Identify The True And False Statements About Multiple-regression Analyses.
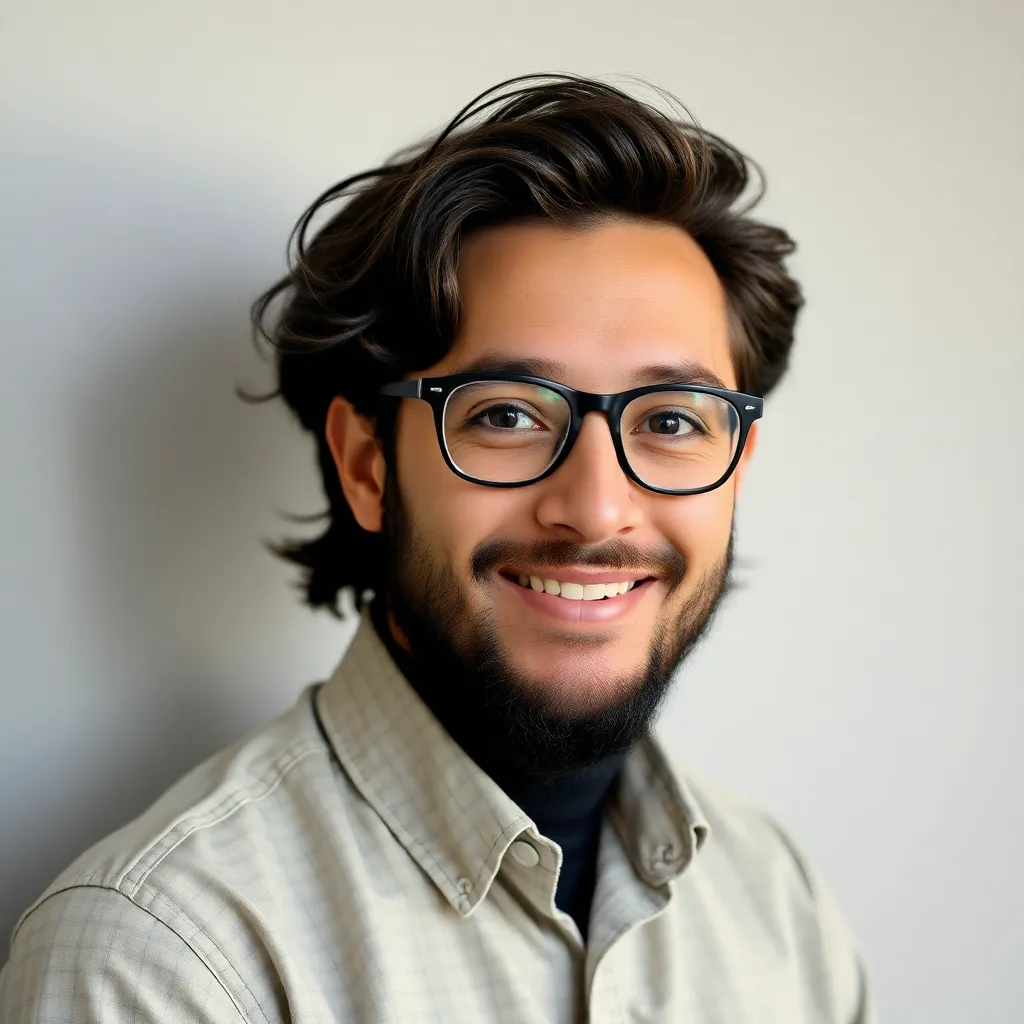
Onlines
Mar 30, 2025 · 7 min read

Table of Contents
Identifying True and False Statements About Multiple Regression Analyses
Multiple regression analysis is a powerful statistical technique used to model the relationship between a dependent variable and two or more independent variables. Understanding its intricacies is crucial for accurate interpretation and application. This article delves into common statements about multiple regression, identifying those that are true and those that are false, providing explanations and highlighting potential pitfalls.
True Statements About Multiple Regression Analyses
1. Multiple regression can model both linear and non-linear relationships.
TRUE. While standard multiple regression assumes a linear relationship between the independent and dependent variables, transformations of variables (e.g., logarithmic, polynomial) can accommodate non-linear associations. This allows for the modeling of a wider range of relationships beyond simple straight lines. Techniques like polynomial regression explicitly incorporate higher-order terms to capture curvature in the relationship.
2. Multiple regression can help identify the relative importance of predictor variables.
TRUE. By examining standardized regression coefficients (beta weights), we can assess the relative contribution of each independent variable to the prediction of the dependent variable, controlling for the effects of other predictors. However, it's crucial to remember that the relative importance can be context-dependent and influenced by the presence of other variables in the model.
3. Multiple regression analysis requires assumptions to be met for valid inferences.
TRUE. The validity of the results hinges on several assumptions, including linearity, independence of errors, homoscedasticity (constant variance of errors), normality of errors, and absence of multicollinearity (high correlation between independent variables). Violations of these assumptions can lead to biased or inefficient estimates.
4. Multiple R-squared indicates the proportion of variance in the dependent variable explained by the model.
TRUE. R-squared, also known as the coefficient of determination, represents the percentage of the total variation in the dependent variable that is accounted for by the independent variables included in the model. A higher R-squared suggests a better fit, but it's not always indicative of a better model, especially if overfitting occurs.
5. Adjusted R-squared penalizes the inclusion of irrelevant predictors.
TRUE. Unlike R-squared, the adjusted R-squared considers the number of predictors in the model. Adding irrelevant predictors can artificially inflate R-squared, but the adjusted R-squared accounts for this, providing a more conservative measure of model fit. This is particularly important when comparing models with different numbers of predictors.
