Unit 4 Progress Check Mcq Part A Ap Stats
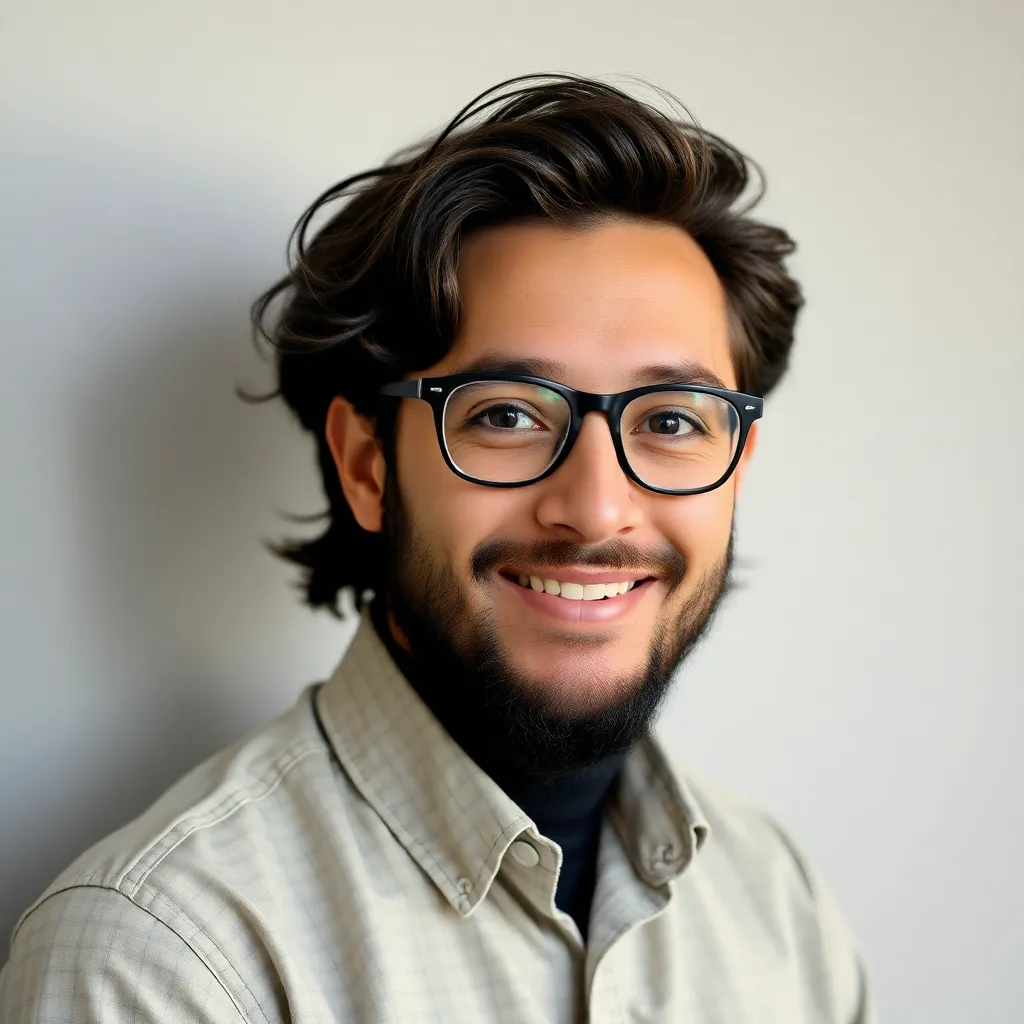
Onlines
Apr 24, 2025 · 7 min read

Table of Contents
Unit 4 Progress Check: MCQ Part A - AP Stats: A Comprehensive Guide
The AP Statistics Unit 4 Progress Check, Part A, covers a significant portion of the course material, focusing primarily on sampling distributions and inference for proportions. This guide provides a thorough review of the key concepts, common question types, and strategies for mastering this crucial section of your AP Statistics journey. We’ll break down the core ideas, practice with example problems, and offer tips to enhance your understanding and performance.
