Determine Which Plot Shows The Strongest Linear Correlation.
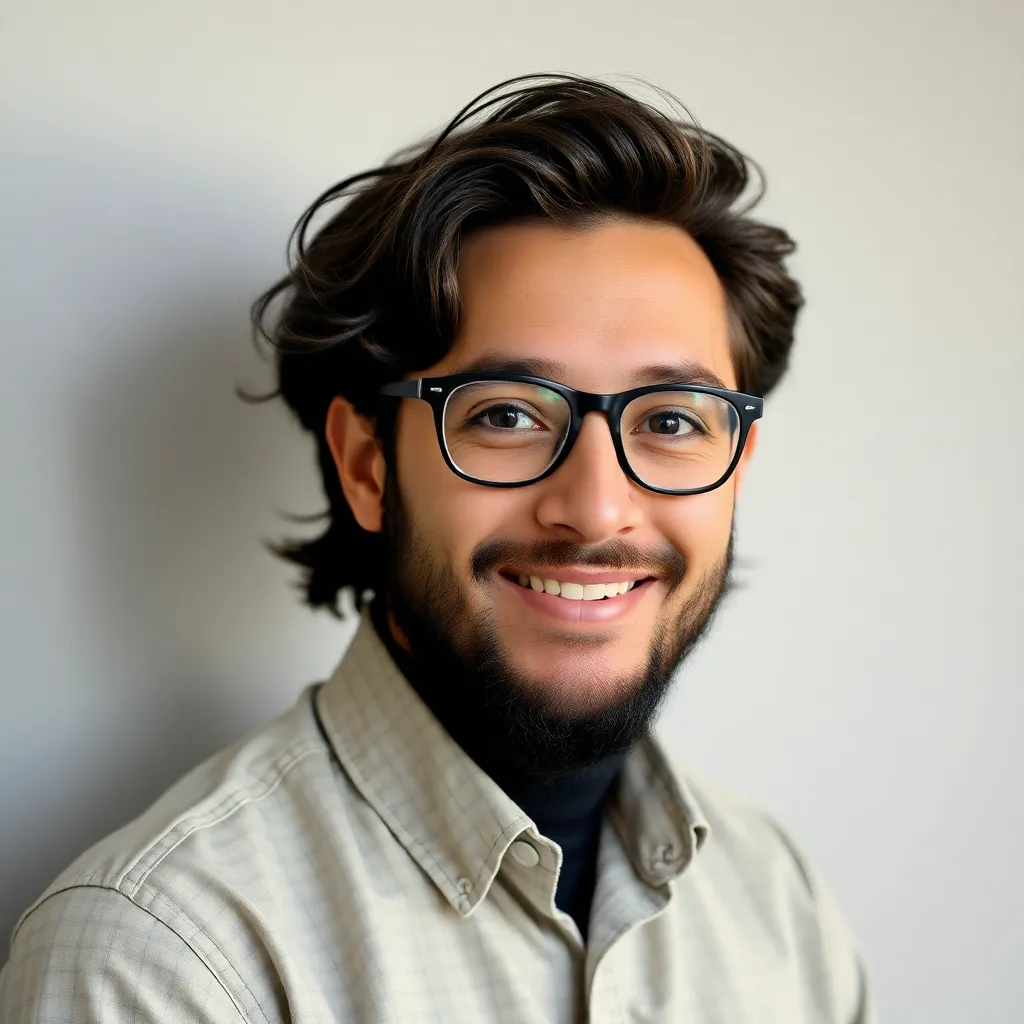
Onlines
May 08, 2025 · 5 min read
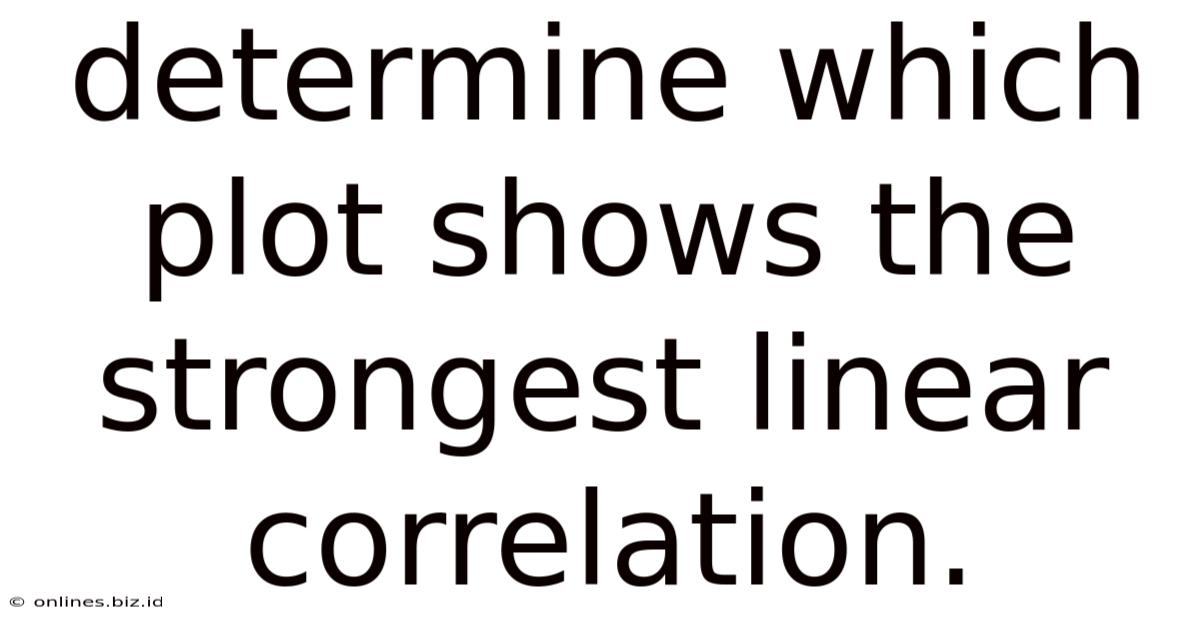
Table of Contents
Determining the Strongest Linear Correlation: A Comprehensive Guide
Understanding correlation is crucial in many fields, from scientific research to financial modeling. Linear correlation, specifically, measures the strength and direction of a linear relationship between two variables. But how do you determine which of several plots exhibits the strongest linear correlation? This comprehensive guide will explore various methods, providing you with the tools to confidently analyze datasets and identify the most compelling linear relationships.
What is Linear Correlation?
Before diving into methods for comparison, let's solidify our understanding of linear correlation. It quantifies the linear association between two variables, typically represented as a scatter plot. A strong positive correlation indicates that as one variable increases, the other tends to increase proportionally. Conversely, a strong negative correlation suggests that as one variable increases, the other tends to decrease proportionally. A weak or no correlation implies little to no linear relationship between the variables.
Visual Inspection: A First Impression
The simplest approach is visual inspection. Examine each scatter plot carefully. Look for:
