With A Probabilistic Model Increasing The Service Level
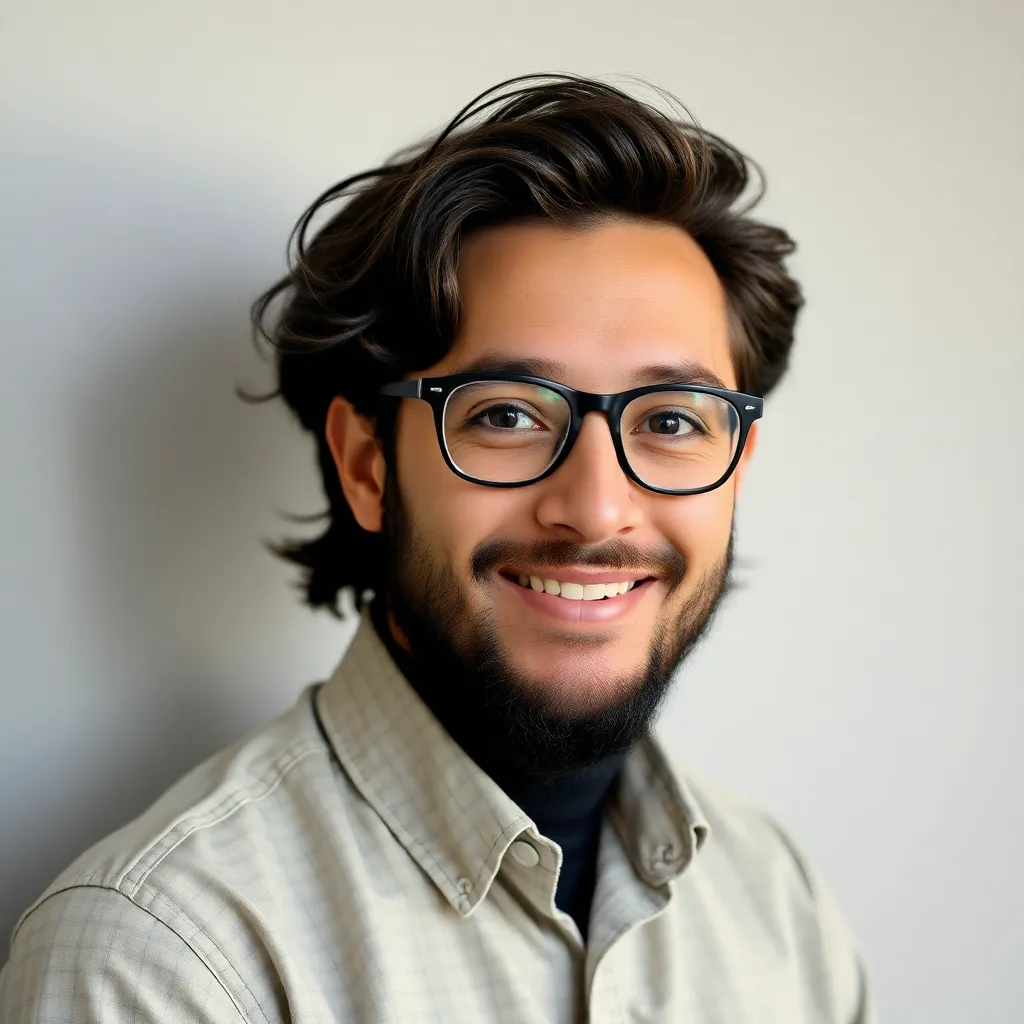
Onlines
May 10, 2025 · 6 min read
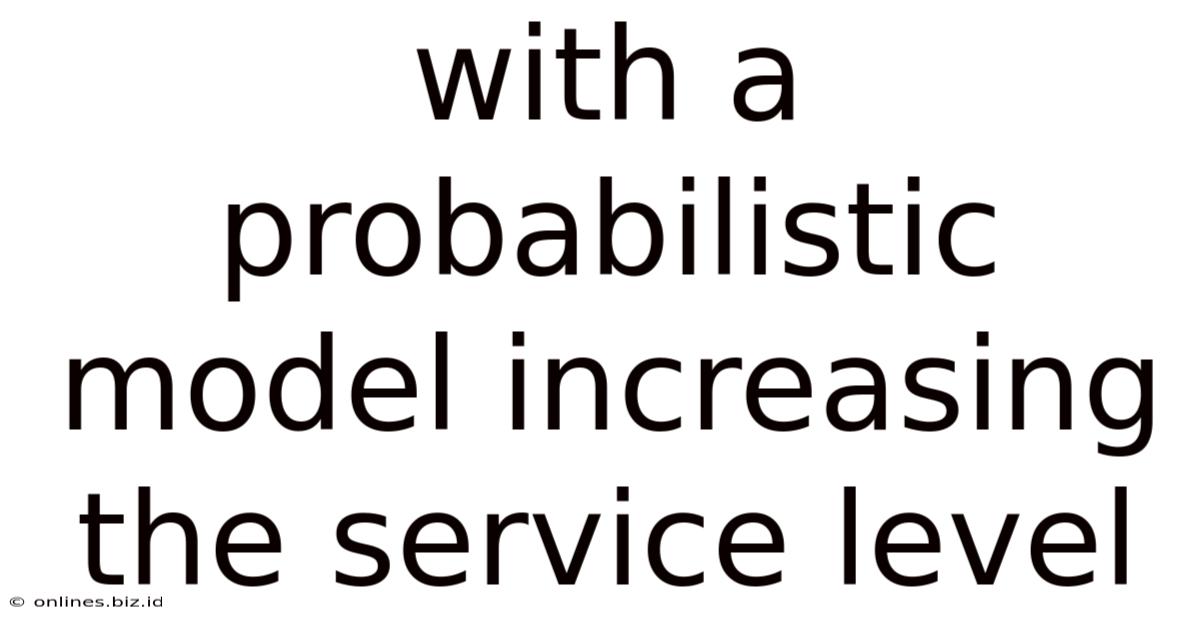
Table of Contents
Increasing Service Level with a Probabilistic Model
In today's competitive landscape, maintaining a high service level is paramount for any business. Customers expect quick response times, minimal downtime, and efficient service delivery. Meeting these expectations requires a proactive and data-driven approach, often leveraging sophisticated models to predict and optimize performance. This article delves into how a probabilistic model can significantly contribute to increasing service levels, exploring its benefits, implementation, and challenges.
Understanding Service Level and its Importance
Service level, often expressed as a percentage or a specific metric (e.g., mean time to resolution), quantifies the performance of a service against predefined expectations. A high service level indicates that the service consistently meets or exceeds customer requirements, leading to increased customer satisfaction, loyalty, and ultimately, revenue. Conversely, a low service level can result in customer churn, damage to reputation, and significant financial losses.
Key aspects of service level:
- Availability: The percentage of time a service is operational and accessible to users.
- Responsiveness: The speed at which a service responds to requests.
- Reliability: The consistency with which a service performs its intended function without failures.
- Recoverability: The speed and efficiency with which a service recovers from failures.
Achieving a high service level requires a comprehensive strategy, encompassing proactive monitoring, preventative maintenance, and efficient incident management. This is where a probabilistic model comes into play.
Introducing Probabilistic Models for Service Level Improvement
