Some Quantitative Data Sets Do Not Have Medians
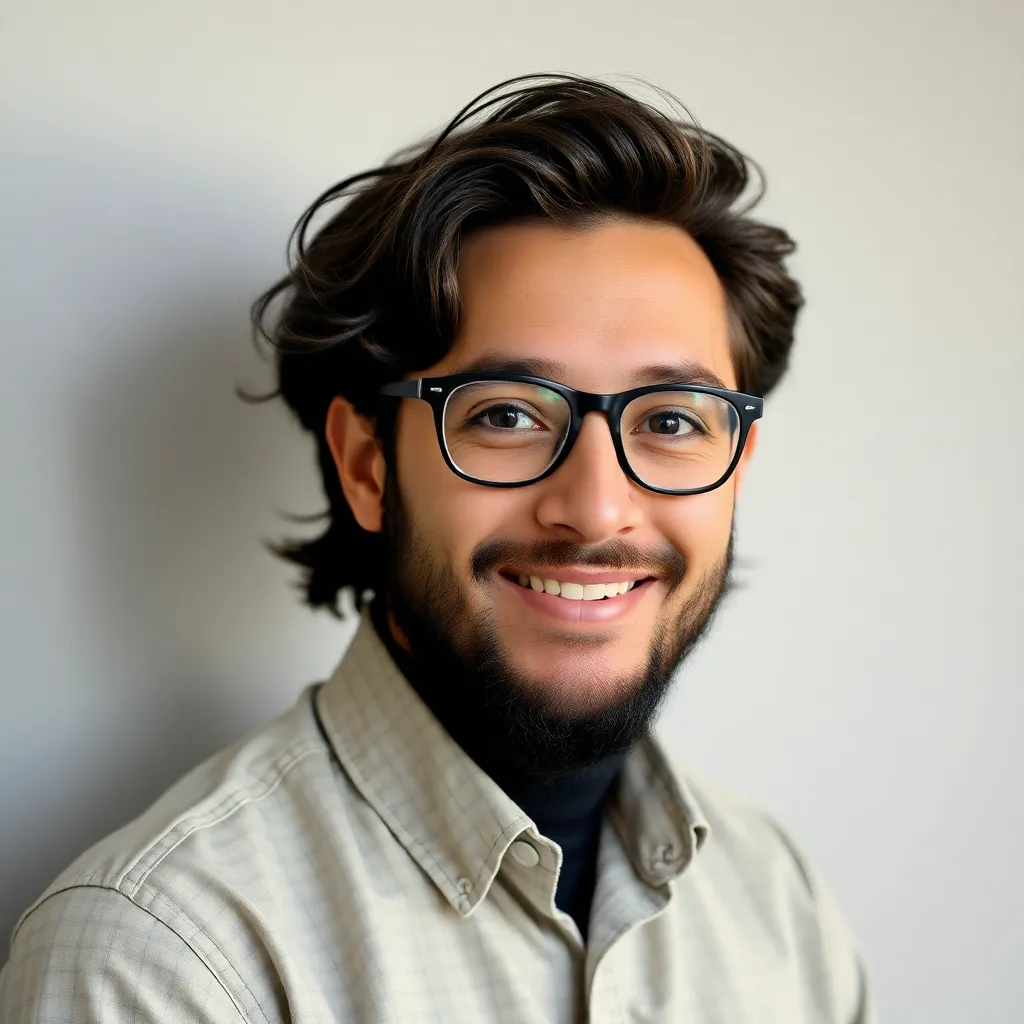
Onlines
May 08, 2025 · 6 min read
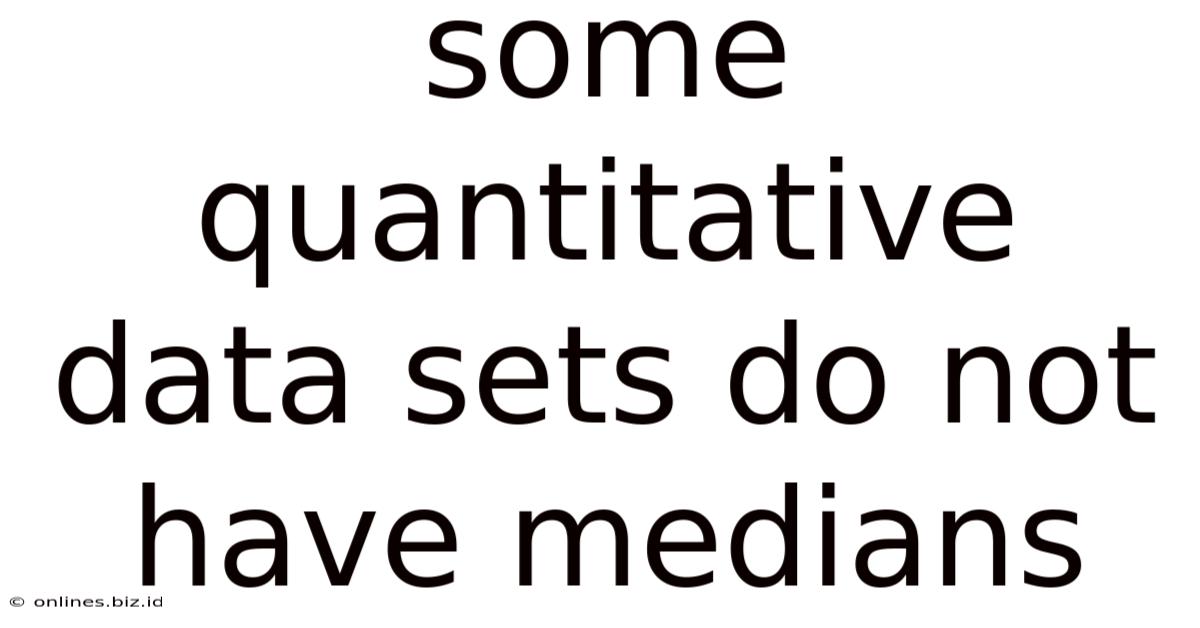
Table of Contents
Some Quantitative Data Sets Do Not Have Medians
The median, a fundamental measure of central tendency, represents the middle value in an ordered data set. Its simplicity and robustness to outliers make it a popular choice in descriptive statistics. However, a crucial aspect often overlooked is that not all quantitative data sets possess a well-defined median. This seemingly counterintuitive fact stems from the very definition of the median and its reliance on the ability to order data points. Understanding the conditions under which a median fails to exist is crucial for data analysts, researchers, and anyone working with numerical data. This article delves into the circumstances where medians are undefined, explores alternative measures of central tendency in such cases, and provides practical examples to illustrate the concept.
When the Median Fails to Exist: Understanding the Limitations
The median is defined as the middle value when a data set is arranged in ascending or descending order. For a data set with an odd number of observations, the median is the single middle value. For a data set with an even number of observations, the median is the average of the two middle values. This definition implicitly assumes that the data points can be meaningfully ordered. The inability to order data points leads to situations where the median is undefined. This typically occurs in the following scenarios:
1. Non-Numerical Data: The Absence of Order