6. F-test assesses the overall significance of the regression model.
TRUE. The F-test evaluates the null hypothesis that all regression coefficients are simultaneously equal to zero. A significant F-test indicates that at least one independent variable significantly predicts the dependent variable. It doesn't, however, pinpoint which specific variables are significant.
7. t-tests assess the significance of individual predictors.
TRUE. Individual t-tests for each regression coefficient determine whether each independent variable significantly contributes to the model, holding other variables constant. These tests help identify the unique predictive power of each predictor.
8. Residual analysis is crucial for assessing model assumptions.
TRUE. Examining the residuals (the differences between observed and predicted values) is vital for diagnosing potential violations of assumptions like linearity, homoscedasticity, and normality. Scatterplots, histograms, and other diagnostic plots help assess the residuals’ distribution and identify patterns that suggest problems.
9. Multicollinearity can inflate standard errors and make it difficult to interpret individual predictor effects.
TRUE. High correlation between independent variables (multicollinearity) can lead to unstable and unreliable estimates of regression coefficients, making it challenging to determine the unique contribution of each predictor. This can result in inflated standard errors and consequently, insignificant p-values even if the predictors are truly influential.
10. Outliers can significantly influence regression results.
TRUE. Outliers, data points that deviate substantially from the general pattern, can exert disproportionate influence on the regression line and its parameters. Identifying and handling outliers (e.g., through transformation or removal) is crucial for obtaining robust and reliable results. However, the decision to remove an outlier must be justified and should not be solely based on its influence on the model.
False Statements About Multiple Regression Analyses
1. A high R-squared always indicates a good model.
FALSE. While a high R-squared suggests a good fit, it doesn't guarantee the model's validity or usefulness. Overfitting, where the model fits the sample data too closely but generalizes poorly to new data, can lead to a high R-squared despite poor predictive accuracy.
2. Including more predictors always improves the model.
FALSE. Adding irrelevant predictors can increase R-squared but decrease the adjusted R-squared and harm the model's predictive ability. Model selection techniques, such as stepwise regression or regularization methods (e.g., Lasso, Ridge), are used to identify the optimal subset of predictors.
3. Correlation implies causation.
FALSE. Multiple regression models only reveal associations between variables. A significant relationship between an independent and dependent variable does not necessarily imply a causal link. Other factors or confounding variables may be responsible for the observed association.
4. Multiple regression is only suitable for large datasets.
FALSE. Although larger datasets generally provide more stable estimates, multiple regression can be applied to datasets of various sizes. However, the reliability of the results may be affected by the sample size, particularly if the number of predictors is high relative to the number of observations.
5. Violation of assumptions always renders the results useless.
FALSE. While violations of assumptions can affect the validity of inferences, the severity of the impact depends on the nature and extent of the violation. In some cases, transformations of variables or robust regression techniques can mitigate the effects of assumption violations.
6. Multiple regression can handle categorical predictors without any modifications.
FALSE. Standard multiple regression requires numerical independent variables. Categorical predictors need to be converted into numerical representations using techniques like dummy coding or effect coding before they can be included in the model.
7. The order of predictors in the model doesn't matter.
FALSE. In hierarchical regression, the order in which predictors are entered into the model affects the interpretation of the results. The unique contribution of a predictor is determined only after controlling for predictors entered earlier in the model.
8. Interaction effects are automatically included in standard multiple regression.
FALSE. Standard multiple regression models only consider the main effects of individual predictors. To assess the interaction between predictors (how the effect of one predictor depends on the level of another), interaction terms (product of predictors) must be explicitly included in the model.
9. You only need to check for multicollinearity amongst predictors.
FALSE. While multicollinearity among independent variables is a major concern, it’s also important to assess the correlation between dependent and independent variables. A lack of substantial correlation between the outcome variable and at least one predictor suggests the model may not be appropriate.
10. Interpreting regression coefficients is always straightforward.
FALSE. Interpreting regression coefficients requires careful consideration of the model's context and the scales of the variables. For example, the interpretation of coefficients differs when using standardized versus unstandardized values. Additionally, interactions and non-linear relationships can complicate the interpretation of coefficients.
Conclusion
Multiple regression analysis is a valuable tool for exploring relationships among variables, but its proper application requires a thorough understanding of its assumptions, strengths, and limitations. Carefully evaluating the statements and explanations provided here will help researchers and practitioners avoid common pitfalls and ensure the responsible and accurate use of this powerful statistical technique. By understanding both the true and false statements concerning multiple regression, researchers can build more robust and reliable models, leading to better insights and more informed decision-making. Always remember to critically evaluate your results and consider the limitations of your analysis. The goal is not just to find a model that fits the data well, but to build a model that accurately reflects the underlying relationships and can generalize to new data effectively.
Latest Posts
Latest Posts
-
Chapter 5 Auto Shop Safety Answer Key
Apr 01, 2025
-
Most Hammer Drills Will Not Hammer Until Pressure Is
Apr 01, 2025
-
Which Of The Following Products Helps Prevent Nails From Splitting
Apr 01, 2025
-
Murder Mystery In The Pickelson Mansion Answer Key
Apr 01, 2025
-
Themes In Chronicle Of A Death Foretold
Apr 01, 2025
Related Post
Thank you for visiting our website which covers about Identify The True And False Statements About Multiple-regression Analyses. . We hope the information provided has been useful to you. Feel free to contact us if you have any questions or need further assistance. See you next time and don't miss to bookmark.