Probabilistic models are statistical tools that leverage probability theory to predict future outcomes based on historical data and assumptions about underlying processes. In the context of service level improvement, these models are invaluable for:
- Predictive Maintenance: By analyzing historical data on equipment failures, a probabilistic model can predict the likelihood of future failures and schedule preventative maintenance accordingly. This reduces downtime and improves overall availability.
- Resource Allocation: By forecasting demand based on historical patterns and seasonal variations, a probabilistic model can optimize resource allocation, ensuring sufficient resources are available to handle peak loads and minimize delays.
- Capacity Planning: Understanding the probabilistic distribution of service requests allows for more accurate capacity planning, preventing bottlenecks and ensuring that the service can handle expected workloads.
- Risk Assessment: By assessing the probability of different failure scenarios, a probabilistic model can help prioritize mitigation strategies, focusing resources on the most likely and impactful risks.
- Performance Monitoring and Alerting: Probabilistic models can analyze real-time data streams, identifying deviations from expected behavior and triggering alerts before service degradation occurs.
Types of Probabilistic Models:
Several probabilistic models are suitable for improving service level. The choice depends on the specific context and available data. Some commonly used models include:
- Markov Chains: Useful for modeling systems with discrete states and transitions between these states. This is suitable for analyzing the behavior of complex systems, such as network infrastructure.
- Bayesian Networks: Represent probabilistic relationships between variables. These are helpful for understanding the influence of different factors on service performance and identifying potential bottlenecks.
