A Bigram Detector Fires In Response To The
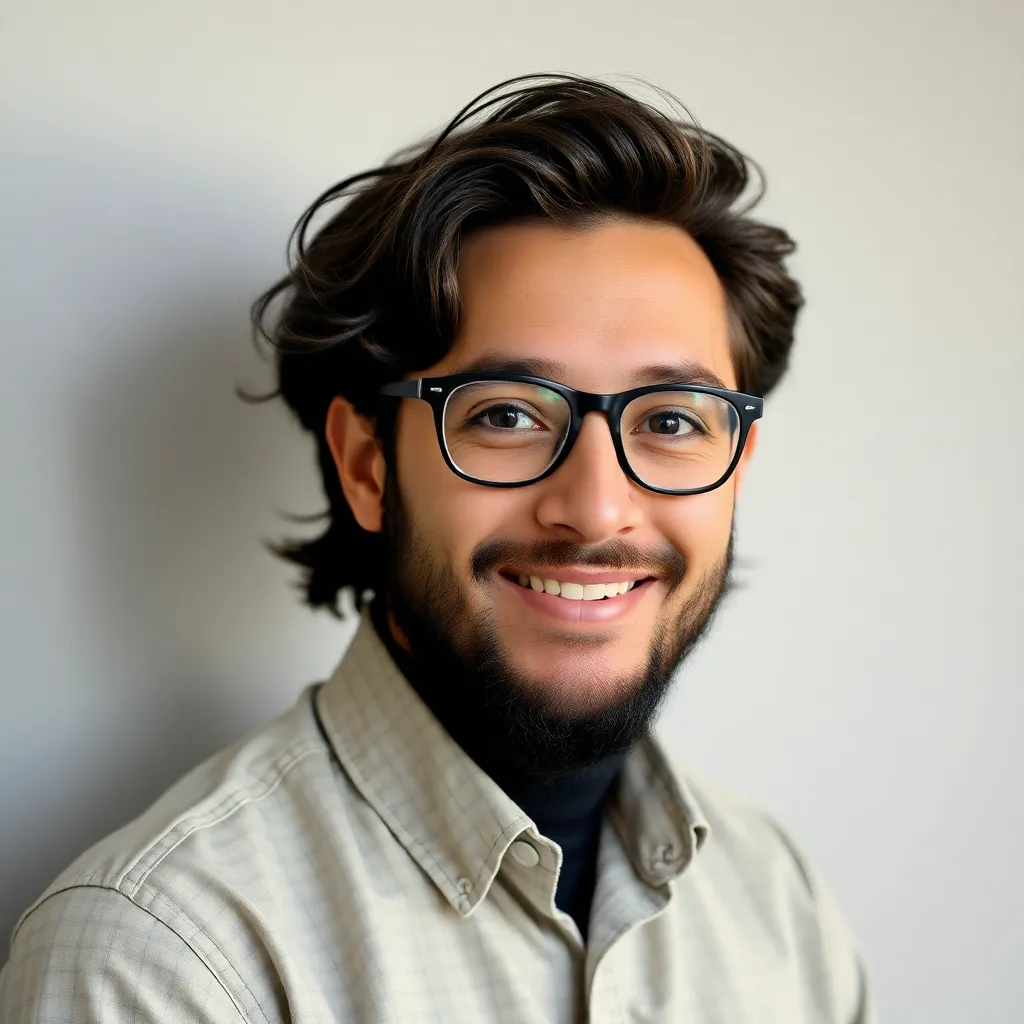
Onlines
Apr 08, 2025 · 5 min read

Table of Contents
A Bigram Detector Fires in Response To: Unveiling the Secrets of N-gram Analysis
The digital age has gifted us with an unprecedented deluge of text data. From social media posts to news articles, emails to scientific papers, the sheer volume is staggering. Extracting meaningful insights from this ocean of words requires sophisticated techniques, and among them, n-gram analysis stands out as a powerful tool. This article delves deep into the workings of n-gram analysis, focusing particularly on bigrams – sequences of two consecutive words – and exploring why a bigram detector might "fire" in response to specific text inputs. We'll uncover the applications, limitations, and future trends of this vital linguistic tool.
Understanding N-grams: The Building Blocks of Text Analysis
Before we dive into the intricacies of bigrams, it's crucial to understand the broader context of n-grams. An n-gram is simply a contiguous sequence of n items from a given sample of text or speech. These items can be words, characters, or even syllables, depending on the application. The "n" in n-gram specifies the length of the sequence:
- Unigrams (n=1): Individual words. Example: "the," "quick," "brown."
- Bigrams (n=2): Pairs of consecutive words. Example: "the quick," "quick brown," "brown fox."
- Trigrams (n=3): Sequences of three consecutive words. Example: "the quick brown," "quick brown fox," "brown fox jumps."
- And so on... You can have 4-grams, 5-grams, and even higher-order n-grams, though the practical application often diminishes as 'n' increases due to data sparsity.
Bigram Detectors: The Watchdogs of Linguistic Patterns
A bigram detector is essentially an algorithm designed to identify and count the occurrences of bigrams within a given text corpus. This seemingly simple function has profound implications across various domains. Imagine a system trained on a massive dataset of Shakespeare's works. When fed a new text, a bigram detector can flag phrases like "fair maiden" or "star-crossed lovers" as high-probability bigrams based on their frequent co-occurrence in Shakespeare's writings. This "firing" of the bigram detector indicates a stylistic similarity to Shakespeare's work.
