Emerging Technologies In Cybersecurity - C844
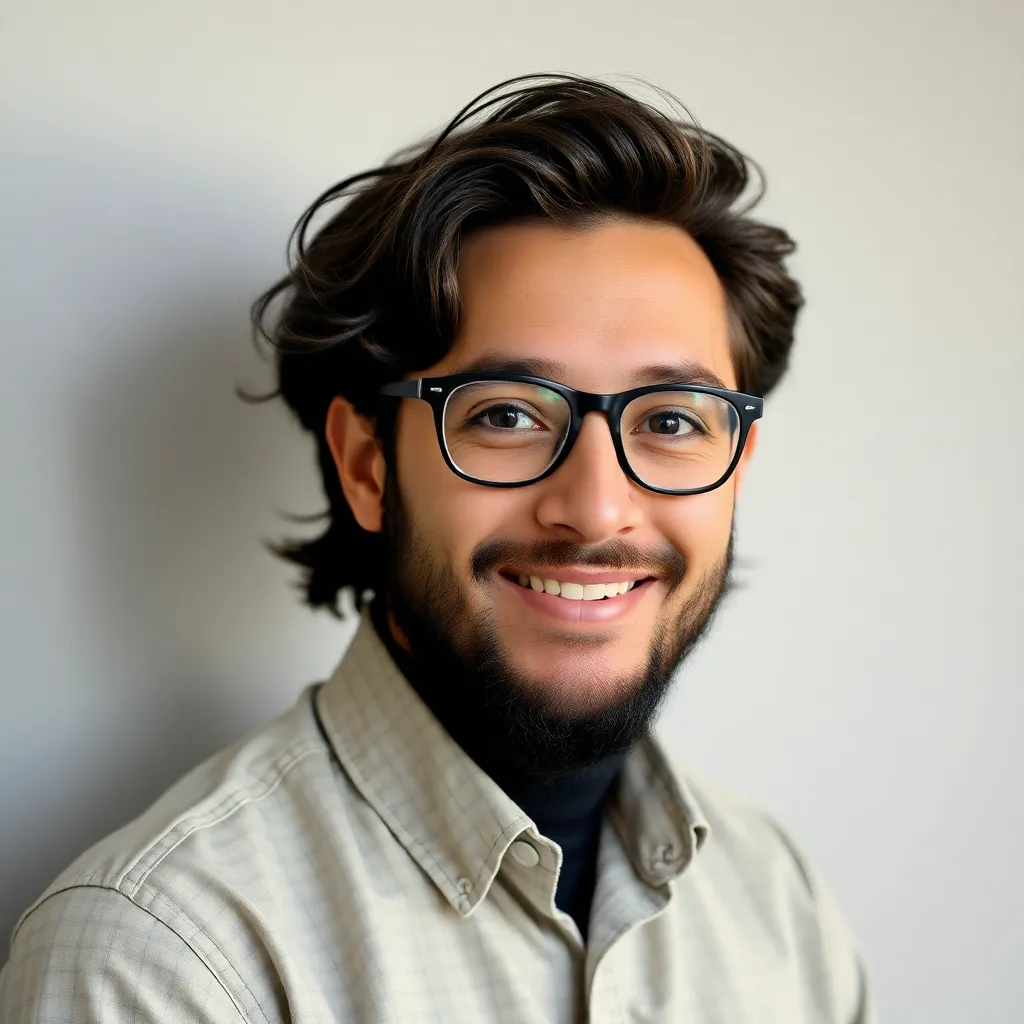
Onlines
Mar 26, 2025 · 7 min read
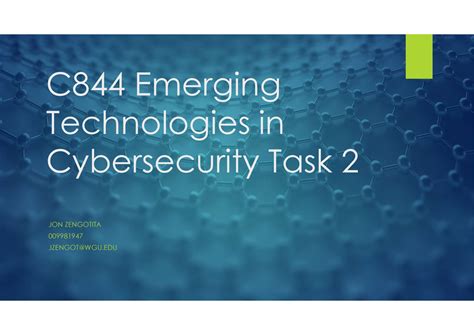
Table of Contents
Emerging Technologies in Cybersecurity: Fortifying the Digital Frontier (C844)
The digital landscape is constantly evolving, with new technologies emerging at an unprecedented pace. This rapid innovation presents both incredible opportunities and significant challenges, particularly in the realm of cybersecurity. As cyber threats become increasingly sophisticated and prevalent, the need for advanced security measures is paramount. This article delves into the emerging technologies shaping the future of cybersecurity, exploring their capabilities and potential impact on protecting our digital assets. We’ll examine how these innovations are transforming how we approach threat detection, prevention, and response.