- Hidden Markov Models (HMMs): Suitable for modeling systems where the underlying state is not directly observable. This can be useful for analyzing situations where the cause of service degradation is not immediately apparent.
- Time Series Analysis: Techniques like ARIMA (Autoregressive Integrated Moving Average) can be used to forecast future service demands based on historical patterns.
Implementing a Probabilistic Model for Service Level Improvement
Implementing a probabilistic model requires a systematic approach:
1. Data Collection and Preparation:
- Identify Key Metrics: Determine the critical metrics that impact service level (e.g., response time, downtime, error rate).
- Gather Historical Data: Collect historical data on these metrics, ensuring data quality and completeness.
- Data Cleaning and Preprocessing: Clean and preprocess the data, handling missing values and outliers.
- Feature Engineering: Create new features from existing data to improve model accuracy (e.g., combining multiple metrics into a composite score).
2. Model Selection and Training:
- Choose an Appropriate Model: Select a probabilistic model suitable for the specific problem and available data.
- Train the Model: Train the chosen model using the prepared data, optimizing its parameters to minimize prediction error.
- Model Evaluation: Evaluate the model's performance using appropriate metrics (e.g., accuracy, precision, recall). Consider techniques like cross-validation to ensure robustness.
3. Model Deployment and Monitoring:
- Integrate the Model: Integrate the trained model into the service monitoring and management system.
- Real-time Predictions: Use the model to generate real-time predictions of service performance.
- Continuous Monitoring: Continuously monitor the model's performance and retrain it periodically with new data to maintain accuracy.
4. Actionable Insights and Decision Making:
- Alerting System: Implement an alerting system to notify relevant personnel of potential service degradation based on the model's predictions.
- Proactive Measures: Take proactive measures based on the model's predictions, such as scheduling preventative maintenance or allocating additional resources.
- Continuous Improvement: Continuously analyze the model's output and refine the service management strategy based on the insights gained.
Challenges and Considerations
While probabilistic models offer significant benefits, there are challenges to consider:
- Data Availability and Quality: Accurate and sufficient historical data is crucial for effective model training. Lack of data or poor data quality can limit the model's accuracy.
- Model Complexity: Some probabilistic models can be complex and require specialized expertise to implement and interpret.
- Computational Resources: Training and deploying complex models may require significant computational resources.
- Model Bias: Models can inherit biases from the training data, leading to inaccurate or unfair predictions.
- Unforeseen Events: Probabilistic models may not accurately predict unforeseen events or "black swan" scenarios.
Case Studies: Real-World Applications
Numerous organizations have successfully implemented probabilistic models to improve service levels. Examples include:
- Telecommunications companies using probabilistic models to predict network outages and optimize resource allocation.
- E-commerce platforms leveraging probabilistic models to forecast demand and ensure sufficient capacity during peak shopping seasons.
- Healthcare providers using probabilistic models to predict patient demand and optimize staffing levels.
- Manufacturing companies employing probabilistic models for predictive maintenance of equipment, reducing downtime and improving production efficiency.
These case studies highlight the significant impact that probabilistic models can have on improving service levels across various industries.
Conclusion: Embracing Probabilistic Modeling for Superior Service
In conclusion, implementing a probabilistic model offers a powerful approach to increasing service level. By leveraging historical data and statistical techniques, these models enable proactive monitoring, preventative maintenance, and optimized resource allocation. While challenges exist regarding data availability, model complexity, and unforeseen events, the benefits significantly outweigh the risks. By adopting a systematic approach to data collection, model selection, deployment, and continuous monitoring, organizations can leverage the power of probabilistic modeling to achieve superior service levels, exceeding customer expectations, and gaining a competitive advantage. The future of service level management increasingly relies on the insightful capabilities of these sophisticated analytical tools. Embracing this technology is no longer a luxury; it's a necessity for success in the modern digital world.
Latest Posts
Latest Posts
-
Nature Of Science Amoeba Sisters Answer Key
May 10, 2025
-
Quantitative Methods For Business 13th Edition
May 10, 2025
-
An Experienced User Has Installed Oracle Vm
May 10, 2025
-
10 6 Study Guide And Intervention
May 10, 2025
-
In Marketing States Of Felt Deprivation Are Referred To As
May 10, 2025
Related Post
Thank you for visiting our website which covers about With A Probabilistic Model Increasing The Service Level . We hope the information provided has been useful to you. Feel free to contact us if you have any questions or need further assistance. See you next time and don't miss to bookmark.