John Wants To Study Whether A Larger
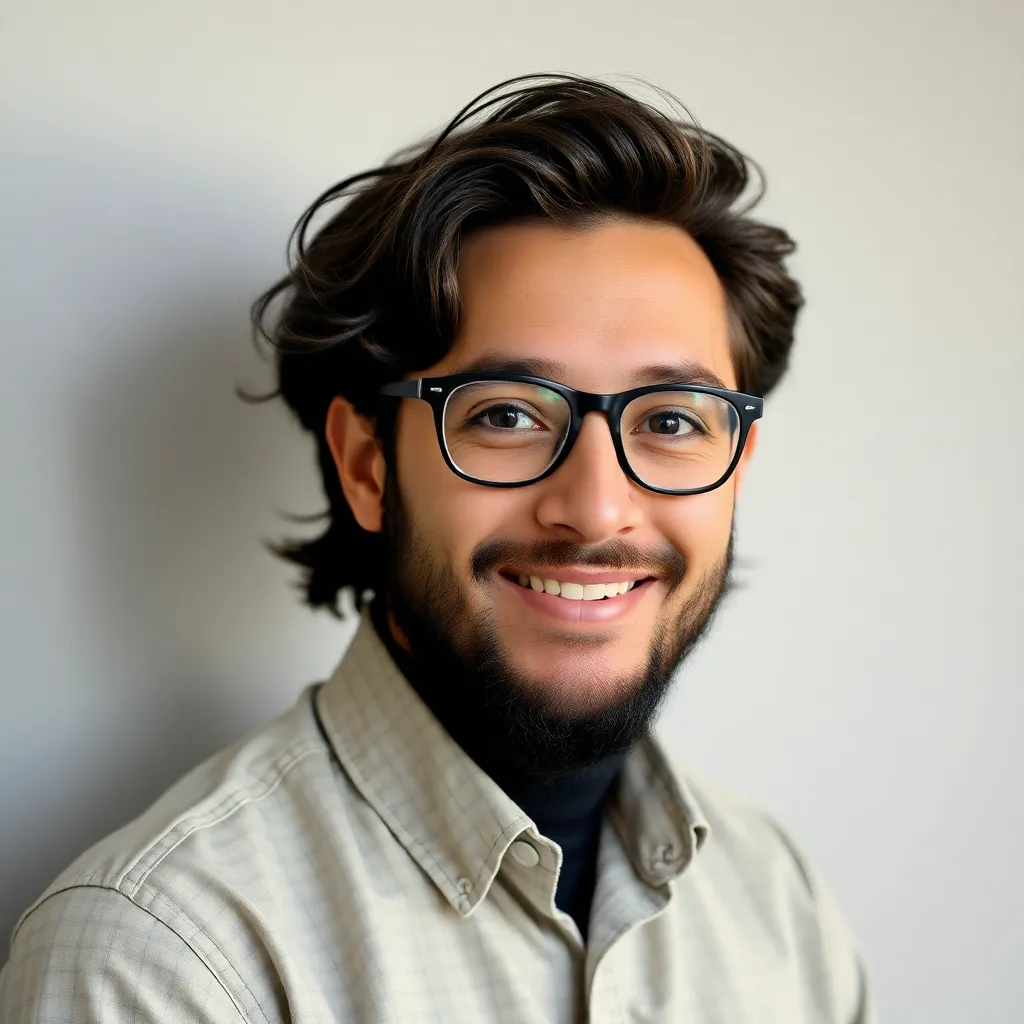
Onlines
May 10, 2025 · 6 min read
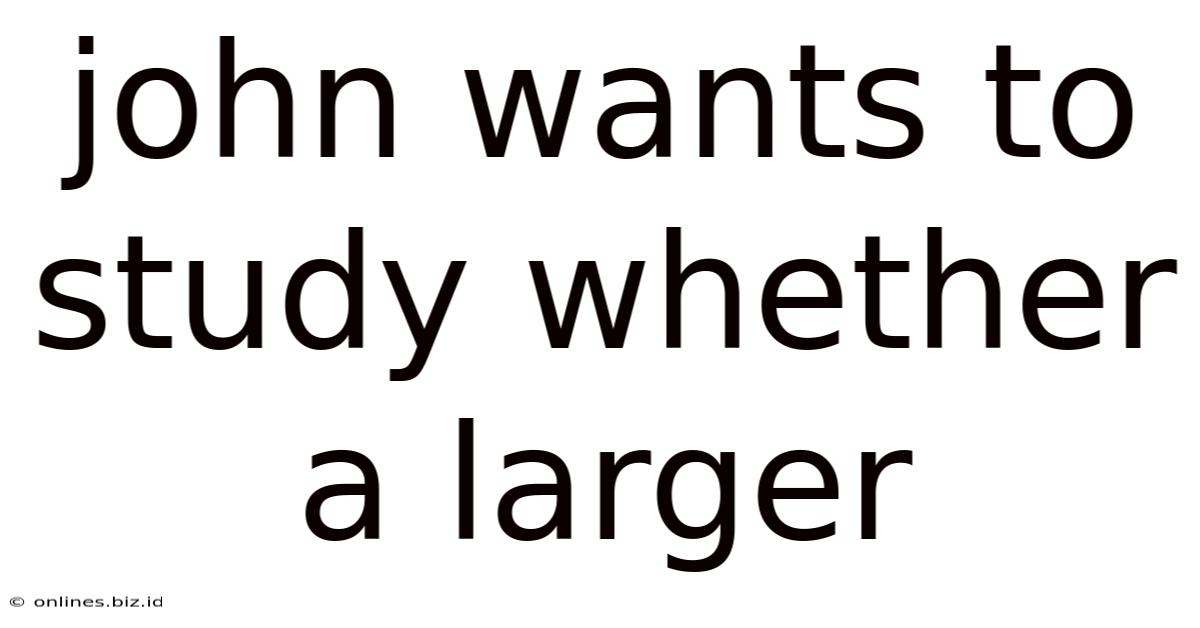
Table of Contents
John Wants to Study Whether a Larger Sample Size Improves Accuracy in Estimating Population Mean: A Deep Dive into Statistical Significance
John, a diligent statistics student, is embarking on a fascinating research project: exploring the relationship between sample size and the accuracy of estimating a population mean. This is a cornerstone concept in inferential statistics, with profound implications across diverse fields. This article will delve into John's research question, outlining the methodology, potential challenges, and broader interpretations of his findings. We'll also explore the crucial role of sample size in achieving statistical significance and generating reliable conclusions.
Understanding the Central Limit Theorem and its Relevance
Before we dive into John's specific research, let's establish a foundational understanding. The Central Limit Theorem (CLT) is paramount. It states that the distribution of sample means, regardless of the shape of the population distribution, will approximate a normal distribution as the sample size increases. This is true provided the sample size is sufficiently large (generally considered to be at least 30). The larger the sample size, the closer the sampling distribution of the mean will resemble a normal distribution. This is crucial because many statistical tests rely on the assumption of normality.
The Implications of the CLT for John's Research
The CLT directly informs John's research. He hypothesizes that a larger sample size will lead to a more accurate estimate of the population mean. This accuracy is reflected in a smaller standard error of the mean (SEM). The SEM quantifies the variability of sample means around the true population mean. A smaller SEM indicates that the sample means are clustered more tightly around the true population mean, suggesting a more precise estimate. John's research will empirically test this relationship.
John's Research Methodology: A Step-by-Step Approach
John's research will follow a structured approach, encompassing several key steps:
1. Defining the Population and Parameter of Interest
First, John must clearly define the population he's studying. This could be anything from the height of adult women in a specific country to the average income of households in a particular city. The parameter of interest is the population mean (µ), which is the true average value of the variable in the entire population.
2. Selecting Sampling Techniques and Sample Sizes
John will employ various sampling techniques to collect data. Random sampling is crucial to ensure that his sample is representative of the population and minimizes bias. He'll compare the results from different sample sizes. For instance, he might draw samples of size 30, 100, 500, and 1000 from the same population. Larger sample sizes generally lead to better representation, but they are also more expensive and time-consuming to collect.