Artificial Intelligence (AI) and Machine Learning (ML) in Cybersecurity
AI and ML are arguably the most transformative technologies impacting cybersecurity today. Their ability to analyze massive datasets, identify patterns, and learn from past experiences makes them invaluable tools in combating cyber threats.
AI-Powered Threat Detection and Prevention:
- Anomaly Detection: AI algorithms can analyze network traffic and system logs to identify deviations from established baselines. These anomalies, often indicative of malicious activity, can be flagged for immediate investigation, allowing for faster response times to potential threats. This proactive approach is far more effective than relying solely on reactive measures.
- Predictive Analysis: By analyzing historical threat data and current trends, AI can predict potential attacks before they occur. This predictive capability allows organizations to proactively strengthen their defenses, focusing resources on areas most vulnerable to attack. Imagine AI predicting a phishing campaign targeting your employees based on past successful attacks against similar organizations.
- Automated Response: AI can automate many aspects of incident response, such as isolating infected systems, blocking malicious traffic, and patching vulnerabilities. This automation significantly reduces the time it takes to contain a security breach, minimizing potential damage.
Machine Learning for Malware Detection:
ML algorithms excel at identifying malicious software. By analyzing the characteristics of known malware samples, ML models can learn to identify new and previously unseen malware variants. This capability is crucial in combating the ever-evolving nature of malware, which constantly adapts to evade traditional signature-based detection methods.
Challenges of AI in Cybersecurity:
While AI offers significant benefits, it also presents certain challenges:
- Adversarial Attacks: Attackers can design sophisticated attacks specifically aimed at deceiving AI systems. These adversarial attacks exploit vulnerabilities in the AI algorithms, rendering them ineffective.
- Data Bias: AI models are trained on data, and if that data is biased, the resulting model will also be biased. This can lead to inaccurate predictions and missed threats. Ensuring diverse and representative training data is crucial.
- Explainability: Understanding why an AI system made a particular decision is crucial for trust and accountability. Many AI models, particularly deep learning models, are "black boxes," making it difficult to interpret their reasoning. This lack of explainability can hinder debugging and troubleshooting.
Blockchain Technology in Cybersecurity
Blockchain, the technology underpinning cryptocurrencies, offers unique advantages in enhancing cybersecurity. Its decentralized, immutable nature makes it a powerful tool for securing sensitive data and managing digital identities.
Enhancing Data Security and Integrity:
Blockchain's decentralized architecture makes it resistant to single points of failure. Data stored on a blockchain is replicated across multiple nodes, making it extremely difficult to alter or delete. This enhanced data integrity is crucial for applications requiring high levels of trust and security.
Secure Identity Management:
Blockchain can streamline identity management by creating secure, verifiable digital identities. These identities can be used for authentication and authorization, reducing the risk of identity theft and unauthorized access. Imagine a system where your digital identity is securely stored and managed on a blockchain, eliminating the need for numerous passwords and vulnerable usernames.
Supply Chain Security:
Blockchain can improve the security of supply chains by providing a transparent and auditable record of product movement and provenance. This can help prevent the introduction of counterfeit goods or compromised components. Imagine tracking the journey of a crucial computer component from manufacturing to its integration into a finished device, ensuring its authenticity and eliminating the risk of tampering.
Challenges of Blockchain in Cybersecurity:
- Scalability: Current blockchain implementations can struggle to handle large volumes of transactions, limiting their applicability to certain use cases.
- Complexity: Implementing and managing blockchain systems can be complex, requiring specialized expertise and infrastructure.
- Regulation: The regulatory landscape surrounding blockchain is still evolving, creating uncertainty for organizations looking to adopt the technology.
Quantum Computing and Post-Quantum Cryptography
Quantum computers, while still in their early stages of development, pose a significant threat to current cryptographic methods. Their immense computational power could break widely used encryption algorithms, rendering sensitive data vulnerable. This necessitates the development of post-quantum cryptography (PQC).
Post-Quantum Cryptography (PQC):
PQC refers to cryptographic algorithms that are designed to be resistant to attacks from both classical and quantum computers. These algorithms are crucial for protecting sensitive data in the future quantum computing era. Governments and organizations are actively researching and developing PQC algorithms to ensure the long-term security of their systems.
