What Is The Best Conclusion Based On This Data
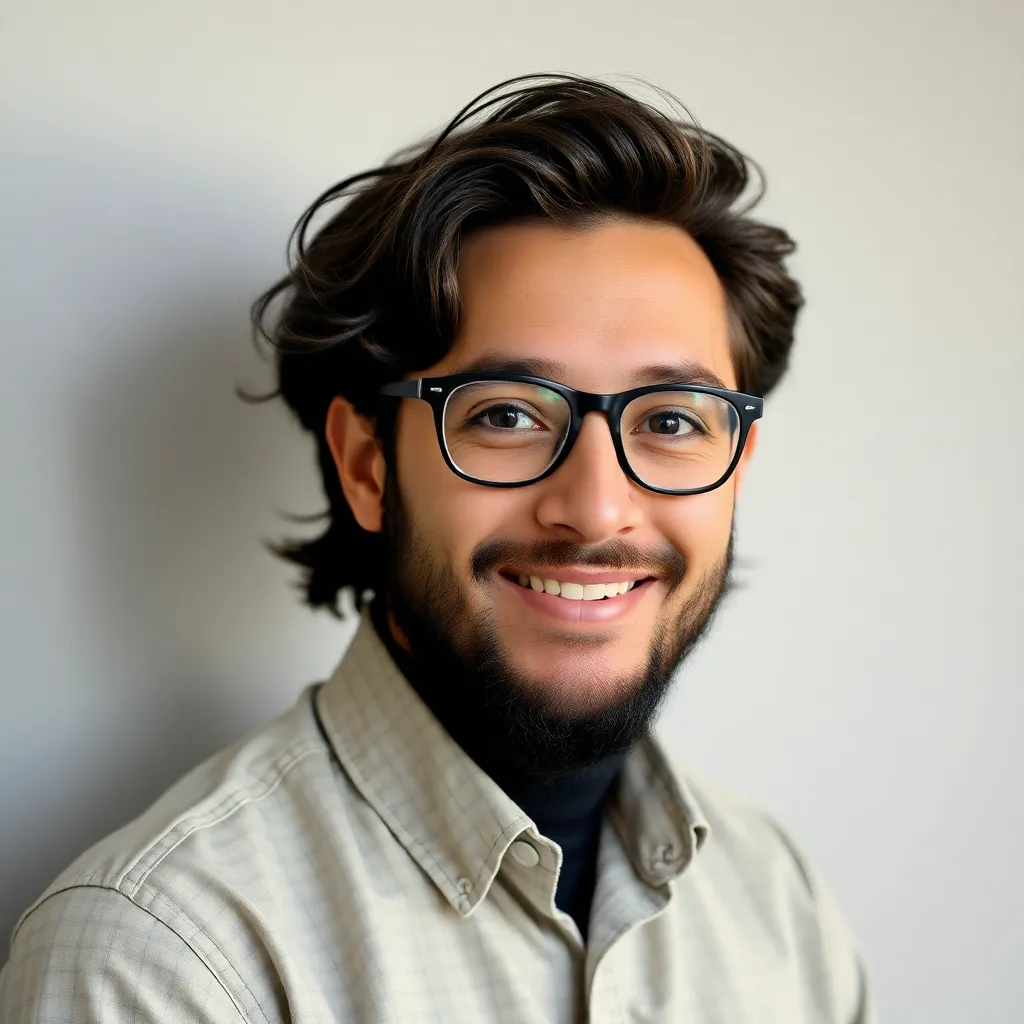
Onlines
May 12, 2025 · 7 min read
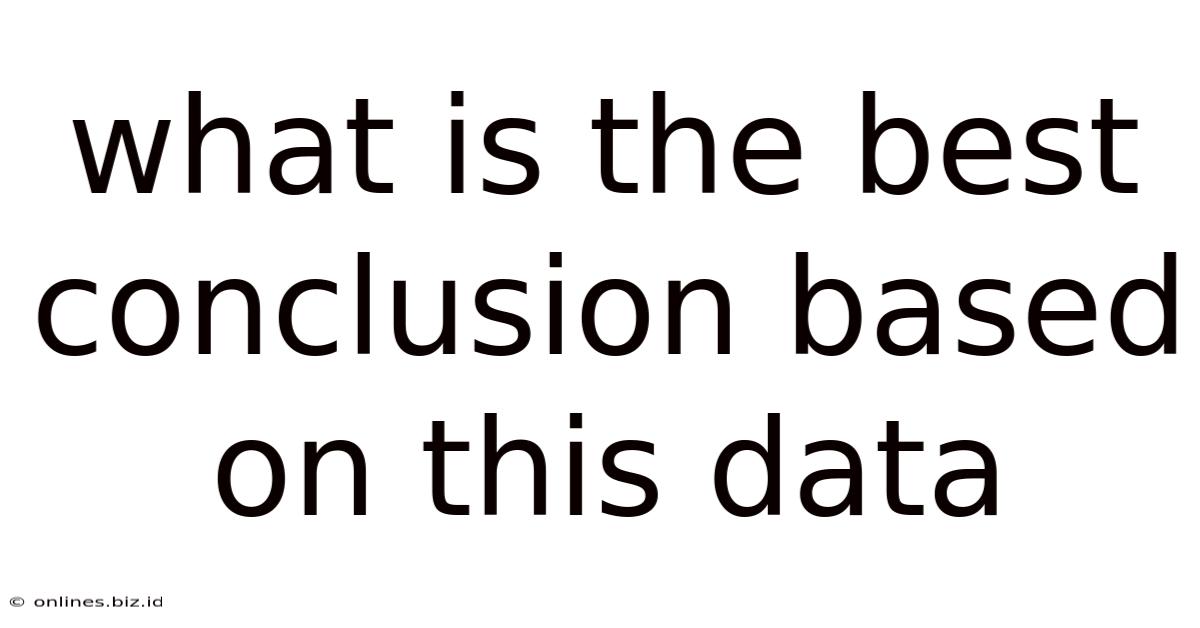
Table of Contents
What's the Best Conclusion Based on This Data? A Guide to Data Analysis and Interpretation
Drawing meaningful conclusions from data is the cornerstone of effective decision-making in any field. Whether you're analyzing market trends, evaluating the success of a marketing campaign, or researching the efficacy of a new drug, the ability to interpret data accurately and draw sound conclusions is paramount. This article will delve into the process of data analysis, exploring techniques to arrive at the best possible conclusions, emphasizing the importance of context, limitations, and the nuances of interpreting statistical significance.
Understanding Your Data: The Foundation of Sound Conclusions
Before we even begin to think about conclusions, a crucial first step is to thoroughly understand the data itself. This involves several key aspects:
1. Data Cleaning and Preparation:
Raw data is rarely perfect. It often contains inconsistencies, errors, and missing values. Data cleaning is the crucial process of identifying and addressing these issues. This might involve:
- Handling Missing Values: Deciding how to deal with missing data points is critical. Options include imputation (estimating missing values based on existing data), removal of incomplete records, or using specialized statistical techniques designed for incomplete datasets. The choice depends on the nature of the data and the extent of missingness.
- Identifying and Correcting Errors: Errors can range from simple typos to more significant data entry mistakes. Careful review and potentially automated error detection tools are invaluable here.
- Data Transformation: This involves converting data into a format suitable for analysis. This might include standardizing units, converting categorical variables into numerical representations (e.g., using dummy variables), or applying transformations to achieve normality (e.g., log transformations).
2. Exploratory Data Analysis (EDA):
Once the data is cleaned, exploratory data analysis (EDA) provides valuable insights into its structure, patterns, and relationships. Common EDA techniques include:
- Descriptive Statistics: Calculating summary statistics like mean, median, mode, standard deviation, and percentiles gives a quick overview of the data's central tendency and variability.
- Data Visualization: Creating charts and graphs (histograms, scatter plots, box plots, etc.) allows for visual exploration of patterns, correlations, and outliers. This is often the most effective way to identify unexpected trends or anomalies.
- Correlation Analysis: Measuring the strength and direction of relationships between variables. This helps identify potential causal links (although correlation doesn't equal causation!).
3. Choosing the Right Analytical Techniques:
The type of data and the research question dictate the appropriate analytical techniques. Some common methods include:
- Regression Analysis: Used to model the relationship between a dependent variable and one or more independent variables. Linear regression is suitable for continuous dependent variables, while logistic regression is used for binary or categorical dependent variables.
- Hypothesis Testing: Used to test specific hypotheses about the population based on sample data. This often involves calculating p-values to assess the statistical significance of the results.
- ANOVA (Analysis of Variance): Used to compare means across multiple groups.
- Clustering Analysis: Used to group similar data points together, revealing underlying structures in the data.
- Time Series Analysis: Used to analyze data collected over time, identifying trends, seasonality, and other patterns.
Interpreting Results and Drawing Conclusions: Avoiding Common Pitfalls
Even with meticulous data analysis, drawing accurate conclusions requires careful interpretation and a critical eye. Here are some key considerations:
1. Statistical Significance vs. Practical Significance:
A statistically significant result (typically indicated by a low p-value) indicates that the observed effect is unlikely due to chance. However, statistical significance doesn't automatically imply practical significance. A small, statistically significant effect might be irrelevant in a real-world context. Always consider the magnitude of the effect and its real-world implications.
