For The Distribution Drawn Here Identify The Mean
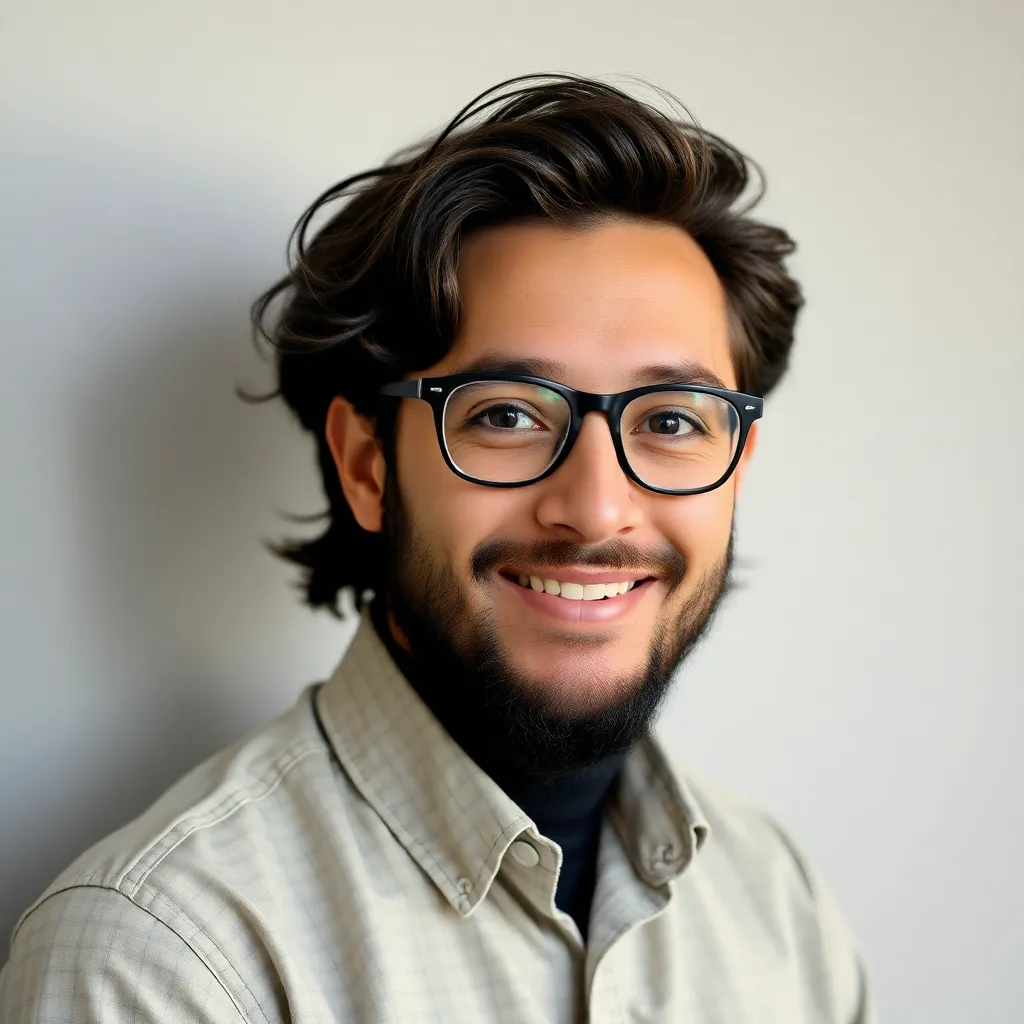
Onlines
Apr 17, 2025 · 6 min read
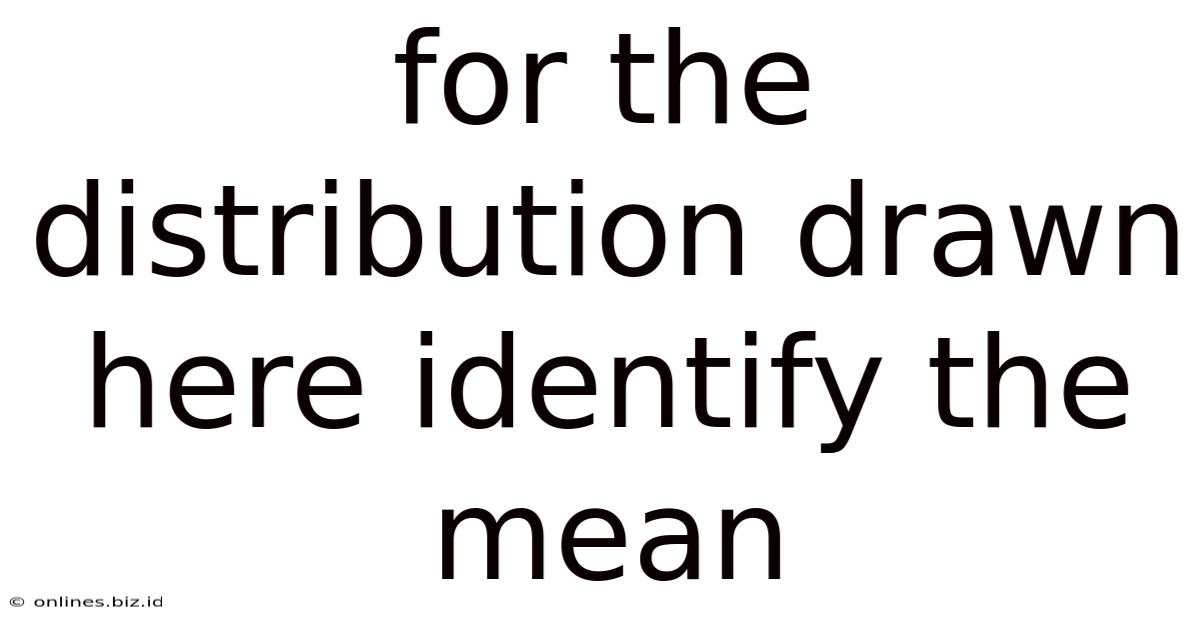
Table of Contents
For the Distribution Drawn Here, Identify the Mean: A Comprehensive Guide
Understanding the mean, or average, of a distribution is fundamental in statistics. It's a measure of central tendency, telling us where the "center" of a dataset lies. However, identifying the mean depends heavily on the type of distribution presented. This article will explore various distribution types, demonstrating how to calculate the mean in each case, and providing practical examples to solidify your understanding. We'll cover discrete distributions, continuous distributions, and even address situations where the distribution is graphically represented rather than given numerically.
Understanding Different Types of Distributions
Before diving into calculations, it's crucial to distinguish between the different kinds of distributions you might encounter:
1. Discrete Distributions:
Discrete distributions deal with data that can only take on specific, separate values. Think of counting something – the number of heads in five coin flips, the number of cars passing a certain point in an hour, etc. Common examples include:
- Binomial Distribution: This distribution models the probability of getting a certain number of "successes" in a fixed number of independent trials, each with the same probability of success.
- Poisson Distribution: Used to model the probability of a given number of events occurring in a fixed interval of time or space if these events occur with a known average rate and independently of the time since the last event.
- Geometric Distribution: This describes the probability of the number of trials needed to get the first success in a sequence of independent Bernoulli trials.
2. Continuous Distributions:
Continuous distributions deal with data that can take on any value within a given range. Think of measurements – height, weight, temperature. Some prominent examples include:
- Normal Distribution (Gaussian Distribution): This is the bell-shaped curve, ubiquitous in statistics due to its frequent appearance in natural phenomena.
- Uniform Distribution: Every value within a given range has an equal probability of occurring.
- Exponential Distribution: Often used to model the time until an event occurs in a Poisson process.
Calculating the Mean for Different Distributions
The method for calculating the mean varies slightly depending on the type of distribution and how the data is presented.
1. Calculating the Mean from a Discrete Probability Distribution:
For a discrete distribution, the mean (often denoted as μ or E(X)) is calculated as the weighted average of all possible values, where the weights are the probabilities of those values. The formula is:
μ = Σ [x * P(x)]
Where:
- x represents each possible value of the random variable.
- P(x) is the probability of x occurring.
- Σ denotes summation over all possible values of x.
