential Data Modeling
Exponential data modeling has broad applications across various fields:
1. Finance: Compound Interest
Compound interest calculations rely heavily on exponential growth models. Understanding this model helps investors predict future investment values and make informed financial decisions.
2. Biology: Population Growth
Modeling population growth of organisms, bacteria, or viruses often involves exponential models. Understanding these models is vital for ecological studies and public health management.
3. Medicine: Drug Metabolism
Pharmacokinetics, the study of drug absorption, distribution, metabolism, and excretion, often utilizes exponential decay models to describe drug clearance from the body.
4. Physics: Radioactive Decay
Radioactive decay follows an exponential decay pattern, allowing scientists to date ancient artifacts and understand nuclear processes.
5. Engineering: System Reliability
Exponential models are used to model the lifespan of systems, aiding in reliability analysis and predictive maintenance.
Limitations and Assumptions
While powerful, exponential models have limitations and underlying assumptions:
-
Constant growth/decay rate: The model assumes a constant rate of growth or decay over time. This may not hold true for real-world scenarios where the rate can fluctuate.
-
Data quality: Accurate and reliable data is crucial for building a meaningful model. Outliers and errors can significantly impact the results.
-
Model extrapolation: Extrapolating beyond the range of the data can be unreliable, as the model's assumptions may not hold outside this range.
Conclusion
Exponential data modeling is a valuable tool for analyzing and predicting data exhibiting exponential patterns. This worksheet has provided a comprehensive overview of the methods, interpretations, and applications of exponential models. Remember that careful consideration of the data, model assumptions, and limitations is crucial for drawing accurate conclusions and making reliable predictions. By understanding these factors, you can effectively leverage the power of exponential data modeling in various scientific, engineering, and financial contexts. Always remember to validate your model and consider potential limitations before making critical decisions based on your analysis. Further exploration into statistical software and advanced regression techniques can enhance your skills and allow you to handle more complex data sets and modeling scenarios. This foundation will enable you to tackle increasingly sophisticated data analysis tasks with confidence.
Latest Posts
Latest Posts
-
A Nurse Is Discussing The Norming Stage Of Group Development
Apr 17, 2025
-
A Blight Begins To Infect Yellow Pine Trees
Apr 17, 2025
-
Which Statement Best Describes The Procedure Of Response Blocking
Apr 17, 2025
-
6 2 8 Area Of A Square With Default Parameters
Apr 17, 2025
-
Beatriz E Ignacio Una Vez Durante La Fiesta
Apr 17, 2025
Related Post
Thank you for visiting our website which covers about Worksheet A Topic 2.5 Exponential Data Modeling . We hope the information provided has been useful to you. Feel free to contact us if you have any questions or need further assistance. See you next time and don't miss to bookmark.