Why Are True Random Samples Rarely Used
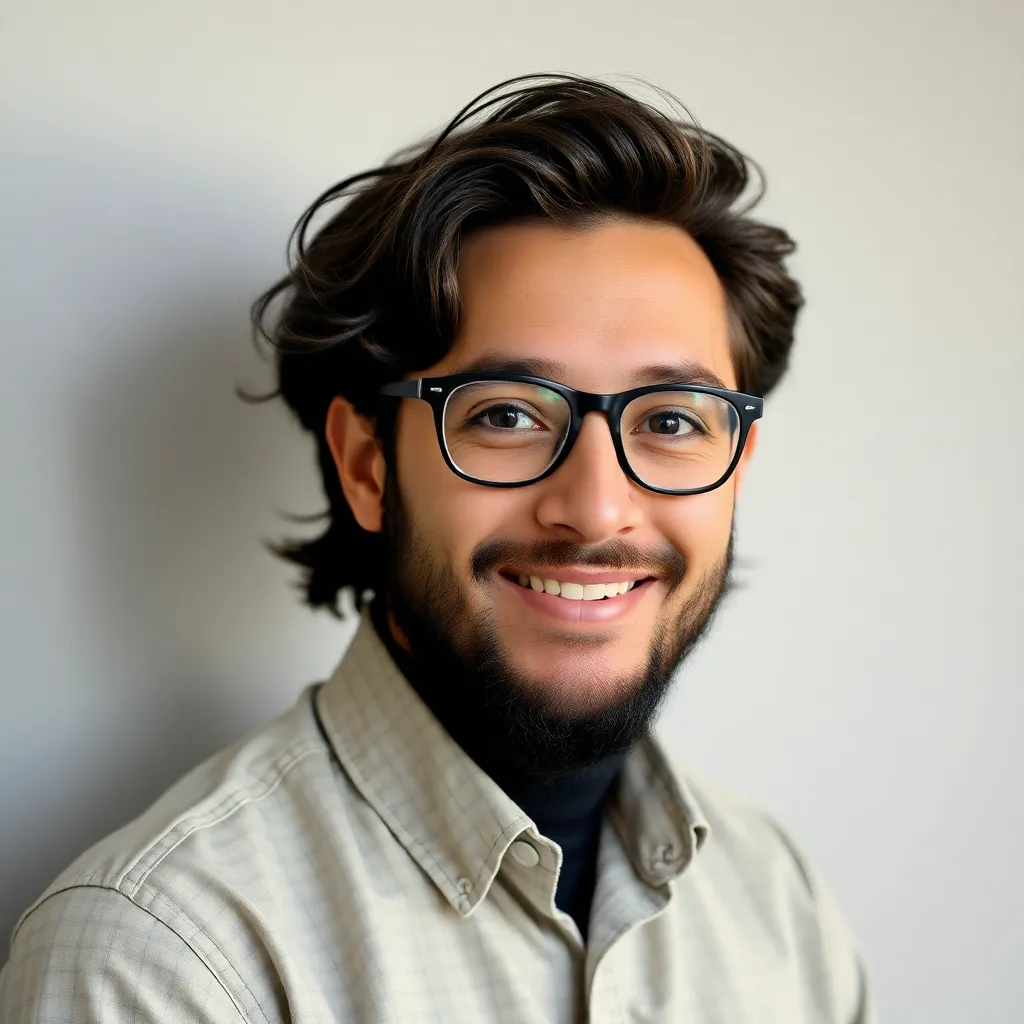
Onlines
May 09, 2025 · 6 min read
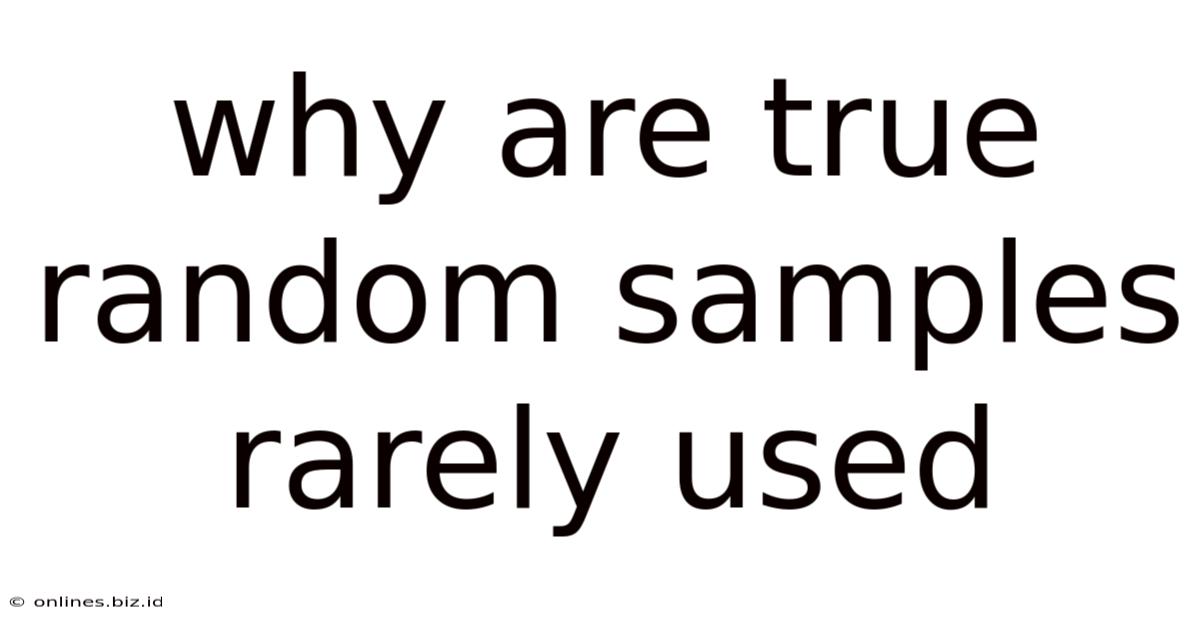
Table of Contents
Why Are True Random Samples Rarely Used? The Challenges of Achieving Perfect Randomness in Research
Obtaining a truly random sample is the holy grail of research design. It promises unbiased results, allowing researchers to generalize findings to the broader population with confidence. However, the reality is that perfectly random samples are exceptionally rare in practice. This article delves into the reasons behind this, exploring the inherent difficulties and practical limitations that prevent researchers from consistently achieving true randomness.
The Ideal: Defining a Truly Random Sample
Before understanding why true random samples are rarely used, let's clarify what constitutes a truly random sample. A truly random sample is one where every member of the population has an equal and independent chance of being selected. This implies:
- Equal Probability: Each individual in the population has the same likelihood of inclusion in the sample. No one is inherently more or less likely to be chosen than anyone else.
- Independence: The selection of one individual does not influence the probability of selecting another. The choices are entirely independent events.
This ideal scenario ensures that the sample accurately reflects the characteristics of the population, minimizing sampling bias and increasing the validity of the research findings.
The Reality: Why True Randomness Is Elusive
While the concept of a truly random sample is straightforward, achieving it in practice presents numerous formidable challenges:
1. Defining and Accessing the Entire Population
The very first hurdle is defining the population. What constitutes the entire group you wish to study? For instance, if you're researching the opinions of university students, defining the "population" requires identifying every single enrolled student across all departments, campuses, and programs. This can be surprisingly complex, especially with large and diverse populations. Even with seemingly straightforward populations, obtaining a complete and accurate list is often impractical.
Moreover, even if you have a complete list, accessing every member of the population can be incredibly difficult and costly. Imagine trying to contact every single citizen of a country for a survey—the logistical challenges and expense would be enormous.
2. Sampling Frame Limitations: The Incomplete List Problem
Often, researchers rely on a sampling frame, a readily available list that approximates the population. This could be a student registry, a voter registration list, or a customer database. However, sampling frames are rarely perfect representations of the entire population. They may:
- Exclude certain segments: Certain groups might be underrepresented or entirely missing from the sampling frame. For example, a phone survey would exclude individuals without landlines or cell phones.
- Contain outdated information: Lists can become outdated, containing inaccurate or incomplete information leading to missed individuals or duplicated entries.
- Be biased: The sampling frame itself might be inherently biased, reflecting systematic over- or under-representation of certain subgroups within the population.