Understanding Sampling Distributions
The cornerstone of Unit 4 is the concept of the sampling distribution. This refers to the distribution of a statistic (like a sample proportion or sample mean) from all possible samples of a given size from a population. Understanding its characteristics – center, shape, and spread – is vital for making inferences about the population.
Center of the Sampling Distribution
The center of a sampling distribution for a sample proportion (p̂) is typically centered around the population proportion (p). This means that if you were to take many samples and calculate the sample proportion for each, the average of those sample proportions would be very close to the true population proportion. This is a key principle for understanding unbiased estimators.
Shape of the Sampling Distribution
The shape of the sampling distribution is often approximately normal, especially when the sample size is large enough. This is a consequence of the Central Limit Theorem (CLT), which states that the sampling distribution of the sample mean (or sample proportion) will be approximately normal if the sample size is sufficiently large, regardless of the shape of the population distribution. A common rule of thumb is to check the conditions: np ≥ 10
and n(1-p) ≥ 10
. If these conditions are met, the normal approximation is usually valid. However, remember that the CLT works best with larger sample sizes.
Spread (Standard Deviation) of the Sampling Distribution
The spread of the sampling distribution, often referred to as the standard error, is a measure of how much the sample proportions (or sample means) vary from sample to sample. The standard error for a sample proportion is given by the formula:
Standard Error (SE) = √[p(1-p)/n]
where 'p' is the population proportion and 'n' is the sample size. Note that a larger sample size leads to a smaller standard error, indicating less variability in the sample proportions.
Inference for Proportions: Confidence Intervals and Hypothesis Tests
Unit 4 heavily emphasizes inference for proportions, using both confidence intervals and hypothesis tests to draw conclusions about population proportions based on sample data.
Confidence Intervals for Proportions
A confidence interval provides a range of plausible values for the population proportion. The formula for a confidence interval for a population proportion is:
p̂ ± z√[p̂(1-p̂)/n]*
where:
- p̂ is the sample proportion
- z* is the critical z-value corresponding to the desired confidence level (e.g., 1.96 for a 95% confidence interval)
- n is the sample size
The interpretation of a confidence interval is crucial. A 95% confidence interval, for instance, means that if we were to repeatedly take samples and construct confidence intervals in this manner, approximately 95% of those intervals would contain the true population proportion.
Hypothesis Tests for Proportions
Hypothesis testing involves using sample data to test a claim about a population proportion. This typically involves:
-
Stating the hypotheses: This involves defining a null hypothesis (H₀) and an alternative hypothesis (Hₐ). For example:
- H₀: p = p₀ (the null hypothesis states that the population proportion is equal to a specific value)
- Hₐ: p ≠ p₀ (the alternative hypothesis states that the population proportion is different from the specific value – this is a two-tailed test) Alternatively, you could have one-tailed tests (Hₐ: p > p₀ or Hₐ: p < p₀).
-
Checking conditions: This involves verifying that the sample is random, the sample size is large enough (np ≥ 10 and n(1-p) ≥ 10), and the observations are independent.
-
Calculating the test statistic: This usually involves calculating a z-statistic:
z = (p̂ - p₀) / √[p₀(1-p₀)/n]
-
Determining the p-value: The p-value is the probability of observing a sample proportion as extreme as (or more extreme than) the one obtained, assuming the null hypothesis is true. A small p-value (typically less than a significance level, such as 0.05) provides evidence against the null hypothesis.
-
Making a decision: Based on the p-value and the significance level, we either reject or fail to reject the null hypothesis.
Common Question Types in the AP Stats Unit 4 Progress Check: Part A MCQ
The multiple-choice questions in the Unit 4 Progress Check, Part A, often test your understanding of the following:
- Interpreting sampling distributions: Questions might ask you to identify the center, shape, or spread of a sampling distribution given a scenario.
- Calculating standard error: You'll need to be comfortable calculating the standard error of a sample proportion.
