Statistics For Business And Economics Chapter 7 Solutions
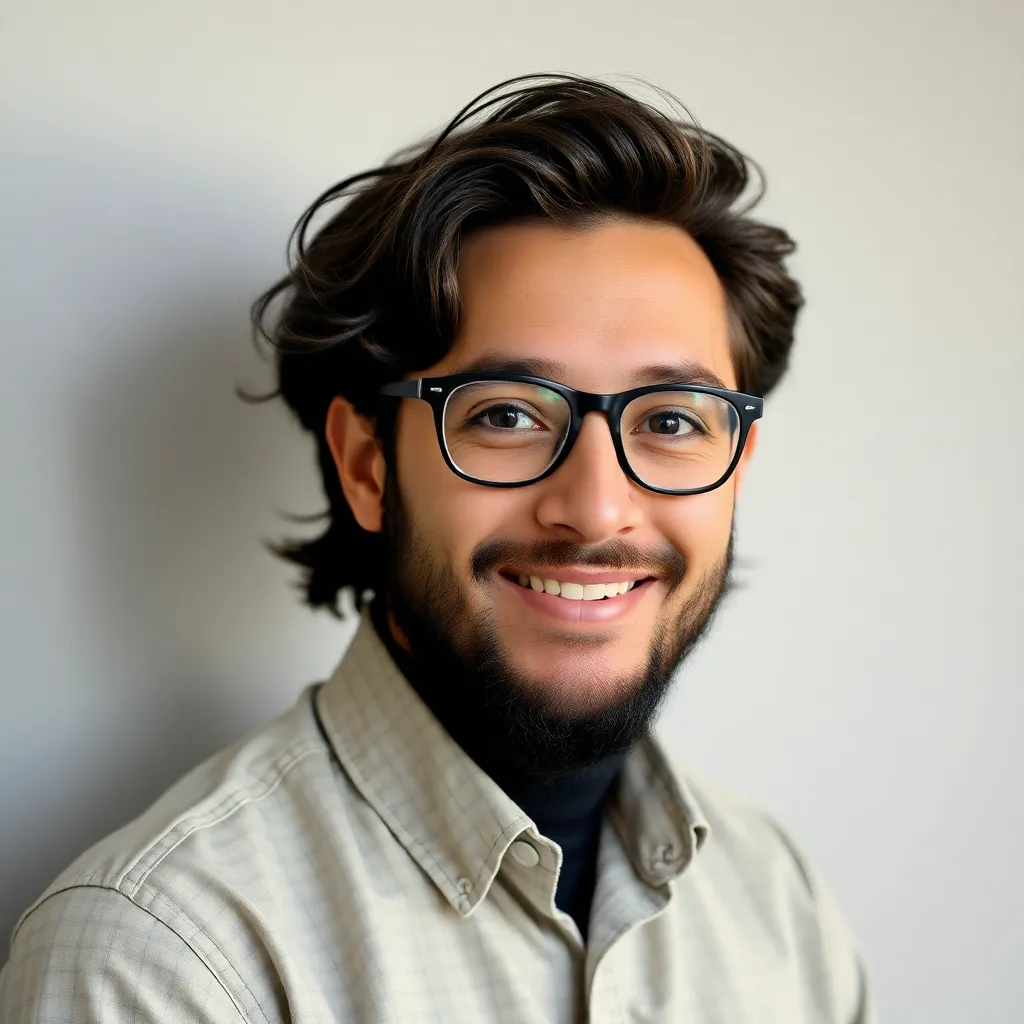
Onlines
Apr 07, 2025 · 6 min read
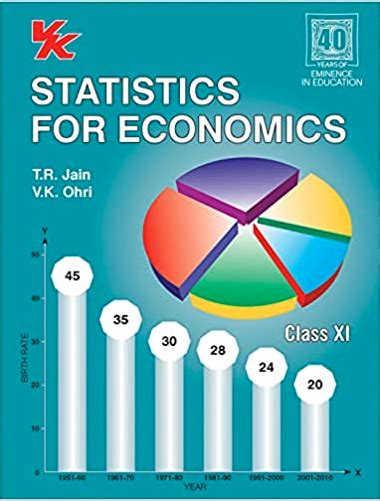
Table of Contents
Statistics for Business and Economics: Chapter 7 Solutions – A Deep Dive
Chapter 7 of most introductory statistics textbooks for business and economics typically covers hypothesis testing. This crucial chapter forms the bedrock of inferential statistics, allowing us to draw conclusions about populations based on sample data. This comprehensive guide will dissect the core concepts of Chapter 7, providing solutions and explanations to common problems encountered in this area. We will explore various hypothesis tests, focusing on understanding the underlying logic and practical applications.
Understanding Hypothesis Testing: The Foundation
Before delving into specific problems, let's solidify our understanding of the fundamental principles. Hypothesis testing involves formulating a null hypothesis (H₀) and an alternative hypothesis (H₁ or Hₐ). The null hypothesis represents the status quo, a claim we are trying to disprove. The alternative hypothesis is what we believe to be true if we reject the null hypothesis.
The process typically involves these steps:
- State the hypotheses: Clearly define H₀ and H₁.
- Set the significance level (α): This represents the probability of rejecting the null hypothesis when it is actually true (Type I error). Common values are 0.05 and 0.01.
- Choose the appropriate test statistic: This depends on the type of data (e.g., z-test, t-test, chi-square test).
