Abram Is A Cognitive Engineer. He Develops ______.
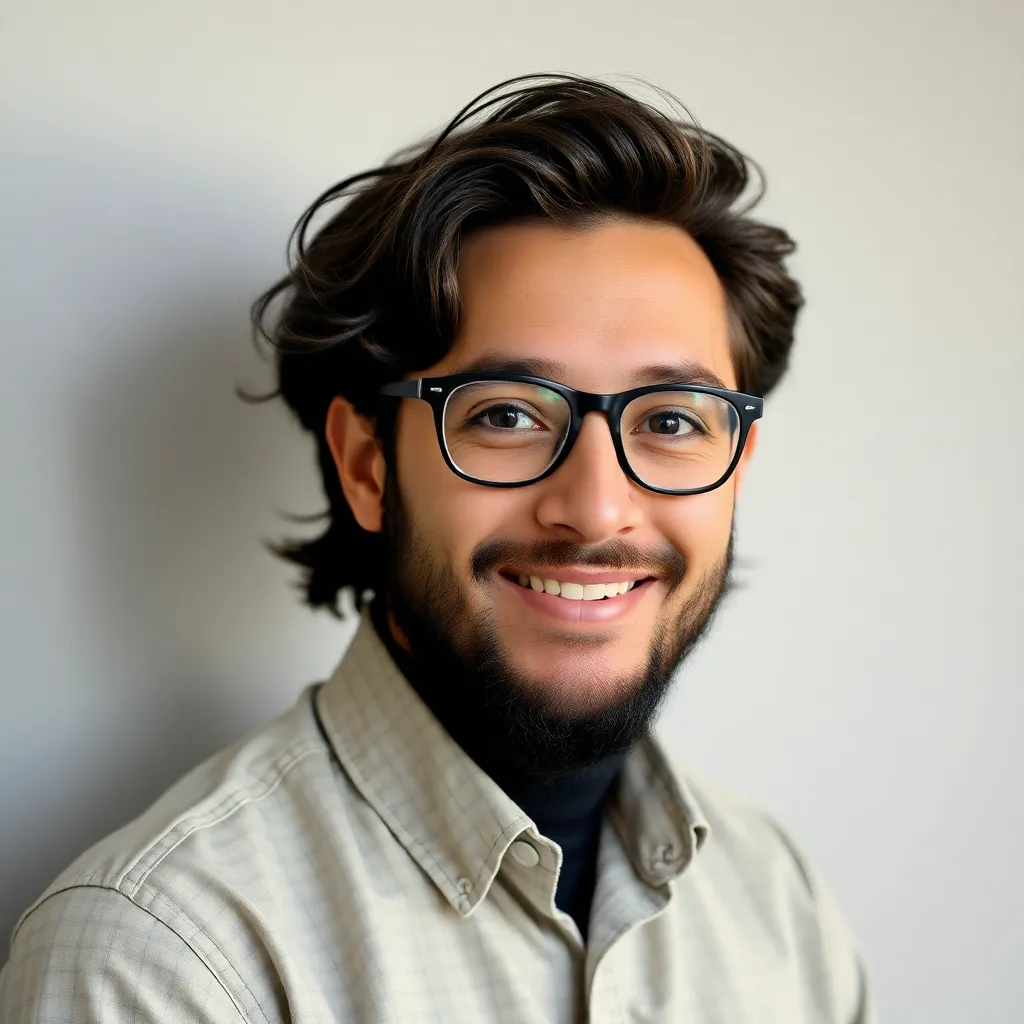
Onlines
Mar 28, 2025 · 7 min read
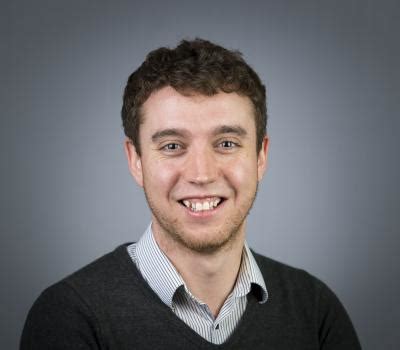
Table of Contents
Abram is a Cognitive Engineer: He Develops Adaptive Learning Systems for Enhanced Human-AI Collaboration
Abram, a highly skilled cognitive engineer, dedicates his expertise to a fascinating and rapidly evolving field: the development of adaptive learning systems designed to enhance human-AI collaboration. His work sits at the crucial intersection of artificial intelligence, cognitive psychology, and human-computer interaction, pushing the boundaries of what's possible in creating intuitive and effective AI tools. Instead of simply building AI that replaces human capabilities, Abram focuses on AI that augments them, creating symbiotic relationships where humans and AI work together seamlessly.