The most common reason for an undefined median is the presence of non-numerical data. Consider a data set representing the colors of cars in a parking lot: {red, blue, green, red, blue}. These categorical values lack inherent numerical order. You cannot definitively say "red" is greater or less than "blue". Therefore, arranging the data in ascending or descending order is impossible, and thus, calculating a median is meaningless.
2. Circular or Cyclic Data: Ambiguity in Ordering
Another situation where ordering becomes problematic involves circular or cyclic data. This type of data often represents angles, times of day, or positions on a circle. Imagine measuring the direction of wind in degrees (0 to 360). A wind direction of 350 degrees is not inherently "smaller" than 10 degrees, despite 10 being numerically smaller than 350. Ordering such data requires arbitrary choices, rendering the median calculation ambiguous and unreliable. The median is not well-defined for this kind of data unless specific conventions are adopted to linearize the circular representation.
3. Data with Open-Ended Intervals: Missing Boundary Information
Data sets containing open-ended intervals also present difficulties. Consider income data with categories like "<$25,000," "$25,000-$50,000," and "$50,000+". The final category lacks an upper bound, preventing a precise ordering of all data points. The median cannot be reliably calculated without specifying the upper limit of the open-ended interval. While approximations are possible using assumed boundaries, the precision of the median estimate suffers considerably.