2. Correlation vs. Causation:
A strong correlation between two variables doesn't necessarily mean that one causes the other. There might be a third, confounding variable influencing both. Careful consideration of potential confounding factors is crucial to avoid drawing erroneous causal conclusions.
3. Sample Size and Generalizability:
The generalizability of your conclusions depends heavily on the sample size. Larger, more representative samples are more likely to yield reliable results that can be generalized to the broader population. Consider the limitations of your sample and avoid overgeneralizing your findings.
4. Limitations of the Data:
Acknowledge any limitations of your data, such as biases in sampling, measurement errors, or missing data. These limitations can affect the reliability and generalizability of your conclusions. Transparency about these limitations is essential for responsible data analysis.
5. Contextual Understanding:
Interpreting data always requires a deep understanding of the context. Consider the broader environment, relevant background information, and potential external factors that might influence the results. This allows for more nuanced and insightful conclusions.
6. Avoiding Confirmation Bias:
Confirmation bias is the tendency to favor information that confirms pre-existing beliefs. Be aware of this bias and actively seek out contradictory evidence to challenge your assumptions. Objectivity is key to drawing accurate conclusions.
The Best Conclusion: A Holistic Approach
The "best" conclusion isn't a single, definitive statement. It's a nuanced interpretation that considers all aspects of the data analysis process. It should:
- Be supported by the evidence: The conclusion should be directly supported by the findings of the analysis, avoiding speculation or unsupported claims.
- Acknowledge limitations: The conclusion should acknowledge any limitations of the data or the analysis, promoting transparency and responsible interpretation.
- Be clear and concise: The conclusion should be easily understood by the intended audience, avoiding jargon or overly technical language.
- Offer actionable insights: The best conclusion doesn't just summarize the findings; it also suggests actionable steps based on the insights gained. This might involve recommending further research, proposing policy changes, or suggesting improvements to a process.
- Consider the broader context: The conclusion should integrate the findings within the broader context, relating them to existing knowledge and theories in the field.
Case Study: Analyzing Marketing Campaign Data
Let's illustrate these principles with a hypothetical case study. Imagine a company analyzing the results of a marketing campaign across different social media platforms (Facebook, Instagram, Twitter). Their data includes metrics like impressions, clicks, conversions, and cost per conversion.
Data Cleaning and Preparation: The team first cleans the data, handling missing values and ensuring data consistency across platforms.
Exploratory Data Analysis: They visualize the data using charts and graphs, exploring the relationship between ad spend and conversions on each platform. They calculate key metrics like click-through rates (CTR) and conversion rates for each platform.
Analytical Techniques: They might use regression analysis to model the relationship between ad spend and conversions, controlling for factors like ad creative and targeting. They might also perform hypothesis testing to compare the effectiveness of different platforms.
Interpreting Results: Suppose the analysis reveals that Instagram yields the highest conversion rate but Facebook has a higher overall number of conversions due to significantly higher ad spend. The team must then consider the cost-effectiveness of each platform, balancing conversion rates with the cost per conversion.
The Best Conclusion: The best conclusion wouldn't simply state "Instagram is best." Instead, it might state something like: "While Instagram demonstrates a significantly higher conversion rate, Facebook's larger reach and higher ad spend resulted in a greater overall number of conversions. Optimizing ad spend on Facebook to improve its conversion rate, while maintaining a strong presence on Instagram, would likely maximize return on investment." This conclusion acknowledges the nuances of the data, avoids oversimplification, and provides actionable insights.
Conclusion: The Power of Critical Analysis
Drawing the "best" conclusion from data is a multifaceted process that requires careful planning, meticulous analysis, and critical interpretation. By following the principles outlined above, focusing on the holistic view, understanding statistical significance alongside practical relevance, and acknowledging the limitations of data and methodology, you can move beyond simple summaries to generate meaningful insights and inform better decisions. Remember, the goal is not just to analyze data but to use it effectively to drive action and achieve tangible results.
Latest Posts
Latest Posts
-
Which Of The Following Is Not Considered A Rebate
May 12, 2025
-
Which Of The Following Is Not An Example Of Privilege
May 12, 2025
-
Unit 4 Progress Check Mcq Ap Calc Bc
May 12, 2025
-
Employees Readiness For Training Includes Identifying Whether
May 12, 2025
-
Compute The Elastic Moduli For The Following Polymers
May 12, 2025
Related Post
Thank you for visiting our website which covers about What Is The Best Conclusion Based On This Data . We hope the information provided has been useful to you. Feel free to contact us if you have any questions or need further assistance. See you next time and don't miss to bookmark.