- Clustering of points: A strong linear correlation will show points tightly clustered around a straight line. The more tightly clustered, the stronger the correlation.
- Direction of the line: Determine whether the general trend is upward (positive correlation) or downward (negative correlation).
- Outliers: Extreme values (outliers) can skew the perception of correlation. Consider their impact on the overall trend.
While visual inspection provides a quick overview, it's subjective and not suitable for precise comparison. Quantitative measures are needed for accurate determination.
Quantitative Measures: Pearson's Correlation Coefficient (r)
The most widely used quantitative measure of linear correlation is Pearson's correlation coefficient (r). It ranges from -1 to +1:
- r = +1: Perfect positive linear correlation
- r = -1: Perfect negative linear correlation
- r = 0: No linear correlation
Values between these extremes indicate varying degrees of correlation. For example, r = 0.8 suggests a strong positive correlation, while r = -0.6 indicates a moderate negative correlation.
Interpreting r:
Strong | Moderate | Weak | |
---|---|---|---|
Positive | 0.8 - 1.0 | 0.5 - 0.79 | 0.3 - 0.49 |
Negative | -0.8 - -1.0 | -0.5 - -0.79 | -0.3 - -0.49 |
Calculating Pearson's r:
The formula for calculating Pearson's r is:
r = Σ[(xi - x̄)(yi - ȳ)] / √[Σ(xi - x̄)²Σ(yi - ȳ)²]
Where:
- xi and yi are individual data points for variables x and y, respectively.