Example:
Suppose we have a discrete probability distribution as follows:
x | P(x) |
---|---|
1 | 0.2 |
2 | 0.3 |
3 | 0.5 |
The mean would be:
μ = (1 * 0.2) + (2 * 0.3) + (3 * 0.5) = 0.2 + 0.6 + 1.5 = 2.3
2. Calculating the Mean from a Continuous Probability Distribution:
For a continuous distribution, the mean is calculated using integration. The formula is:
μ = ∫ x * f(x) dx
Where:
- x represents the value of the random variable.
- f(x) is the probability density function (PDF) of the distribution.
- The integral is taken over the entire range of x.
This calculation can be complex and often requires specialized knowledge of calculus. For common distributions like the normal distribution, the mean is a parameter of the distribution itself. For example, a normal distribution is defined by its mean (μ) and standard deviation (σ), often written as N(μ, σ).
3. Calculating the Mean from a Frequency Distribution (Grouped Data):
When data is grouped into classes or intervals, we estimate the mean using the midpoint of each class interval.
μ ≈ Σ [mᵢ * fᵢ] / Σ fᵢ
Where:
- mᵢ is the midpoint of the i-th class interval.
- fᵢ is the frequency of the i-th class interval.
- Σ fᵢ is the total frequency (sum of all frequencies).
4. Calculating the Mean from a Graphical Representation:
If the distribution is presented graphically (e.g., histogram, bar chart, or even a scatter plot if the data is deemed to have a general pattern), estimating the mean requires careful observation and potentially some approximations.
- Symmetrical Distributions: For a perfectly symmetrical distribution, the mean is located at the point of symmetry.
- Skewed Distributions: For skewed distributions, the mean is pulled towards the tail of the distribution. A visual estimation can be made, but it will be less precise than calculations based on numerical data.
Advanced Considerations and Real-World Applications
The concept of the mean extends beyond simple calculations. Understanding the mean's relationship to other statistical measures, such as the median (middle value) and mode (most frequent value), is essential for a comprehensive analysis. The differences between these measures can reveal important insights about the shape and characteristics of a distribution, particularly its symmetry or skewness. For instance, a significant difference between the mean and the median often indicates skewness in the data.
The mean finds widespread application in numerous fields:
- Finance: Calculating average returns on investments, average transaction values.
- Engineering: Determining average product dimensions, average process times.
- Healthcare: Calculating average patient recovery times, average hospital stays.
- Social Sciences: Analyzing average incomes, average test scores, average age of a population.
Dealing with Outliers and Data Transformations
Outliers, data points significantly different from the rest, can heavily influence the mean. A single extreme outlier can dramatically inflate or deflate the average, providing a misleading representation of the central tendency. In such cases, robust measures of central tendency, like the median, might be more appropriate. Alternatively, you may need to investigate the cause of the outliers and decide whether they should be included in the analysis or handled differently (e.g., removed or transformed).
Data transformation techniques can also be applied to address issues like skewness in the data, making the mean a more reliable measure of central tendency. Common transformations include logarithmic transformations or square root transformations, but the choice of transformation will depend on the specific characteristics of the data and the goals of the analysis.
Conclusion
Identifying the mean of a distribution is a crucial skill in statistics. The approach varies based on whether the distribution is discrete or continuous, how the data is presented, and whether there are outliers or skewness to consider. By understanding these different approaches and their nuances, you can effectively analyze data and draw meaningful conclusions, whether working with raw numerical data or visual representations of distributions. Remember to always carefully consider the context of your data and choose the most appropriate method for calculating and interpreting the mean. Thorough understanding of your data and the limitations of statistical measures is key to reliable and meaningful insights.
Latest Posts
Latest Posts
-
Electrical Bonding Investigation Data Quick Check
Apr 19, 2025
-
1 16 Unit Test Basic Tools And Transformations
Apr 19, 2025
-
Cellular Respiration Graphic Organizer Answer Key Pdf
Apr 19, 2025
-
The Storyboard Should Be Created With Software Not Drawn Freehand
Apr 19, 2025
-
Effective Capacity Is The Capacity Allowances Such As Maintenance
Apr 19, 2025
Related Post
Thank you for visiting our website which covers about For The Distribution Drawn Here Identify The Mean . We hope the information provided has been useful to you. Feel free to contact us if you have any questions or need further assistance. See you next time and don't miss to bookmark.