These imperfections in the sampling frame directly compromise the randomness and representativeness of the sample.
3. Non-Response Bias: The Silent Majority Problem
Even with a perfect sampling frame and a meticulously designed random sampling method, researchers face the significant challenge of non-response bias. Not everyone selected for participation will actually respond. This non-response can be due to various factors:
- Inability to contact: Individuals might be unreachable due to travel, unlisted numbers, or other reasons.
- Refusal to participate: Some participants might decline to participate, perhaps due to time constraints, privacy concerns, or mistrust of the research.
- Incomplete responses: Even those who participate might not complete the entire survey or provide incomplete data.
Non-response disproportionately affects certain groups, leading to a sample that underrepresents these segments and distorts the overall findings. The silent majority, the non-respondents, remain a significant source of bias.
4. The Practicalities of Random Sampling Methods: Challenges in Implementation
Various random sampling techniques exist, each with its own complexities:
- Simple Random Sampling: While conceptually simple, assigning random numbers to every member of a large population and then selecting those numbers is often logistically challenging and time-consuming.
- Stratified Random Sampling: This method aims to improve representation by dividing the population into strata (subgroups) and then randomly sampling from each stratum. However, accurate stratification requires detailed knowledge of the population and can be challenging to achieve.
- Cluster Sampling: This involves dividing the population into clusters and then randomly selecting clusters to sample. While efficient for large populations, cluster sampling can introduce clustering effects, where individuals within the same cluster share similar characteristics, leading to potential bias.
Regardless of the method used, implementing random sampling perfectly often requires significant resources, technical expertise, and meticulous attention to detail. Errors in implementation can easily introduce bias, undermining the integrity of the random sample.
5. Ethical Considerations and Informed Consent: Respecting Participant Rights
Ethical considerations play a critical role in research. Obtaining true random samples often clashes with ethical principles. For instance, contacting individuals randomly might intrude on their privacy, particularly if the research involves sensitive topics.
The need for informed consent necessitates explaining the research's purpose and obtaining explicit permission from participants before collecting data. This requirement can significantly alter the sample's randomness, as some individuals might refuse to participate, introducing bias.
Approximations of Randomness: The Practical Approach
Given the inherent difficulties in achieving a truly random sample, researchers often rely on approximations of randomness. These methods aim to minimize bias as much as possible while acknowledging the practical limitations:
- Probability Sampling: These techniques, including stratified, cluster, and systematic sampling, aim to increase the likelihood of a representative sample, though they don't guarantee perfect randomness.
- Quota Sampling: This non-probability method aims for representativeness by ensuring specific subgroups are proportionately represented in the sample. While convenient, it lacks the randomness of probability methods.
- Convenience Sampling: This non-probability method uses readily available participants, often leading to significant bias. While frequently used due to its ease, results should be interpreted cautiously.
Researchers must carefully select and justify their sampling method, acknowledging its limitations and potential sources of bias in the analysis and interpretation of findings. Transparency about the sampling method is crucial for maintaining research integrity.
Conclusion: Striving for Representativeness, Not Perfect Randomness
While the ideal of a truly random sample remains a theoretical benchmark, the practical realities of research often necessitate compromises. Researchers should prioritize strategies that minimize bias and maximize the representativeness of their samples, acknowledging the inherent limitations of any sampling method. The focus should be on producing reliable and meaningful results, even if perfect randomness remains elusive. Transparency regarding the sampling method employed and potential biases is crucial for responsible and ethical research practice. Understanding the challenges of achieving true randomness allows for a more nuanced interpretation of research findings and fosters a more critical evaluation of the evidence base.
Latest Posts
Latest Posts
-
Answer The Following Question In 3 4 Complete Sentences
May 10, 2025
-
Anatomy Of The Heart Review Sheet Exercise 20
May 10, 2025
-
Calculus Early Transcendentals Eighth Edition Answers
May 10, 2025
-
Select The True Statement For Completely Random Design
May 10, 2025
-
The Second Statement Is The Of The First A B
May 10, 2025
Related Post
Thank you for visiting our website which covers about Why Are True Random Samples Rarely Used . We hope the information provided has been useful to you. Feel free to contact us if you have any questions or need further assistance. See you next time and don't miss to bookmark.