How Bigram Detectors Work: A Peek Under the Hood
At its core, a bigram detector relies on statistical methods. The process typically involves:
-
Tokenization: Breaking down the input text into individual words. This step requires handling punctuation and other non-alphanumeric characters effectively.
-
Bigram Extraction: Identifying all consecutive pairs of words.
-
Frequency Counting: Counting the occurrences of each unique bigram.
-
Probability Calculation: Often, bigram detectors calculate the probability of each bigram occurring, based on its frequency relative to the total number of bigrams in the corpus. This probability score serves as a measure of how "likely" a given bigram is within the context of the training data.
-
Threshold Setting: A crucial step is defining a threshold. The detector "fires" (indicates a match) only when the probability of a detected bigram exceeds this predefined threshold. Adjusting this threshold allows for control over sensitivity and specificity.
Applications of Bigram Detectors: A Multifaceted Tool
The applications of bigram detectors extend far beyond simple stylistic analysis. Here are some key areas where they find widespread use:
-
Spam Detection: Bigram detectors can effectively identify spam emails by recognizing frequently occurring bigrams associated with spam (e.g., "free money," "guaranteed results"). The firing of the detector for these bigrams can trigger further analysis or automatic filtering.
-
Natural Language Processing (NLP): Bigrams are fundamental in various NLP tasks, including language modeling, part-of-speech tagging, and machine translation. They help predict the likelihood of a word following another, improving the accuracy of these models.
-
Information Retrieval: Search engines utilize bigram analysis to improve search relevance. By identifying frequently co-occurring words in relevant documents, they can better match user queries to appropriate results.
-
Sentiment Analysis: Bigrams can reflect sentiment. For example, the bigram "highly recommended" often indicates positive sentiment, while "utterly disappointed" suggests negative sentiment. Detecting these bigrams helps classify the overall sentiment of a piece of text.
-
Author Identification: As demonstrated with Shakespeare's works, bigram analysis can be employed in authorship attribution, helping to determine the likely author of an anonymous text based on stylistic similarities to known authors.
Limitations and Challenges in Bigram Detection
While bigram detectors are incredibly useful, they are not without their limitations:
-
Data Sparsity: For less frequent bigrams, especially in smaller datasets, reliable probability estimates can be difficult to obtain. This leads to potential inaccuracies.
-
Contextual Ambiguity: Bigrams can be context-dependent. A bigram that appears frequently in one context might have a different meaning or probability in another. This necessitates careful consideration of the training data and the specific application.
-
Computational Cost: Processing massive datasets to extract and analyze bigrams can be computationally expensive, particularly for higher-order n-grams. Efficient algorithms and optimized data structures are crucial for practical implementation.
-
Overfitting: If the training data isn't representative of the broader language, the bigram detector might overfit to the specific characteristics of that dataset and perform poorly on new, unseen data.
Advanced Techniques and Future Trends
Researchers are constantly developing more sophisticated approaches to address the limitations of basic bigram detectors. Some notable advancements include:
-
Smoothed Probabilities: Techniques like Laplace smoothing or Good-Turing smoothing help to mitigate the issue of data sparsity by assigning non-zero probabilities to unseen bigrams.
-
Weighted Bigrams: Instead of treating all bigrams equally, weighted bigrams assign different weights based on their importance or relevance to the specific application.
-
Neural Language Models: Deep learning architectures, such as recurrent neural networks (RNNs) and transformers, are increasingly used for language modeling. These models can capture more complex linguistic patterns than simple bigram analysis, although they generally require much larger datasets and more computational resources.
Conclusion: The Enduring Relevance of Bigrams
Bigram detectors, while seemingly simple in concept, play a critical role in numerous natural language processing and text analysis tasks. Their ability to identify and quantify the co-occurrence of word pairs offers powerful insights into linguistic patterns, stylistic features, and semantic relationships. Despite their limitations, ongoing advancements in statistical methods and deep learning are continually enhancing their capabilities, ensuring their enduring relevance in the ever-evolving landscape of text data analysis. As the volume of digital text continues to grow exponentially, the need for robust and efficient bigram detectors, and n-gram analysis more broadly, will only intensify. The "firing" of a bigram detector, therefore, represents not just a simple algorithmic event, but a significant step in unlocking the rich semantic information embedded within the vast expanse of human language.
Latest Posts
Latest Posts
-
Miguel Y Maru Estan Muy Cansados
Apr 17, 2025
-
Which Box And Whisker Plot Represents The Data Set
Apr 17, 2025
-
How Should The Supported Commander Address Lack Of Support Issues
Apr 17, 2025
-
System Administration Consultation Scenario 2 Github
Apr 17, 2025
-
3 08 Quiz Managing Your Weight Part 2
Apr 17, 2025
Related Post
Thank you for visiting our website which covers about A Bigram Detector Fires In Response To The . We hope the information provided has been useful to you. Feel free to contact us if you have any questions or need further assistance. See you next time and don't miss to bookmark.