4. Missing Values: Incomplete Data Sets
Although not strictly a failure of the median concept, missing data can significantly impact the calculation. Missing values disrupt the ordering process, requiring imputation or omission. While imputation techniques (replacing missing values with estimates) can allow for median calculation, the result's accuracy depends heavily on the imputation method's quality. Omitting data with missing values can lead to biased results if the missingness is not completely random.
Alternative Measures of Central Tendency
When the median is undefined, alternative measures of central tendency become necessary. The choice of alternative depends on the nature of the data and the research objectives:
1. Mode: For Categorical and Multimodal Data
The mode, the most frequent value in a data set, is suitable for categorical data and even numerical data with multiple peaks (multimodal data). The mode is not affected by the lack of order and remains definable even when the median is not. However, the mode can be ambiguous, particularly if multiple values have the same highest frequency.
2. Mean (Average): When Data Ordering is Not Critical
The mean (arithmetic average) is another common measure of central tendency. It's calculated by summing all values and dividing by the number of values. While the mean is sensitive to outliers and requires numerical data, it doesn't require explicit ordering of data points. However, the mean might not be representative if the data distribution is heavily skewed or contains extreme values.
3. Geometric Mean: For Data with Multiplicative Relationships
The geometric mean, calculated as the nth root of the product of n values, is applicable for data representing ratios or multiplicative relationships (e.g., growth rates). The geometric mean is less sensitive to outliers than the arithmetic mean and is useful when dealing with data values that span wide ranges.
4. Robust Alternatives: Winsorized and Trimmed Means
For numerical data with outliers, robust alternatives like the Winsorized mean and the trimmed mean offer resilience to extreme values. These methods involve replacing or removing extreme values before calculating the mean, reducing the influence of outliers on the measure of central tendency. The choice of Winsorization or trimming parameters impacts the final result, requiring careful consideration.
Illustrative Examples
Let's illustrate the challenges with concrete examples:
Example 1: Non-numerical data
Data set: {apple, banana, apple, orange, banana}
Median: Undefined. There is no inherent order to the fruits. The mode is 'apple' and 'banana'.
Example 2: Circular data
Data set: {350 degrees, 10 degrees, 170 degrees, 300 degrees, 20 degrees}
Median: Ambiguous. The median's value depends on how you order the angles. If you treat them linearly, the median would be 170 degrees. But this is misleading considering their cyclic nature.
Example 3: Open-ended intervals
Data set: {<$20,000, $20,000-$40,000, $40,000-$60,000, >$60,000}
Median: Undefined without specific upper and lower limits for each interval. The median's calculation requires making assumptions about the boundaries of these open-ended intervals.
Example 4: Missing values
Data set: {10, 15, NA, 25, 30}
Median: The median cannot be calculated directly. Either imputation is needed to fill the NA value or the NA value must be omitted. Either option will influence the result.
Conclusion
The median, while a valuable measure of central tendency, is not universally applicable. Understanding the situations where it's undefined is vital for accurate data analysis. The absence of a well-defined median isn't a flaw in the median itself; rather, it highlights the importance of understanding the data's characteristics before choosing appropriate statistical methods. When confronted with data sets lacking inherent order or containing open-ended intervals or missing values, alternative measures such as the mode, mean, geometric mean, or robust alternatives to the mean become necessary. The selection of the most appropriate measure depends on the specific context, the nature of the data, and the analytical goals. The key takeaway is that a thoughtful assessment of data properties precedes any statistical analysis, ensuring accurate and meaningful interpretations.
Latest Posts
Latest Posts
-
A Type Ambulance Features A Conventional Truck Cab Chassis
May 08, 2025
-
Activity 1 1 5 Gears Pulley Drives And Sprockets Practice Problems
May 08, 2025
-
The Usual Ordering Of Accounts In The General Ledger Is
May 08, 2025
-
Estimation Is Important For Which Capabilities And Value Proposition
May 08, 2025
-
Aegean Artists Created Exquisite Luxury Goods From Imported
May 08, 2025
Related Post
Thank you for visiting our website which covers about Some Quantitative Data Sets Do Not Have Medians . We hope the information provided has been useful to you. Feel free to contact us if you have any questions or need further assistance. See you next time and don't miss to bookmark.