- x̄ and ȳ are the means of variables x and y, respectively.
- Σ denotes summation.
While the formula looks complex, statistical software packages (like R, Python's SciPy, Excel, etc.) readily calculate Pearson's r, making manual calculation unnecessary in most cases.
Comparing Multiple Plots: A Step-by-Step Approach
To determine which plot shows the strongest linear correlation among several, follow these steps:
-
Calculate Pearson's r for each plot: Use statistical software to calculate the correlation coefficient for each dataset.
-
Examine the absolute value of r: The absolute value of r (|r|) indicates the strength of the correlation, regardless of direction. The higher the absolute value, the stronger the linear correlation.
-
Compare the absolute values: Compare the |r| values for all plots. The plot with the highest |r| exhibits the strongest linear correlation.
-
Consider the p-value: Along with r, statistical software will also provide a p-value. This represents the probability of observing such a correlation by chance if there's no actual correlation. A small p-value (typically below 0.05) suggests the correlation is statistically significant. While the highest |r| indicates the strongest correlation, ensure the corresponding p-value is statistically significant to confirm the result's reliability.
-
Visual Inspection (Secondary): After quantitative analysis, revisit the scatter plots. The plot with the highest |r| and statistically significant p-value should indeed visually represent the strongest linear correlation. This helps to corroborate the quantitative findings.
Beyond Pearson's r: Addressing Non-Linear Relationships and Outliers
Pearson's r is excellent for linear relationships. However, if the relationship between variables isn't linear (e.g., curved), Pearson's r might underestimate or misrepresent the true association. In such cases, consider:
-
Spearman's rank correlation: This non-parametric method measures the monotonic relationship (consistent increase or decrease) between variables, regardless of linearity. It's less sensitive to outliers than Pearson's r.
-
Visual inspection for non-linearity: Examine scatter plots for clear curves or patterns suggesting a non-linear relationship. Consider transformations of the data (like logarithmic or square root transformations) to linearize the relationship if possible. If linearization fails, then applying non-parametric methods like Spearman’s rank correlation becomes important.
-
Outlier analysis: Identify and assess the impact of outliers. Outliers can disproportionately affect Pearson's r. Techniques include robust regression or removing outliers if justified (with careful consideration and documentation).
Real-World Examples and Applications
The ability to determine the strongest linear correlation has diverse applications:
-
Finance: Analyzing stock prices, interest rates, and economic indicators to identify relationships and predict future trends.
-
Medicine: Studying the relationship between lifestyle factors (e.g., diet, exercise) and health outcomes (e.g., blood pressure, cholesterol levels).
-
Engineering: Analyzing the relationship between material properties and performance characteristics.
-
Environmental science: Studying the correlation between pollution levels and environmental impacts.
-
Social sciences: Analyzing the relationship between social factors (e.g., education, income) and social outcomes (e.g., crime rates, poverty).
Conclusion: A Holistic Approach
Determining the strongest linear correlation requires a balanced approach combining visual inspection with quantitative analysis. Pearson's r is a powerful tool, but remember its limitations regarding non-linear relationships and outliers. When necessary, explore alternative methods like Spearman's rank correlation, and always consider the statistical significance (p-value) of your findings. By applying these techniques systematically, you can confidently identify the strongest linear correlation within a set of datasets, facilitating informed decisions across numerous fields. Remember to document your methods clearly, including the chosen correlation coefficient, p-values, and any outlier handling strategies. Transparency and rigorous methodology are essential for reliable conclusions.
Latest Posts
Latest Posts
-
Factorial Designs Are Often Employed Because
May 08, 2025
-
Calculating Entropy Change Using The Boltzmann Hypothesis
May 08, 2025
-
During Assessment Scenarios Instructors Are Responsible For
May 08, 2025
-
Periodic Table Of Acquisition Innovations Interactive Q And A
May 08, 2025
-
Advertisers On A Display Network Can Pay
May 08, 2025
Related Post
Thank you for visiting our website which covers about Determine Which Plot Shows The Strongest Linear Correlation. . We hope the information provided has been useful to you. Feel free to contact us if you have any questions or need further assistance. See you next time and don't miss to bookmark.