- Constructing confidence intervals: Expect questions that require you to construct and interpret confidence intervals for proportions.
- Conducting hypothesis tests: Questions will often involve conducting a hypothesis test for a proportion, including stating hypotheses, checking conditions, calculating the test statistic, finding the p-value, and making a conclusion.
- Understanding Type I and Type II errors: Questions might assess your understanding of the concepts of Type I and Type II errors in the context of hypothesis testing.
- Interpreting p-values: You need to be able to correctly interpret the meaning of a p-value in the context of a hypothesis test.
- Applying the Central Limit Theorem: Questions might test your understanding of when the Central Limit Theorem applies and its implications for the sampling distribution.
Example Problems and Solutions
Let's illustrate these concepts with some example problems:
Example 1: Confidence Interval
A random sample of 100 students from a large university reveals that 60% support a new campus policy. Construct a 95% confidence interval for the true proportion of students who support the policy.
Solution:
- p̂ = 0.60
- n = 100
- z* (for 95% confidence) = 1.96
Confidence interval = 0.60 ± 1.96√[(0.60)(0.40)/100] ≈ 0.60 ± 0.096 ≈ (0.504, 0.696)
We are 95% confident that the true proportion of students who support the policy lies between 50.4% and 69.6%.
Example 2: Hypothesis Test
A company claims that at least 80% of its customers are satisfied. A random sample of 200 customers reveals that 150 are satisfied. Test the company's claim at a significance level of 0.05.
Solution:
-
Hypotheses:
- H₀: p ≥ 0.80
- Hₐ: p < 0.80 (one-tailed test)
-
Conditions: Assuming the sample is random and observations are independent, we check:
- np₀ = 200(0.80) = 160 ≥ 10
- n(1-p₀) = 200(0.20) = 40 ≥ 10 Conditions are met.
-
Test statistic: p̂ = 150/200 = 0.75 z = (0.75 - 0.80) / √[(0.80)(0.20)/200] ≈ -2.236
-
P-value: Using a z-table or calculator, the p-value for a one-tailed test with z = -2.236 is approximately 0.0126.
-
Decision: Since the p-value (0.0126) is less than the significance level (0.05), we reject the null hypothesis. There is sufficient evidence to suggest that the company's claim is false; less than 80% of customers are satisfied.
Strategies for Success on the AP Statistics Unit 4 Progress Check: Part A
- Master the formulas: Understand and be able to apply the formulas for standard error, confidence intervals, and hypothesis tests for proportions.
- Practice, practice, practice: Work through numerous practice problems to build your understanding and identify areas where you need improvement. Use released AP Statistics exams and practice materials for additional practice.
- Understand the concepts: Don't just memorize formulas; understand the underlying concepts of sampling distributions, confidence intervals, and hypothesis tests.
- Check conditions: Always check the conditions for inference before conducting a hypothesis test or constructing a confidence interval.
- Interpret your results: Be able to correctly interpret the results of your calculations in the context of the problem.
- Review thoroughly: Ensure a comprehensive review of all relevant concepts before attempting the progress check.
By diligently reviewing these concepts, practicing with example problems, and utilizing effective study strategies, you can significantly improve your performance on the AP Statistics Unit 4 Progress Check, Part A, and enhance your overall understanding of statistical inference. Remember that consistent effort and a deep understanding of the underlying principles are key to success.
Latest Posts
Latest Posts
-
A Customer Enrolled In A 1 Year Product Purchase
Apr 24, 2025
-
Escoge Los Pronombres Correctos Para Completar Las Oraciones
Apr 24, 2025
-
Martha Is Considering Acquiring Another Piano
Apr 24, 2025
-
3 12 Unit Test Polynomials Part 1
Apr 24, 2025
-
Why Might Some Ecoregions Have More Species Than Others
Apr 24, 2025
Related Post
Thank you for visiting our website which covers about Unit 4 Progress Check Mcq Part A Ap Stats . We hope the information provided has been useful to you. Feel free to contact us if you have any questions or need further assistance. See you next time and don't miss to bookmark.