A Major Misuse Of Significance Tests Is The Tendency To
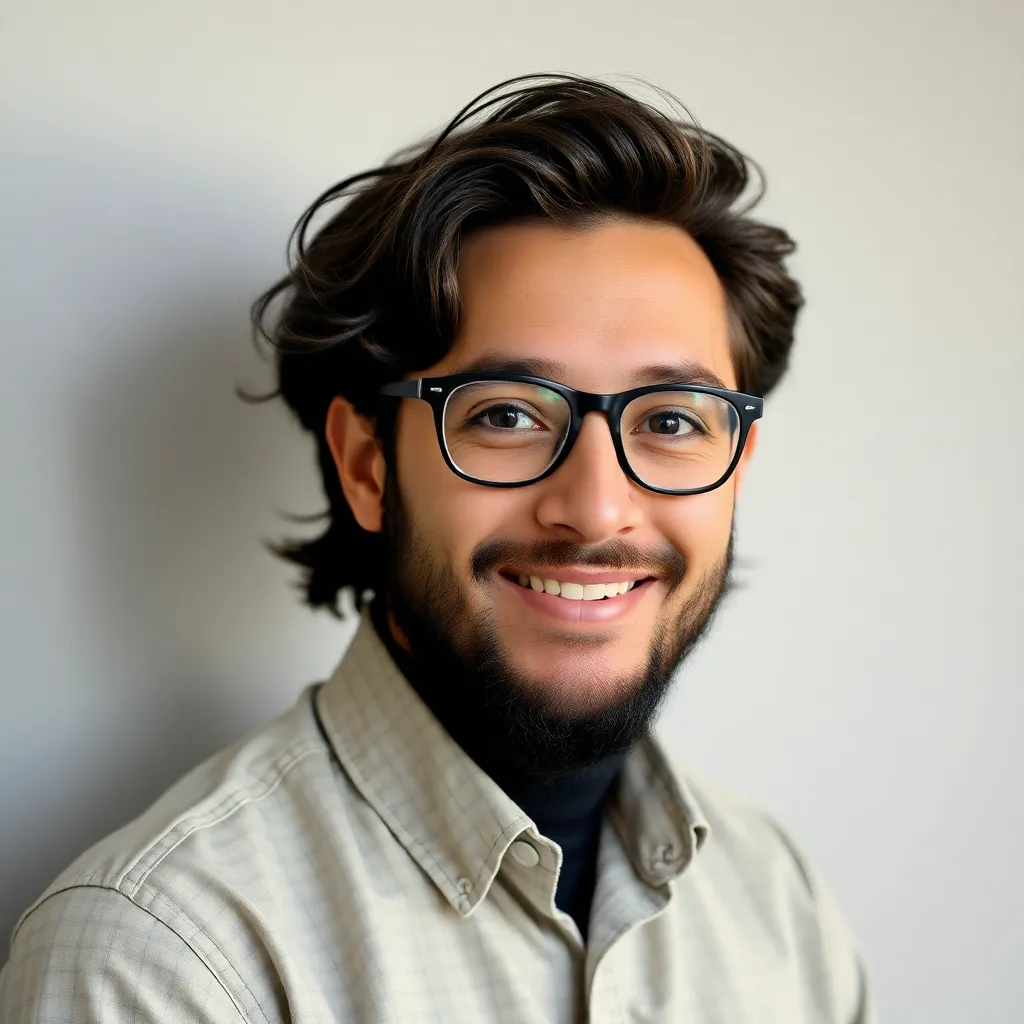
Onlines
May 06, 2025 · 5 min read

Table of Contents
A Major Misuse of Significance Tests: The Tendency to Overemphasize p-Values and Ignore Practical Significance
Significance testing, a cornerstone of statistical inference, plays a crucial role in evaluating research hypotheses. However, a major misuse stems from the overemphasis on p-values and the neglect of practical significance. This article delves into this pervasive issue, exploring its consequences, and offering alternative approaches to enhance the reliability and interpretability of research findings.
The Allure and Pitfalls of p-Values
The p-value, representing the probability of observing results as extreme as, or more extreme than, the obtained results under the null hypothesis, has become a ubiquitous metric in scientific research. A p-value below a predetermined threshold (typically 0.05) leads to the rejection of the null hypothesis, often interpreted as evidence supporting the alternative hypothesis. While seemingly straightforward, this approach harbors several critical flaws:
1. Misinterpretation of p-Values: Statistical Significance vs. Practical Significance
A statistically significant result (p < 0.05) simply indicates that the observed effect is unlikely to have occurred by chance alone. It does not automatically imply practical significance or real-world importance. A small, statistically significant effect might be trivial in a real-world context, particularly when considering the cost, resources, or ethical implications of implementing any resulting changes. For instance, a statistically significant improvement in test scores by 0.1 points might be statistically significant but practically meaningless.
2. The Influence of Sample Size: Inflating Statistical Significance
Larger sample sizes increase the power of a statistical test, making it more likely to detect even small, practically insignificant effects. This can lead to statistically significant results that lack practical relevance. A large study might demonstrate a statistically significant difference between two groups, but the magnitude of that difference might be too small to warrant any meaningful intervention or change in practice.