3. Data Collection and Analysis
Once the samples are drawn, John will collect the data for his variable of interest. After data collection, he’ll employ descriptive statistics to summarize the data for each sample size. This includes calculating the sample mean (x̄) and the sample standard deviation (s). Crucially, he’ll calculate the standard error of the mean (SEM) for each sample, using the formula: SEM = s / √n, where 'n' is the sample size.
4. Comparing Results Across Different Sample Sizes
John will compare the SEM across different sample sizes. He expects to observe a pattern: as the sample size ('n') increases, the SEM will decrease. This decrease signifies a reduction in the variability of sample means, indicating a more precise estimate of the population mean. He can visually represent this using graphs and tables showing the relationship between sample size and SEM. This visual representation will aid in communicating his findings effectively.
5. Statistical Inference and Hypothesis Testing
To provide stronger evidence, John will use hypothesis testing. He can formulate a null hypothesis (H0) stating that there is no difference in the accuracy of estimating the population mean between different sample sizes. The alternative hypothesis (H1) would state that a larger sample size leads to a more accurate estimate (smaller SEM). He might use ANOVA or a t-test to compare the means and variances of the SEMs across different sample sizes. The p-value obtained from these tests will determine whether he can reject the null hypothesis.
Potential Challenges and Considerations
John's research, while straightforward in its conception, may encounter several challenges:
1. Obtaining Representative Samples
Achieving truly representative samples can be difficult. Bias can creep in through various sampling methods. Careful consideration of sampling techniques and potential sources of bias is crucial.
2. Cost and Time Constraints
Larger sample sizes require more resources. Balancing the need for a large enough sample to achieve statistical power with the practical constraints of time and budget is important.
3. Data Availability and Quality
Accessing sufficient data for analysis can be challenging depending on the population and variable of interest. Data quality is also paramount. Inaccurate or incomplete data can significantly skew the results and compromise the validity of the study.
Broader Implications and Applications of John's Research
John's research holds considerable significance across various fields:
1. Market Research and Business Decisions
Businesses rely heavily on sampling to understand customer preferences and market trends. John’s findings underscore the importance of appropriately sized samples for accurate market research that can inform strategic business decisions.
2. Public Health and Epidemiology
Epidemiological studies frequently rely on sampling to track disease prevalence and understand health outcomes. Accurate sampling is essential for effective public health interventions. John's research helps to refine sampling strategies and improve the reliability of epidemiological findings.
3. Social Sciences and Political Science
Research in these fields often involves surveying populations to gather opinions and understand social phenomena. John's work highlights the critical role of sample size in obtaining statistically meaningful results that can inform policy decisions.
4. Environmental Science and Ecology
Monitoring environmental changes and studying ecological systems often involve sampling techniques. Accurate estimation of parameters like pollution levels or species populations requires careful attention to sample size.
Conclusion: The Power of Sample Size in Statistical Inference
John's research into the relationship between sample size and the accuracy of estimating population means is a fundamental undertaking in statistics. His findings will empirically demonstrate the crucial role of sample size in reducing the standard error of the mean, leading to more precise estimates and greater confidence in statistical inferences. The implications of his research extend far beyond the academic realm, affecting diverse fields that rely on sampling for data collection and decision-making. By understanding and applying the principles of statistical sampling, researchers and practitioners alike can improve the accuracy and reliability of their findings, ultimately leading to better-informed decisions and more effective interventions. John's work serves as a reminder of the foundational importance of sound statistical methodology in any quantitative research endeavor. The larger the sample size, the smaller the standard error, and the greater the confidence in the accuracy of the estimate of the population mean. This principle underpins much of statistical inference and highlights the significance of careful planning and execution of sampling procedures in various fields of study.
Latest Posts
Latest Posts
-
A Pressure Regulator Valve Ensures That
May 10, 2025
-
Which Statement Must Be True About The Diagram
May 10, 2025
-
A Scientist Studied The Formation Of The Protein Hemoglobin
May 10, 2025
-
According To Isaiah 53 Which Of The Following
May 10, 2025
-
Alexanders Ragtime Band Is An Example Of
May 10, 2025
Related Post
Thank you for visiting our website which covers about John Wants To Study Whether A Larger . We hope the information provided has been useful to you. Feel free to contact us if you have any questions or need further assistance. See you next time and don't miss to bookmark.