Quantum Key Distribution (QKD):
QKD uses the principles of quantum mechanics to securely distribute encryption keys. Any attempt to intercept the keys will inevitably alter them, alerting the communicating parties to the intrusion. QKD offers a fundamentally secure way to exchange cryptographic keys, eliminating the risk of key compromise.
Challenges of Quantum Computing and PQC:
- Technological Maturity: Quantum computers are still under development, and their widespread availability is some time away. However, the potential threat they pose requires proactive measures.
- Algorithm Development and Standardization: The development and standardization of PQC algorithms is an ongoing process, and it's crucial to ensure the chosen algorithms are robust and secure.
- Implementation Costs: Implementing PQC and QKD can be expensive, requiring significant investment in new hardware and software.
Zero Trust Security
Zero trust security is a security model that assumes no implicit trust granted to any user, device, or network, regardless of location. This model moves away from traditional perimeter-based security and focuses on verifying every access request individually.
Microsegmentation and Least Privilege:
Zero trust emphasizes microsegmentation, dividing the network into smaller, isolated segments. This limits the impact of a potential breach, preventing attackers from easily moving laterally across the network. It also incorporates the principle of least privilege, granting users only the minimum access necessary to perform their tasks.
Continuous Authentication and Authorization:
Zero trust continuously verifies the identity and authorization of users and devices. This can involve multi-factor authentication, device posture assessment, and behavioral analytics. This continuous verification significantly reduces the window of vulnerability.
Data Loss Prevention (DLP):
Zero trust employs robust DLP measures to prevent sensitive data from leaving the organization's control. This includes encryption, access controls, and data monitoring.
Challenges of Zero Trust Security:
- Complexity: Implementing zero trust can be complex, requiring significant changes to existing infrastructure and security policies.
- Cost: Zero trust implementation can be expensive, requiring investments in new technologies and expertise.
- Integration: Integrating zero trust with existing security systems can be challenging.
Extended Detection and Response (XDR)
XDR consolidates security data from various sources, such as endpoints, networks, and cloud environments, to provide a unified view of the security landscape. This holistic approach improves threat detection and response capabilities.
Centralized Security Monitoring and Management:
XDR provides a centralized platform for monitoring and managing security events across various systems. This improved visibility enhances threat detection and speeds up incident response.
Advanced Threat Hunting and Investigation:
XDR's consolidated data allows for more effective threat hunting and investigation. Security analysts can use this data to identify and respond to advanced threats that might be missed by traditional security tools.
Automation of Security Operations:
XDR can automate many aspects of security operations, such as threat detection, investigation, and response. This automation frees up security personnel to focus on more strategic tasks.
Challenges of XDR:
- Data Integration: Integrating data from diverse sources can be complex and require significant effort.
- Data Volume and Velocity: XDR generates a large volume of data, requiring robust infrastructure and processing capabilities.
- Skill Requirements: Effectively using XDR requires skilled security personnel capable of interpreting and analyzing the data.
Conclusion: A Multifaceted Approach to Cybersecurity
The future of cybersecurity relies on a multifaceted approach that leverages the strengths of emerging technologies. While AI, ML, blockchain, quantum computing, zero trust, and XDR offer significant advancements in threat detection, prevention, and response, it's crucial to acknowledge their limitations and address the challenges they present. A comprehensive strategy involves integrating these technologies strategically, fostering collaboration across the cybersecurity community, and prioritizing continuous learning and adaptation. The digital frontier demands a proactive and evolving defense, one that embraces innovation while remaining vigilant against the ever-changing landscape of cyber threats. The successful deployment of these technologies requires not only technological advancements but also a skilled workforce capable of understanding and implementing these complex systems. Continuous training and education are essential for maintaining a robust and adaptive cybersecurity posture.
Latest Posts
Latest Posts
-
Why Did The Rabbit Wear A Shower Cap
Mar 30, 2025
-
Dna Base Pairing Worksheet Answer Sheet
Mar 30, 2025
-
How Does Shakespeare Use The Motif Of Morning
Mar 30, 2025
-
Which Characteristic Is True Of Credentialing
Mar 30, 2025
-
Unit 5 Polynomial Functions Homework 2 Answer Key
Mar 30, 2025
Related Post
Thank you for visiting our website which covers about Emerging Technologies In Cybersecurity - C844 . We hope the information provided has been useful to you. Feel free to contact us if you have any questions or need further assistance. See you next time and don't miss to bookmark.