3. The Problem of Multiple Comparisons: Inflating Type I Error Rate
Conducting multiple statistical tests increases the likelihood of obtaining at least one statistically significant result by chance alone (Type I error). Without appropriate adjustments (e.g., Bonferroni correction), the overall Type I error rate inflates, potentially leading to false-positive conclusions. Researchers need to carefully consider and manage the problem of multiple comparisons to avoid drawing erroneous inferences.
4. Ignoring Effect Size: Quantifying the Magnitude of the Effect
The p-value alone provides limited information. It doesn't quantify the magnitude of the observed effect. Effect size measures (e.g., Cohen's d, Pearson's r) provide crucial information about the practical importance of the findings. A small effect size, even if statistically significant, might be inconsequential in practice. Researchers should always report effect sizes alongside p-values to provide a comprehensive understanding of the results.
5. Focusing on Null Hypothesis Significance Testing (NHST): A Limited Framework
NHST, the dominant approach in many fields, suffers from several limitations. It's inherently binary (reject or fail to reject the null hypothesis), ignoring the possibility of partial support for the alternative hypothesis. It emphasizes statistical significance over practical relevance, potentially leading to misleading conclusions.
Beyond p-Values: Alternative Approaches
To mitigate the misuses associated with over-reliance on p-values, researchers should adopt alternative approaches that emphasize practical significance and provide a more nuanced interpretation of research findings:
1. Emphasize Effect Sizes and Confidence Intervals
Instead of solely focusing on p-values, researchers should prioritize reporting effect sizes and confidence intervals. Effect sizes quantify the magnitude of the observed effect, while confidence intervals provide a range of plausible values for the true effect. This approach provides a more comprehensive and informative assessment of the research findings.
2. Bayesian Statistics: Incorporating Prior Knowledge
Bayesian statistics offers an alternative framework that incorporates prior knowledge and beliefs into the analysis. This approach allows researchers to update their beliefs in light of new data, providing a more nuanced understanding of the evidence. Bayesian methods can be particularly useful when dealing with small sample sizes or when prior knowledge is available.
3. Focus on Practical Significance: Real-World Impact
Researchers should prioritize the practical significance of their findings, considering the real-world impact of the results. This involves evaluating whether the observed effect is large enough to warrant any meaningful intervention or change in practice. This requires careful consideration of the context, costs, resources, and ethical implications involved.
4. Reporting Uncertainty: Acknowledging Limitations
Research findings are inherently uncertain. Researchers should transparently acknowledge limitations, such as sample size, methodological constraints, and potential biases. Reporting uncertainty helps readers understand the context of the findings and avoid overgeneralizations.
5. Replication and Reproducibility: Strengthening Findings
Replication and reproducibility are crucial for validating research findings. Repeating studies using different samples and methodologies strengthens confidence in the robustness of the results. Encouraging replication enhances the reliability and validity of scientific knowledge.
Case Studies Illustrating Misuse of Significance Testing
Numerous examples illustrate the pitfalls of overemphasizing p-values and neglecting practical significance. Consider these scenarios:
- Medicine: A statistically significant reduction in blood pressure by 1 mmHg might be statistically significant but clinically insignificant.
- Education: A statistically significant improvement in test scores by 0.2 points might be statistically significant but practically meaningless.
- Marketing: A statistically significant increase in website clicks by 0.1% might be statistically significant but commercially insignificant.
In each of these cases, the statistically significant result fails to translate into meaningful practical implications. The emphasis on p-values has obscured the lack of practical relevance.
Conclusion: Reframing the Approach to Statistical Inference
The overemphasis on p-values and the neglect of practical significance represent a major misuse of significance testing. This practice can lead to misleading conclusions, misallocation of resources, and flawed decision-making. To enhance the reliability and interpretability of research findings, researchers must shift their focus toward a more holistic approach. This involves:
- Prioritizing effect sizes and confidence intervals alongside p-values.
- Considering practical significance alongside statistical significance.
- Addressing multiple comparisons appropriately.
- Employing alternative statistical frameworks, such as Bayesian methods.
- Transparent reporting of limitations and uncertainty.
- Encouraging replication and reproducibility of studies.
By adopting these strategies, researchers can contribute to a more robust and reliable scientific literature, fostering better decision-making and a more nuanced understanding of research findings. The goal is not to eliminate significance testing altogether, but to use it judiciously as one piece of a much larger puzzle in interpreting and understanding research data. A more holistic and contextualized approach to statistical inference will significantly improve the quality and impact of scientific research.
Latest Posts
Latest Posts
-
Declare An Integer Variable Named Degreescelsius
May 06, 2025
-
Which Of The Following Have Two Congruent Parallel Bases
May 06, 2025
-
What Is The Binary Representation Of 0xca
May 06, 2025
-
Ted Conducts A Study Regarding How Walking On A Treadmill
May 06, 2025
-
Which Statement Is True About Specialized Diets In General
May 06, 2025
Related Post
Thank you for visiting our website which covers about A Major Misuse Of Significance Tests Is The Tendency To . We hope the information provided has been useful to you. Feel free to contact us if you have any questions or need further assistance. See you next time and don't miss to bookmark.