- Determine the critical value or p-value: The critical value marks the boundary for rejecting H₀. The p-value represents the probability of obtaining the observed results if H₀ is true.
- Make a decision: Compare the test statistic to the critical value or the p-value to α. Reject H₀ if the test statistic falls in the rejection region or if the p-value is less than α.
- Interpret the results: Clearly state the conclusion in the context of the problem.
Types of Hypothesis Tests Covered in Chapter 7
Chapter 7 usually covers several types of hypothesis tests, including:
- One-sample z-test: Used to test a hypothesis about a population mean when the population standard deviation is known.
- One-sample t-test: Used to test a hypothesis about a population mean when the population standard deviation is unknown.
- Two-sample t-test: Used to compare the means of two independent populations.
- Paired t-test: Used to compare the means of two related populations (e.g., before and after measurements).
- One-sample proportion z-test: Used to test a hypothesis about a population proportion.
- Two-sample proportion z-test: Used to compare two population proportions.
Solved Examples and Explanations
Let's work through some typical Chapter 7 problems, focusing on understanding the process and interpreting the results. We'll use hypothetical examples for clarity and to avoid specific textbook questions that may be copyrighted.
Example 1: One-Sample t-test
Problem: A company claims that its new light bulb has an average lifespan of 1000 hours. A sample of 50 bulbs is tested, yielding a sample mean of 980 hours and a sample standard deviation of 80 hours. Test the company's claim at a 5% significance level.
Solution:
-
Hypotheses:
- H₀: μ = 1000 (The average lifespan is 1000 hours)
- H₁: μ ≠ 1000 (The average lifespan is different from 1000 hours) This is a two-tailed test.
-
Significance Level: α = 0.05
-
Test Statistic: One-sample t-test, since the population standard deviation is unknown.
-
Calculations:
- t = (sample mean - population mean) / (sample standard deviation / √sample size)
- t = (980 - 1000) / (80 / √50) ≈ -1.77
-
Critical Value or p-value: Using a t-distribution table with 49 degrees of freedom (df = n - 1), the critical values for a two-tailed test at α = 0.05 are approximately ±2.01. Alternatively, using statistical software, we find a p-value of approximately 0.08.
-
Decision: Since the calculated t-value (-1.77) is not less than -2.01 and the p-value (0.08) is greater than α (0.05), we fail to reject the null hypothesis.
-
Interpretation: There is not enough evidence at the 5% significance level to reject the company's claim that the average lifespan of the light bulbs is 1000 hours.
Example 2: Two-Sample t-test
Problem: Two different teaching methods are being compared. A sample of 25 students using Method A achieves an average test score of 85 with a standard deviation of 10. A sample of 30 students using Method B achieves an average test score of 88 with a standard deviation of 12. Is there a significant difference in the average test scores between the two methods at a 1% significance level?
Solution: This requires a two-sample t-test assuming unequal variances (since the sample standard deviations differ significantly). The steps would be similar to the previous example, but the calculation of the t-statistic and degrees of freedom would be adjusted accordingly. Statistical software is highly recommended for these calculations.
Example 3: Chi-Square Test
While often covered in a later chapter, the basics of chi-square tests might be introduced in chapter 7.
Problem: A company wants to determine if there's a relationship between customer age group and their preference for a certain product. They gather data and want to perform a chi-square test for independence.
Solution: A contingency table would be created summarizing the observed frequencies of each age group and product preference. The chi-square statistic would then be calculated, comparing the observed frequencies to the expected frequencies under the assumption of independence. The p-value is then compared to the significance level to determine whether to reject the null hypothesis (that there's no relationship between age and product preference).
Advanced Concepts and Further Exploration
Chapter 7 often touches upon more advanced topics, laying the groundwork for subsequent chapters. These may include:
- Type I and Type II errors: Understanding the trade-off between these errors and the concept of power.
- Confidence intervals: Connecting hypothesis testing to confidence intervals, showing how they provide additional information about the estimated population parameter.
- Effect size: Measuring the magnitude of the difference between groups, independent of sample size.
- Assumptions of hypothesis tests: Understanding the conditions that need to be met for the tests to be valid (e.g., normality, independence).
Conclusion: Mastering Hypothesis Testing
Mastering Chapter 7 is critical for anyone studying business and economics. A solid understanding of hypothesis testing empowers you to analyze data effectively, draw meaningful conclusions, and make informed decisions based on evidence. Remember that the key lies not just in performing the calculations but also in correctly interpreting the results within the context of the problem. Statistical software significantly aids in the calculation process, allowing you to focus on the interpretation and application of the results in real-world scenarios. Consistent practice and a thorough understanding of the underlying principles are essential for success. Don't hesitate to seek further resources and clarification to strengthen your grasp on these vital statistical concepts.
Latest Posts
Latest Posts
-
Financial Analysis With Microsoft Excel 9th Edition
Apr 07, 2025
-
What Complaint Do Defense Lawyers Have About Csi Type Shows
Apr 07, 2025
-
Which Scenario Describes The Highest Level Of Productivity
Apr 07, 2025
-
In Hair Design The Term Texture Character Refers To The
Apr 07, 2025
-
7 8 Exponential Models With Differential Equations
Apr 07, 2025
Related Post
Thank you for visiting our website which covers about Statistics For Business And Economics Chapter 7 Solutions . We hope the information provided has been useful to you. Feel free to contact us if you have any questions or need further assistance. See you next time and don't miss to bookmark.