Understanding the Role of a Cognitive Engineer
Before delving into the specifics of Abram's work, it's essential to understand the broader context of cognitive engineering. It's a multidisciplinary field that leverages principles from cognitive psychology, computer science, and human factors engineering to design and develop systems that are compatible with human cognitive abilities and limitations. Cognitive engineers strive to create systems that are:
- Intuitive: Easy to learn and use, requiring minimal cognitive effort.
- Efficient: Optimizing human performance and minimizing errors.
- Adaptable: Responding effectively to the changing needs and contexts of the user.
- Safe: Minimizing the risk of errors and accidents.
Abram's specialization within cognitive engineering is particularly relevant in the current landscape of rapidly advancing AI. As AI systems become more complex and integrated into our lives, the challenge lies not just in building powerful algorithms but also in designing systems that humans can understand, trust, and effectively collaborate with.
Abram's Focus: Adaptive Learning Systems
Abram's core work revolves around adaptive learning systems. These are AI-powered systems that dynamically adjust their teaching methods and content based on the individual learner's performance and understanding. This contrasts with traditional learning systems that employ a one-size-fits-all approach. Adaptive learning systems personalize the learning experience, ensuring that each learner receives the support and challenges they need to succeed.
The Key Features of Abram's Adaptive Learning Systems:
-
Personalized Learning Paths: These systems analyze a learner's strengths and weaknesses, creating a customized learning pathway tailored to their specific needs. This might involve adjusting the difficulty level, focusing on specific concepts that need more attention, or recommending relevant resources.
-
Real-time Feedback and Assessment: Continuous monitoring of learner performance allows the system to provide immediate feedback, identify areas needing improvement, and adjust the learning trajectory accordingly. This immediate feedback loop is crucial for effective learning and helps prevent misconceptions from taking root.
-
Intelligent Tutoring Systems: Some of Abram's systems incorporate intelligent tutoring systems (ITS) which provide personalized guidance and support, acting as virtual tutors that interact with the learner in a conversational manner. These ITS can answer questions, provide explanations, and offer hints when needed.
-
Data-Driven Optimization: Abram's adaptive learning systems rely heavily on data analysis. By tracking learner performance, the systems learn which teaching strategies are most effective and continuously refine their algorithms to optimize the learning process. This data-driven approach ensures that the systems continually improve over time.
The Human-AI Collaboration Aspect
A crucial element differentiating Abram's work is his strong emphasis on human-AI collaboration. His adaptive learning systems are not intended to replace human teachers or instructors. Instead, they are designed to augment their capabilities, empowering them to better serve their students.
How Abram's Systems Enhance Human-AI Collaboration:
-
Teacher Time Optimization: By automating certain tasks such as grading, providing feedback, and creating personalized learning plans, Abram's systems free up teachers' time, allowing them to focus on more complex and nuanced aspects of teaching, like individual student mentoring and curriculum development.
-
Data-Driven Insights: The data collected by the systems provide valuable insights into student learning patterns, allowing teachers to identify areas where their students are struggling and adjust their teaching strategies accordingly. This empowers teachers with evidence-based data to inform their instructional decisions.
-
Personalized Support: While the system provides automated feedback and support, the human teacher can still intervene and provide more personalized attention when necessary. The system and the teacher work in tandem, providing a comprehensive and supportive learning environment.
-
Scalability and Accessibility: Abram's adaptive learning systems can be scaled to reach a large number of students, making quality education more accessible to diverse populations, regardless of geographical location or resource constraints. This has significant implications for bridging educational equity gaps.
The Technological Underpinnings
Abram's systems utilize a sophisticated blend of technologies to achieve their adaptive capabilities. These include:
-
Machine Learning Algorithms: At the heart of the systems are sophisticated machine learning algorithms, capable of analyzing vast amounts of data to identify patterns and make predictions about student performance. These algorithms are constantly refined and improved through ongoing data analysis.
-
Natural Language Processing (NLP): Many of Abram's systems incorporate NLP techniques to allow for natural and intuitive interaction between the learner and the system. This includes features such as conversational tutoring, intelligent question answering, and personalized feedback delivery.
-
Knowledge Representation and Reasoning: The systems need to effectively represent and reason about the knowledge being taught. This often involves the use of ontologies and knowledge graphs, which provide a structured representation of the domain knowledge.
-
User Interface (UI) and User Experience (UX) Design: Abram pays close attention to the user interface and user experience, ensuring that the systems are intuitive, easy to navigate, and engaging for learners of all ages and technological proficiency. A well-designed interface is crucial for maximizing user engagement and learning effectiveness.
Future Directions and Challenges
Abram's work is constantly evolving, driven by advancements in AI and a deeper understanding of human cognition. Future directions include:
-
Incorporating Social-Emotional Learning: Expanding the systems to incorporate social-emotional learning aspects, recognizing that effective learning involves not just cognitive skills but also emotional well-being and social interaction.
-
Enhanced Explainability and Transparency: Improving the transparency and explainability of the systems' decision-making processes to build trust and understanding among users. This is crucial for addressing concerns about the "black box" nature of many AI systems.
-
Addressing Bias and Fairness: Actively mitigating biases in the data used to train the systems, ensuring fairness and equity in access to educational opportunities.
-
Integration with Other Educational Technologies: Seamless integration of Abram's adaptive learning systems with other educational technologies, such as virtual reality and gamification, to create richer and more immersive learning experiences.
Despite the significant progress, challenges remain:
-
Data Privacy and Security: Ensuring the privacy and security of the learner data collected by the systems is a paramount concern. Robust data protection measures are necessary to maintain user trust.
-
Ethical Considerations: Careful consideration of the ethical implications of using AI in education, particularly concerning potential biases, fairness, and accountability.
-
Teacher Training and Support: Providing adequate training and support to teachers to help them effectively integrate these new technologies into their teaching practices.
Conclusion
Abram's work in developing adaptive learning systems represents a significant advancement in the field of cognitive engineering and AI-powered education. His focus on human-AI collaboration ensures that these systems serve as powerful tools to augment human capabilities, rather than replace them. By addressing the challenges and pursuing the future directions outlined above, Abram and others in the field are paving the way for a future where AI plays a transformative role in enhancing learning and fostering educational equity. His contributions are not just technological, but also pedagogical and societal, promising to reshape the landscape of education for the better. The seamless integration of AI into the learning process, personalized to the individual learner, holds immense potential for improving educational outcomes globally, and Abram's contributions are pivotal in realizing this potential. His dedication to creating truly adaptive and collaborative learning environments positions him as a leader in the future of education.
Latest Posts
Latest Posts
-
Practice Complex Inheritance Patterns Answer Key
Mar 31, 2025
-
The Guest Summary By Albert Camus
Mar 31, 2025
-
The Great Gatsby Student Workbook Answer Key Pdf
Mar 31, 2025
-
Statement Of Cash Flows For Snowdrop A Limited Company
Mar 31, 2025
-
What Page Does Juror 10 Talk About African Americnas
Mar 31, 2025
Related Post
Thank you for visiting our website which covers about Abram Is A Cognitive Engineer. He Develops ______. . We hope the information provided has been useful to you. Feel free to contact us if you have any questions or need further assistance. See you next time and don't miss to bookmark.